Machine learning enabled prediction of tribological properties of Cu-TiC-GNP nanocomposites synthesized by electric resistance sintering: A comparison with RSM
JOURNAL OF MATERIALS RESEARCH AND TECHNOLOGY-JMR&T(2024)
摘要
In the present study, copper matrix composites were successfully produced through the powder metallurgy route by applying the electrical resistance sintering technique. Copper composites were reinforced with 5 wt. % TiC and different concentrations of GNP (0.1, 0.2, and 0.3 wt. %). Microstructural investigations confirmed uniform dispersion of TiC and GNP micro and nanoparticles in the copper matrix. A sintering temperature of 900 degrees C resulted in better densification and hardness of the prepared composites. Moreover, ML models were developed to predict the sintered density, hardness, and wear loss of the composites. Further, it was found that Multi-Layer Perceptron outperforms all other ML models with R2 values of 0.975, 0.934, and 0.948 in the prediction of density, hardness, and wear loss of the composites. On the other hand, RSM shows predicted R2 values of 0.8012, 0.8507, and 0.8756.
更多查看译文
关键词
Copper metal matrix composite,Graphene,Electric resistance sintering,Response surface methodology,Machine learning,Hybrid nanocomposite
AI 理解论文
溯源树
样例
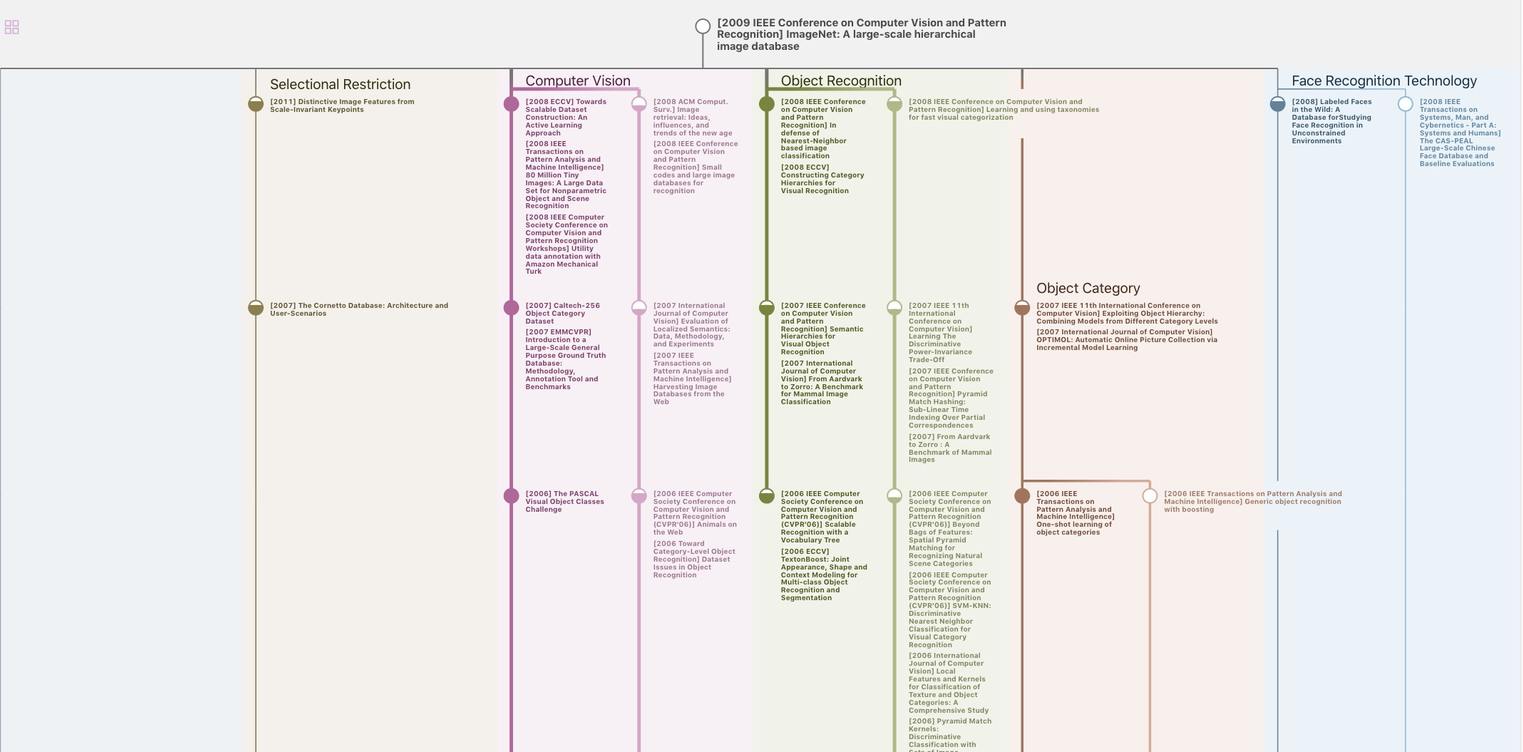
生成溯源树,研究论文发展脉络
Chat Paper
正在生成论文摘要