FS-DCL: Distributed Collaborative Learning for Few-Shot Remote Sensing Image Classification
IEEE GEOSCIENCE AND REMOTE SENSING LETTERS(2024)
摘要
With the development of on-orbit hardware and distributed multiplatform observation systems in satellite remote sensing (RS) scenario, on-orbit collaborative model updating has become a promising trend. Due to restrictions of imaging conditions and storage resources, on-orbit updating is usually carried out with limited samples. However, existing collaborative learning methods rarely consider the few-shot problem. To address this issue, this letter innovatively proposes a distributed collaborative learning method for few-shot RS image classification (FS-DCL), which encourages the collaboration between satellites with similar data distribution to supplement useful information for each satellite, and design on-orbit models to extract more discriminative features. Specifically, a personalized parameter aggregation strategy (PPAS) is proposed to generate personalized parameters for each satellite based on information from satellites with similar data distributions, providing information gain to alleviate problems of insufficient samples. Besides, a feature enhancement method (FEM) is applied to on-orbit models to enhance the feature representation and produce a more discriminative feature space, thus improving the accuracy of few-shot metric classification. Extensive experiments on two RS datasets demonstrate the superiority of FS-DCL.
更多查看译文
关键词
Satellites,Training,Data models,Computational modeling,Image classification,Finite element analysis,Federated learning,Collaborative learning,few-shot learning,heterogeneous data,on-orbit model updating
AI 理解论文
溯源树
样例
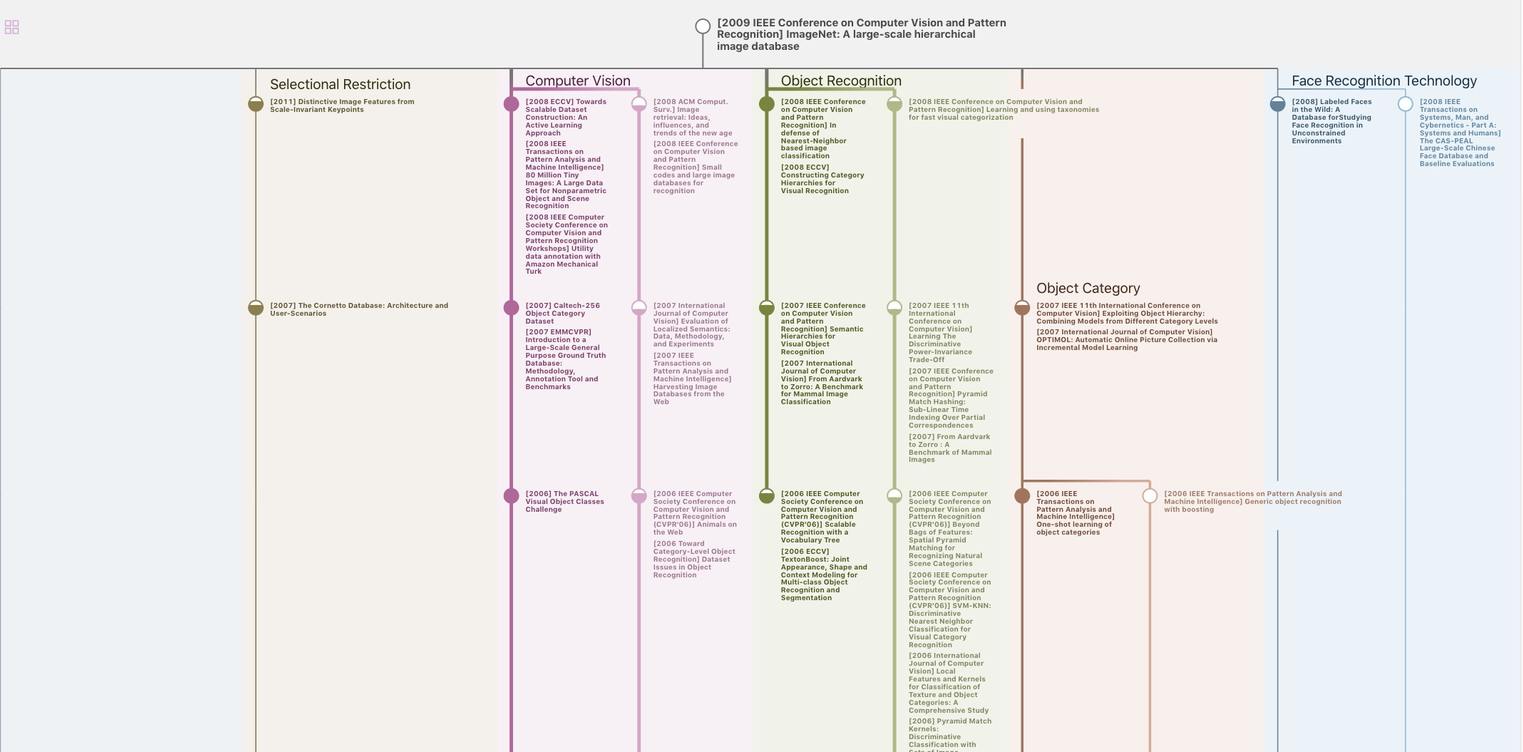
生成溯源树,研究论文发展脉络
Chat Paper
正在生成论文摘要