Multiorder Graph Convolutional Network With Channel Attention for Hyperspectral Change Detection
IEEE JOURNAL OF SELECTED TOPICS IN APPLIED EARTH OBSERVATIONS AND REMOTE SENSING(2024)
摘要
Hyperspectral change detection (CD) aims to obtain the change information of objects in the multitemporal hyperspectral images (HSIs). Recently, with the advantages in fully extracting the image features of irregular areas, the graph convolutional network (GCN) has attracted increasing attention for hyperspectral CD. The existing GCN-based CD methods usually use a graph structure constructed by superpixels to reduce the computational cost, which ignores the multiorder difference information among graph nodes and the local difference information within superpixels. To address these problems, this article proposes an efficient multiorder GCN with a channel attention module (CAM) for hyperspectral CD. Specifically, the multiorder GCN module is designed by repeatedly mixing the feature representations of neighborhoods. The CAM is then proposed to enhance the difference features of bitemporal HSIs. After that, the pixel-wise CD is accomplished by a lightweight feature fusion module and a fully connected layer. Experiments on three hyperspectral datasets illustrated the effectiveness of the proposed algorithm.
更多查看译文
关键词
Change detection (CD),graph convolutional network (GCN),hyperspectral images (HSI)
AI 理解论文
溯源树
样例
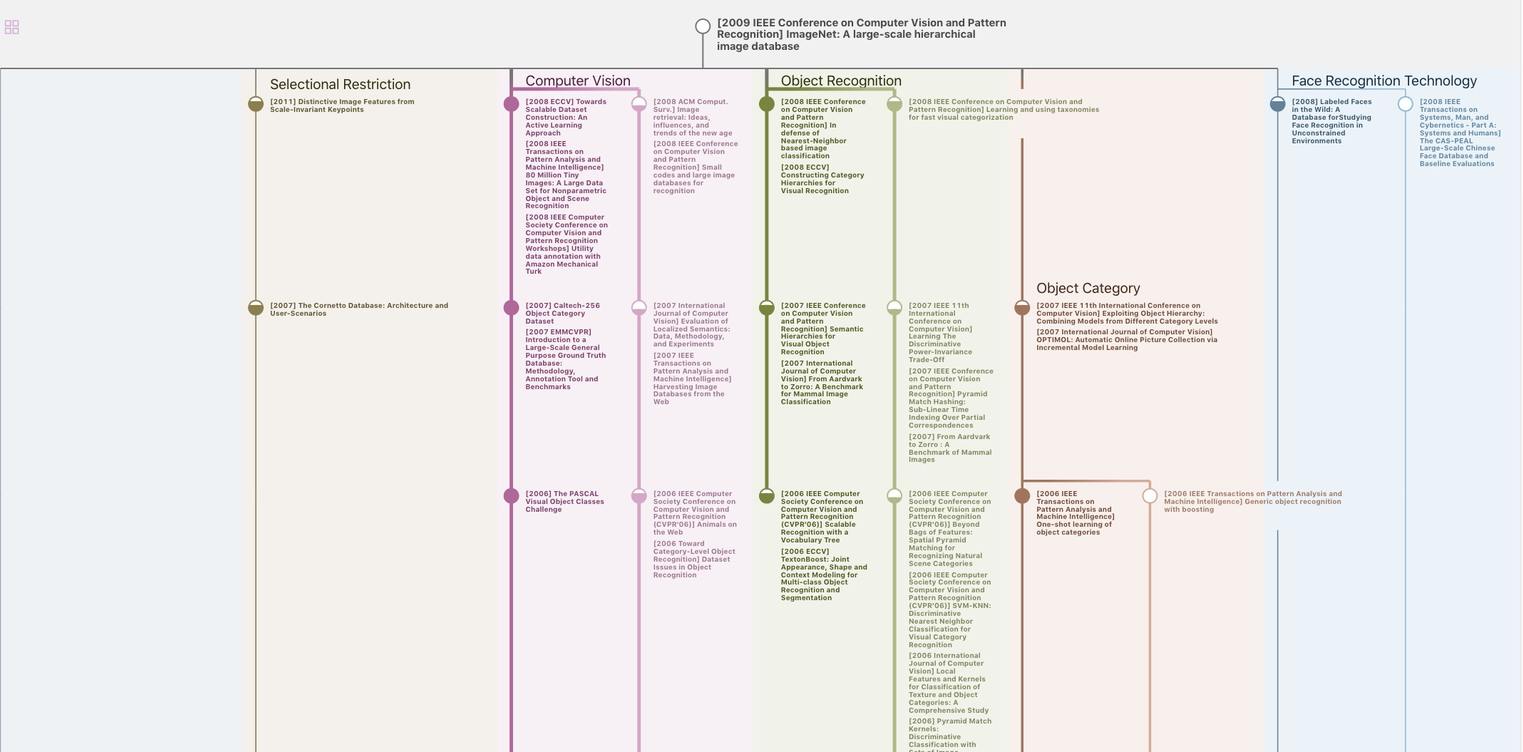
生成溯源树,研究论文发展脉络
Chat Paper
正在生成论文摘要