Provably Convergent Federated Trilevel Learning
AAAI 2024(2024)
摘要
Trilevel learning, also called trilevel optimization (TLO), has been recognized as a powerful modelling tool for hierarchical decision process and widely applied in many machine learning applications, such as robust neural architecture search, hyperparameter optimization, and domain adaptation. Tackling TLO problems has presented a great challenge due to their nested decision-making structure. In addition, existing works on TLO face the following key challenges: 1) they all focus on the non-distributed setting, which may lead to privacy breach; 2) they do not offer any non-asymptotic convergence analysis which characterizes how fast an algorithm converges. To address the aforementioned challenges, this paper proposes an asynchronous federated trilevel optimization method to solve TLO problems. The proposed method utilizes u-cuts to construct a hyper-polyhedral approximation for the TLO problem and solve it in an asynchronous manner. We demonstrate that the proposed u-cuts are applicable to not only convex functions but also a wide range of non-convex functions that meet the u-weakly convex assumption. Furthermore, we theoretically analyze the non-asymptotic convergence rate for the proposed method by showing its iteration complexity to obtain ϵ-stationary point is upper bounded by O(1/ϵ²). Extensive experiments on real-world datasets have been conducted to elucidate the superiority of the proposed method, e.g., it has a faster convergence rate with a maximum acceleration of approximately 80%.
更多查看译文
关键词
ML: Distributed Machine Learning & Federated Learning,ML: Optimization
AI 理解论文
溯源树
样例
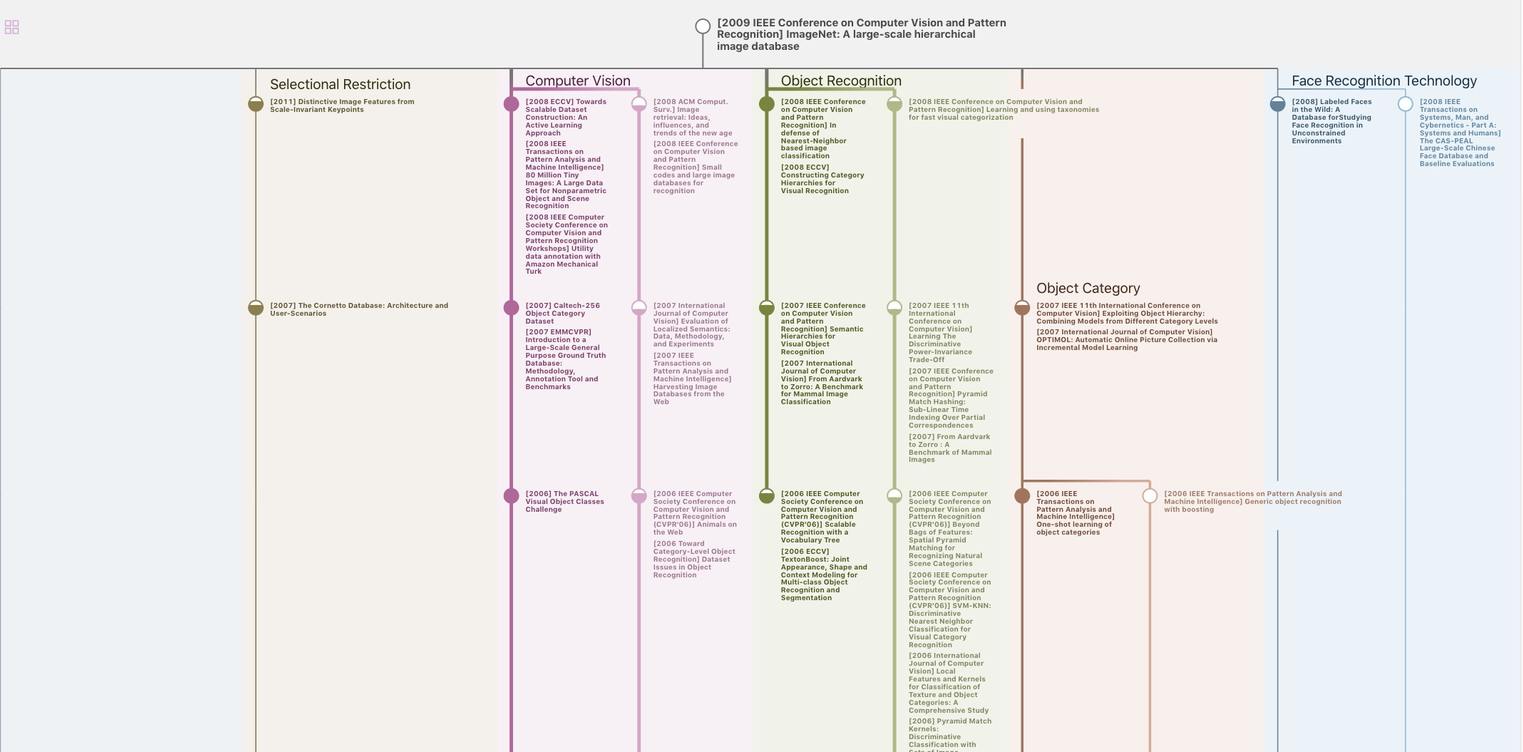
生成溯源树,研究论文发展脉络
Chat Paper
正在生成论文摘要