Airborne Integrated Access and Backhaul Systems: Learning-Aided Modeling and Optimization
IEEE TRANSACTIONS ON VEHICULAR TECHNOLOGY(2023)
摘要
The deployment of millimeter-wave (mmWave) 5G New Radio (NR) networks is hampered by the properties of the mmWave band, such as severe signal attenuation and dynamic link blockage, which together limit the cell range. To provide a cost-efficient and flexible solution for network densification, 3GPP has recently proposed integrated access and backhaul (IAB) technology. As an alternative approach to terrestrial deployments, the utilization of unmanned aerial vehicles (UAVs) as IAB-nodes may provide additional flexibility for topology configuration. The aims of this study are to (i) propose efficient optimization methods for airborne and conventional IAB systems and (ii) numerically quantify and compare their optimized performance. First, by assuming fixed locations of IAB-nodes, we formulate and solve the joint path selection and resource allocation problem as a network flow problem. Then, to better benefit from the utilization of UAVs, we relax this constraint for the airborne IAB system. To efficiently optimize the performance for this case, we propose to leverage deep reinforcement learning (DRL) method for specifying airborne IAB-node locations. Our numerical results show that the capacity gains of airborne IAB systems are notable even in non-optimized conditions but can be improved by up to 30 % under joint path selection and resource allocation and, even further, when considering aerial IAB-node locations as an additional optimization criterion.
更多查看译文
关键词
5G NR,mmWave,integrated access and backhaul,deep reinforcement learning,actor-critic
AI 理解论文
溯源树
样例
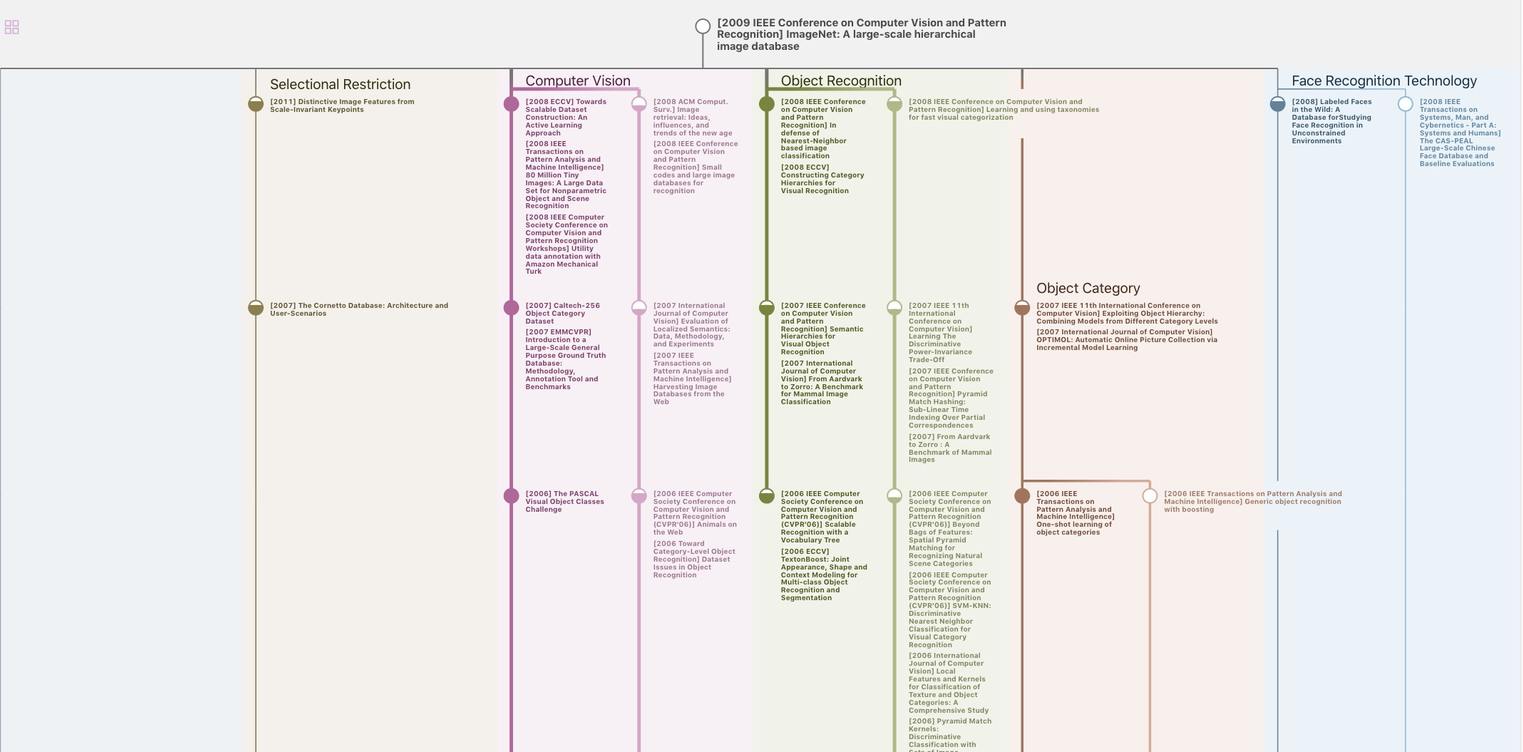
生成溯源树,研究论文发展脉络
Chat Paper
正在生成论文摘要