Controllable 3D Face Generation with Conditional Style Code Diffusion
AAAI 2024(2024)
摘要
Generating photorealistic 3D faces from given conditions is a challenging task. Existing methods often rely on time-consuming one-by-one optimization approaches, which are not efficient for modeling the same distribution content, e.g., faces. Additionally, an ideal controllable 3D face generation model should consider both facial attributes and expressions.
Thus we propose a novel approach called TEx-Face(TExt & Expression-to-Face) that addresses these challenges by dividing the task into three components, i.e., 3D GAN Inversion, Conditional Style Code Diffusion, and 3D Face Decoding. For 3D GAN inversion, we introduce two methods, which aim to enhance the representation of style codes and alleviate 3D inconsistencies. Furthermore, we design a style code denoiser to incorporate multiple conditions into the style code and propose a data augmentation strategy to address the issue of insufficient paired visual-language data. Extensive experiments conducted on FFHQ, CelebA-HQ, and CelebA-Dialog demonstrate the promising performance of our TEx-Face in achieving the efficient and controllable generation of photorealistic 3D faces. The code will be publicly available.
更多查看译文
关键词
CV: 3D Computer Vision,CV: Biometrics, Face, Gesture & Pose,CV: Computational Photography, Image & Video Synthesis,CV: Language and Vision,CV: Multi-modal Vision
AI 理解论文
溯源树
样例
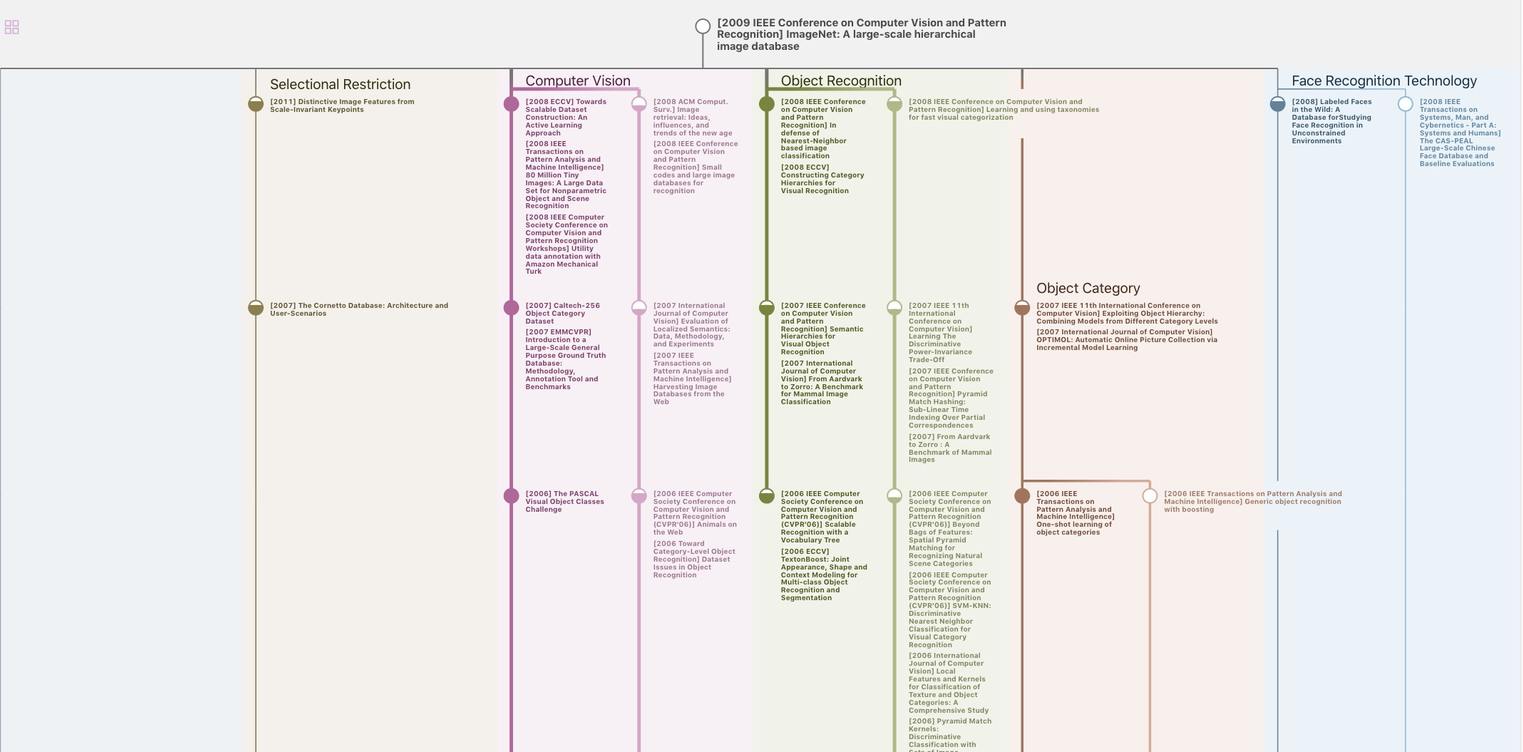
生成溯源树,研究论文发展脉络
Chat Paper
正在生成论文摘要