IS-DARTS: Stabilizing DARTS through Precise Measurement on Candidate Importance
AAAI 2024(2024)
摘要
Among existing Neural Architecture Search methods, DARTS is known for its efficiency and simplicity. This approach applies continuous relaxation of network representation to construct a weight-sharing supernet and enables the identification of excellent subnets in just a few GPU days. However, performance collapse in DARTS results in deteriorating architectures filled with parameter-free operations and remains a great challenge to the robustness. To resolve this problem, we reveal that the fundamental reason is the biased estimation of the candidate importance in the search space through theoretical and experimental analysis, and more precisely select operations via information-based measurements. Furthermore, we demonstrate that the excessive concern over the supernet and inefficient utilization of data in bi-level optimization also account for suboptimal results. We adopt a more realistic objective focusing on the performance of subnets and simplify it with the help of the informationbased measurements. Finally, we explain theoretically why progressively shrinking the width of the supernet is necessary and reduce the approximation error of optimal weights in DARTS. Our proposed method, named IS-DARTS, comprehensively improves DARTS and resolves the aforementioned problems. Extensive experiments on NAS-Bench-201 and DARTS-based search space demonstrate the effectiveness of IS-DARTS.
更多查看译文
关键词
ML: Auto ML and Hyperparameter Tuning,CV: Learning & Optimization for CV,ML: Deep Neural Architectures and Foundation Models,ML: Optimization
AI 理解论文
溯源树
样例
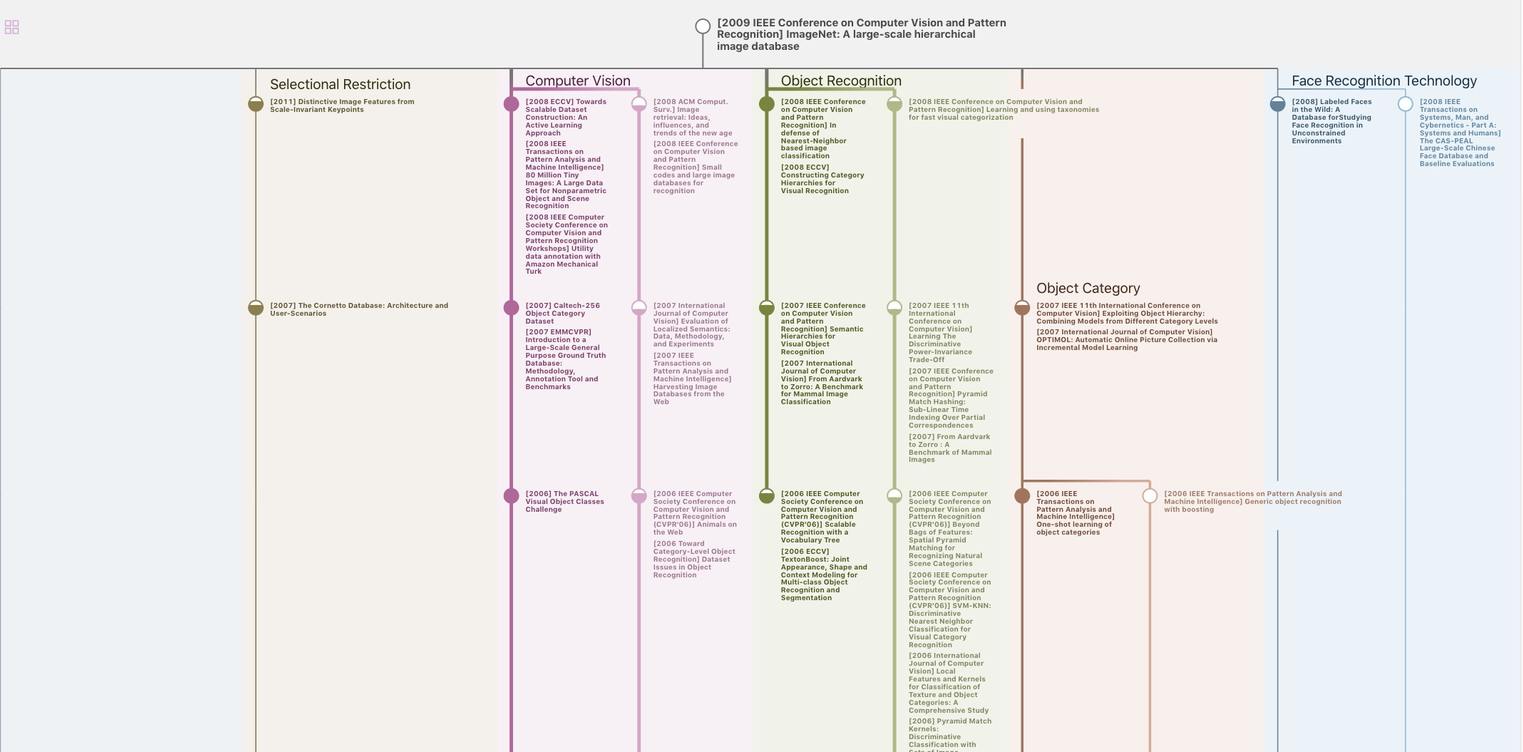
生成溯源树,研究论文发展脉络
Chat Paper
正在生成论文摘要