Multi-Modal Domain Adaptation Across Video Scenes for Temporal Video Grounding
CoRR(2023)
摘要
Temporal Video Grounding (TVG) aims to localize the temporal boundary of a
specific segment in an untrimmed video based on a given language query. Since
datasets in this domain are often gathered from limited video scenes, models
tend to overfit to scene-specific factors, which leads to suboptimal
performance when encountering new scenes in real-world applications. In a new
scene, the fine-grained annotations are often insufficient due to the expensive
labor cost, while the coarse-grained video-query pairs are easier to obtain.
Thus, to address this issue and enhance model performance on new scenes, we
explore the TVG task in an unsupervised domain adaptation (UDA) setting across
scenes for the first time, where the video-query pairs in the source scene
(domain) are labeled with temporal boundaries, while those in the target scene
are not. Under the UDA setting, we introduce a novel Adversarial Multi-modal
Domain Adaptation (AMDA) method to adaptively adjust the model's scene-related
knowledge by incorporating insights from the target data. Specifically, we
tackle the domain gap by utilizing domain discriminators, which help identify
valuable scene-related features effective across both domains. Concurrently, we
mitigate the semantic gap between different modalities by aligning video-query
pairs with related semantics. Furthermore, we employ a mask-reconstruction
approach to enhance the understanding of temporal semantics within a scene.
Extensive experiments on Charades-STA, ActivityNet Captions, and YouCook2
demonstrate the effectiveness of our proposed method.
更多查看译文
AI 理解论文
溯源树
样例
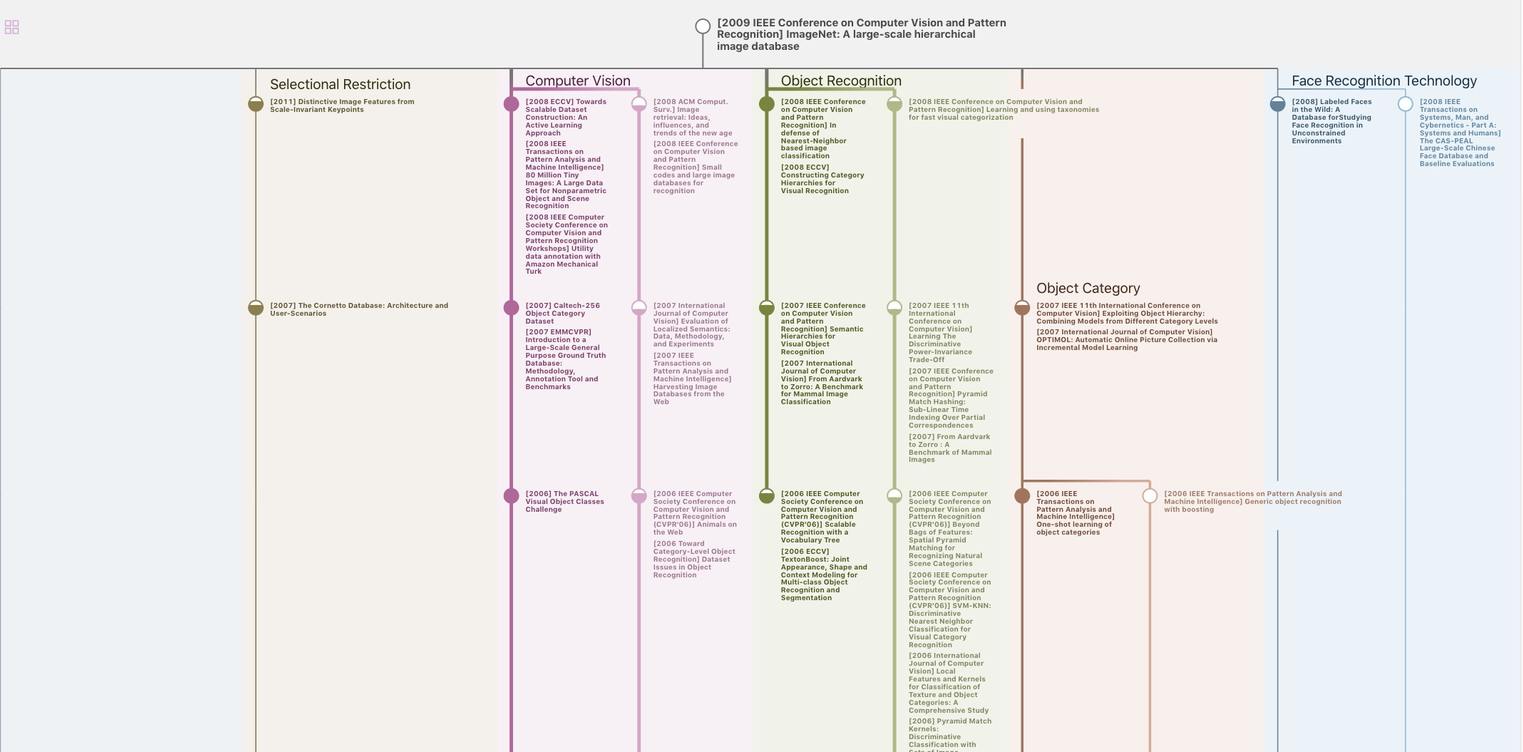
生成溯源树,研究论文发展脉络
Chat Paper
正在生成论文摘要