Computer vision-based six layered ConvNeural network to recognize sign language for both numeral and alphabet signs
Biomimetic Intelligence and Robotics(2024)
摘要
People who have trouble communicating verbally are often dependent on sign language, which can be difficult for most people to understand, making interaction with them a difficult endeavor. The Sign Language Recognition (SLR) system takes an input expression from a hearing or speaking-impaired person and outputs it in the form of text or voice to a normal person. The existing study related to the Sign Language Recognition system has some drawbacks, such as a lack of large datasets and datasets with a range of backgrounds, skin tones, and ages. This research efficiently focuses on Sign Language Recognition to overcome previous limitations. Most importantly, we use our proposed Convolutional Neural Network (CNN) model, “ConvNeural”, in order to train our dataset. Additionally, we develop our own datasets, “BdSL_OPSA22_STATIC1” and “BdSL_OPSA22_STATIC2”, both of which have ambiguous backgrounds. “BdSL_OPSA22_STATIC1” and “BdSL_OPSA22_STATIC2” both include images of Bangla characters and numerals, a total of 24,615 and 8437 images, respectively. The “ConvNeural” model outperforms the pre-trained models with accuracy of 98.38% for “BdSL_OPSA22_STATIC1” and 92.78% for “BdSL_OPSA22_STATIC2”. For “BdSL_OPSA22_STATIC1” dataset, we get precision, recall, F1-score, sensitivity and specificity of 96%, 95%, 95%, 99.31% , and 95.78% respectively. Moreover, in case of “BdSL_OPSA22_STATIC2” dataset, we achieve precision, recall, F1-score, sensitivity and specificity of 90%, 88%, 88%, 100%, and 100% respectively.
更多查看译文
关键词
ConvNeural,Sign language,CNN,Static,Feature extraction,Convolution2D,Fully connected layer,Dropout
AI 理解论文
溯源树
样例
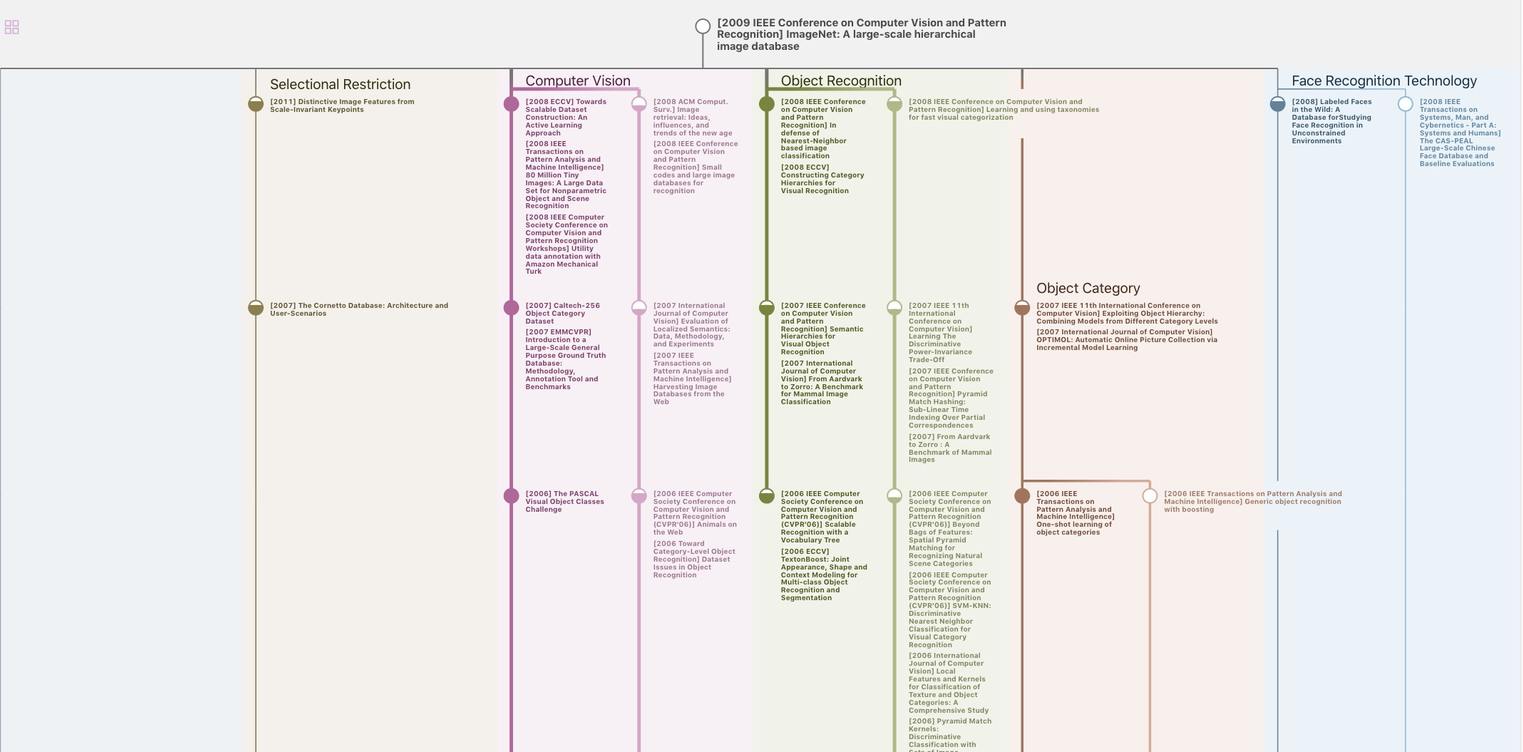
生成溯源树,研究论文发展脉络
Chat Paper
正在生成论文摘要