20 Using Automated Sentiment Analysis to Examine Self-Evaluation in Youth with Autism Spectrum Disorder
Journal of the International Neuropsychological Society(2023)
摘要
Objective:Individuals diagnosed with autism spectrum disorder (ASD) experience negative self-evaluation, indicated by low levels of self-esteem and describing themselves more negatively to others. Variations in reading comprehension, difficulty identifying emotions, and masking (camouflaging of autistic traits) make it difficult to accurately measure self-evaluation of individuals with ASD using subjective self-report scales such as the Rosenburg Self-Esteem Scale. Therefore, it is important to explore more objective methods of measuring self-evaluation in ASD. Sentiment analysis is a popular Natural Language Processing (NLP) technique used to quantify the emotional content of language programmatically by automatically transforming text into a data frame of words represented as individual values or tokens. Each token can then be categorized as positive or negative with a sentiment dictionary. The current study aims to investigate an automated sentiment analysis approach to evaluate self-evaluation by quantifying implicit linguistic affective valence of ASD participants' verbal self-describing statements in a naturalistic setting. Specifically, we evaluated the frequency of positive or negative words used during a mock job interview in which individuals with ASD were asked to describe themselves. We then examined the relationship between positive and negative word usage and standard self-report measures of self-evaluation.Participants and Methods:Twenty-four young adults with ASD were included in this study with an age range of 15-24 and a mean age of 19.2 years. Participants completed a battery of assessments including a mock job interview in which they were asked to describe themselves as a measure of implicit self-esteem. Self-esteem and knowledge of personal strengths was assessed using the Rosenberg Self-Esteem Scale and Strengths Knowledge Scale, respectively. Interview transcripts were automatically transformed into word token data frames using the tidytext package in Rstudio. Frequencies of positive and negative words were calculated and their ratio to total word count was used to measure the implicit positivity and negativity of transcripts.Results:There was a significant negative correlation between the frequency of negative sentiment in transcripts and measure on the Rosenburg Self-Esteem Scale (r = -.376, p = .035) and the Strengths Knowledge Scale (r = -.387, p = .031) indicating that individuals with higher self-esteem and knowledge of their strengths used fewer negative words when talking during a mock interview.Conclusions:While our results are preliminary, this pilot study represents the first to use automated sentiment analysis to study self-evaluation in individuals with ASD. The use of this technique on natural linguistic data collected through a mock job interview allows researchers to quantitatively analyze the emotionality of transcriptions and create insights that would otherwise be unavailable using more subjective qualitative techniques. Limited research into self-evaluation in this population has yielded inconsistent results, relying too heavily upon qualitative or self-report measures. The ability to programmatically quantify affective valence in transcripts is a time and cost-effective technique for improving validity of future measures of self-evaluation.
更多查看译文
AI 理解论文
溯源树
样例
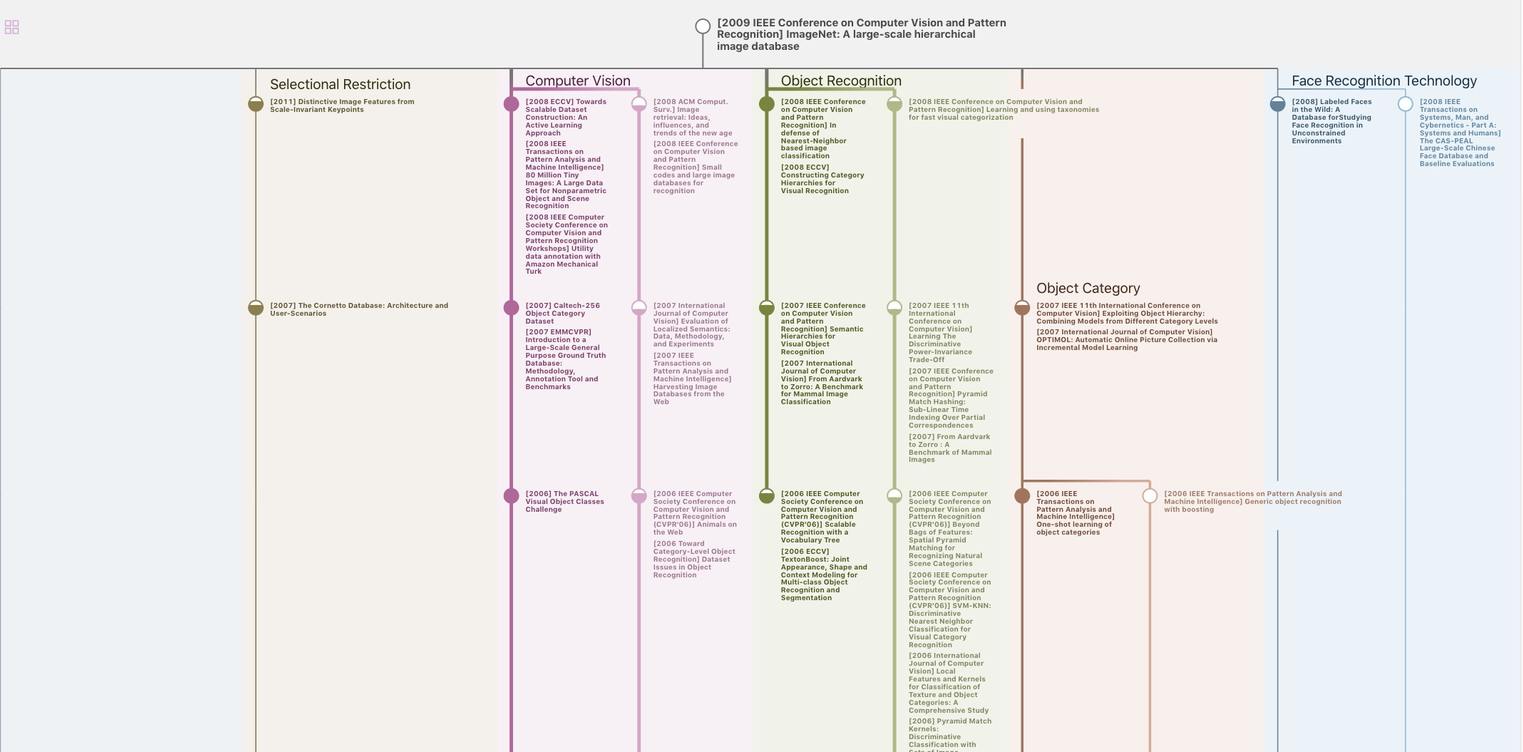
生成溯源树,研究论文发展脉络
Chat Paper
正在生成论文摘要