Efficient and accurate TEC modeling and prediction approach with random forest and Bi-LSTM for large-scale region
ADVANCES IN SPACE RESEARCH(2024)
摘要
The total electron content (TEC) is a crucial parameter for ionosphere monitoring, and the development of accurate TEC estimation and prediction models is significant for high-precision positioning. However, establishing a high-precision ionospheric model that can effectively improve prediction accuracy remains a challenging task. We propose a method that combines random forest with a BiLSTM neural network to establish a high-precision ionospheric prediction model. The random forest algorithm is used for regression analysis and to estimate the variable importance of input parameters. We use observation data from the Crustal Movement Observation Network of China and International GNSS Services while estimating the relative importance of 14 input variables at different latitudes. The results demonstrate that our proposed model achieves more efficient and accurate predictions, with a correlation coefficient of 0.96, indicating significant improvement in accuracy compared to traditional RNN and LSTM models. Overall, the Bi-LSTM forecast model based on random forest can effectively capture the temporal and spatial variation characteristics of ionospheric TEC in China. This enables the model to provide accurate ionospheric information for positioning users, thereby improving the precision of positioning applications. (c) 2023 COSPAR. Published by Elsevier B.V. All rights reserved.
更多查看译文
关键词
Ionospheric modeling,Ionospheric prediction,Deep learning,Random forest
AI 理解论文
溯源树
样例
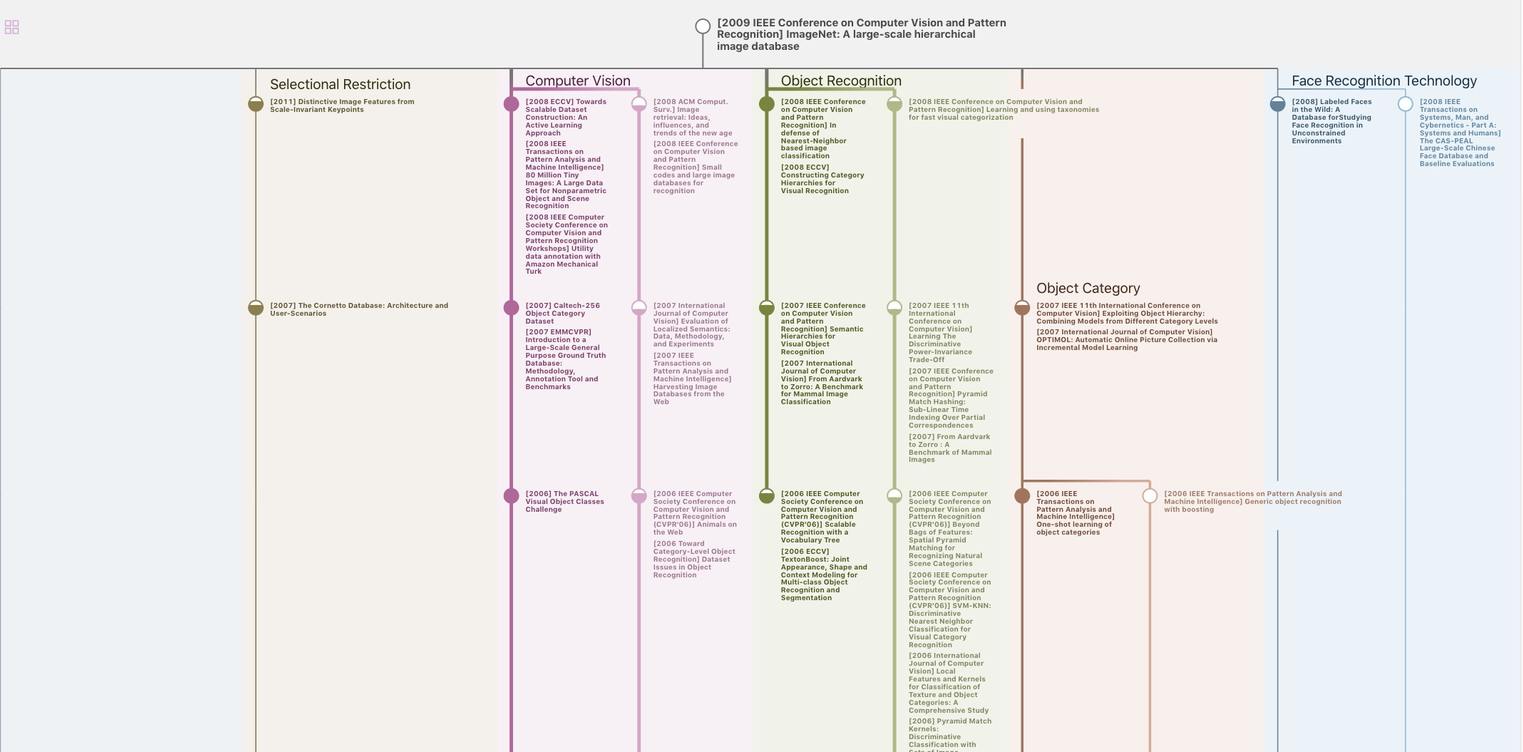
生成溯源树,研究论文发展脉络
Chat Paper
正在生成论文摘要