TA-denseNet: Efficient hardware trust and assurance model based on feature extraction and comparison of SEM images and GDSII images
INTEGRATION-THE VLSI JOURNAL(2024)
摘要
Hardware trust and assurance, which relies on extracting and analyzing information from GDSII images and SEM images of integrated circuits, plays a critical role in ensuring the integrity, privacy, security, and functionality of integrated circuits. However, with the continuous improvement of integrated circuits integration, traditional approaches for hardware trust and assurance face great challenges owing to the low efficiency, low precision, and high cost. To solve the issue mentioned above, we propose a novel, automatic, and efficient deep learning-based method, called TA-denseNet, which directly learns the mapping from GDSII images and SEM images to the compact Euclidean space, where the distances between GDSII images and SEM images directly correspond to measures of similarity. Also, a new hardware trust and assurance dataset for model training and evaluation, named TA-dataset, is proposed. Finally, we evaluate the accuracy of the proposed TA-denseNet model in simi-larity measures between GDSII images and SEM images on the test sets of the proposed dataset and obtain an accuracy of over 99.83%, which shows that the TA-denseNet model proposed in this paper has good performance on direct feature extraction and comparison between GDSII images and SEM images.
更多查看译文
关键词
Scanning electron microscopy,Deep learning,Hardware trust and assurance,Integrated circuit
AI 理解论文
溯源树
样例
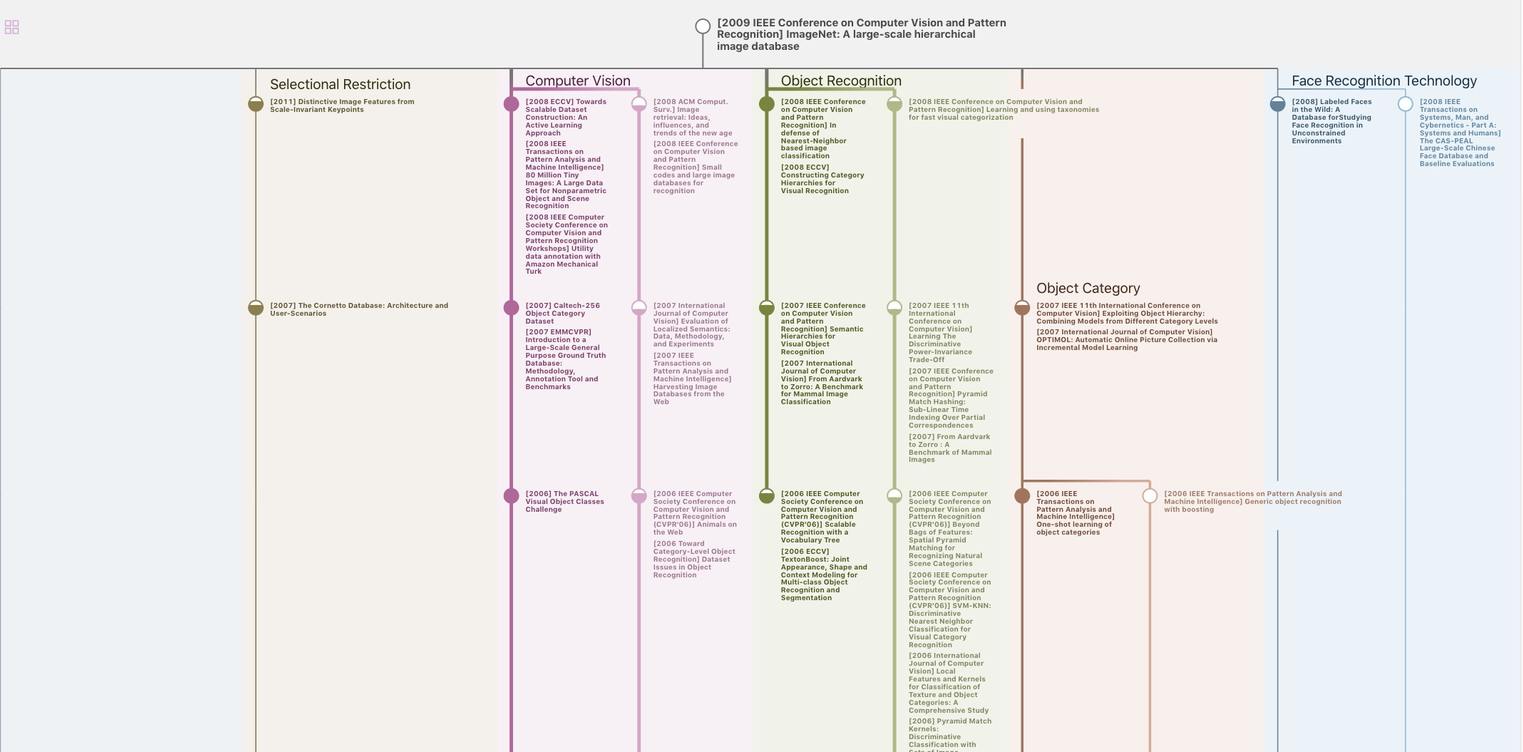
生成溯源树,研究论文发展脉络
Chat Paper
正在生成论文摘要