Understanding the Multi-modal Prompts of the Pre-trained Vision-Language Model
arxiv(2023)
摘要
Prompt learning has emerged as an efficient alternative for fine-tuning
foundational models, such as CLIP, for various downstream tasks. However, there
is no work that provides a comprehensive explanation for the working mechanism
of the multi-modal prompts. In this paper, we conduct a direct analysis of the
multi-modal prompts by asking the following questions: (i) How do the learned
multi-modal prompts improve the recognition performance? (ii) What do the
multi-modal prompts learn? To answer these questions, we begin by isolating the
component of the formula where the prompt influences the calculation of
self-attention at each layer in two distinct ways, , (1) introducing
prompt embeddings makes the [cls] token focus on foreground objects. (2)
the prompts learn a bias term during the update of token embeddings, allowing
the model to adapt to the target domain. Subsequently, we conduct extensive
visualization and statistical experiments on the eleven diverse downstream
recognition datasets. From the experiments, we reveal that the learned prompts
improve the performance mainly through the second way, which acts as the
dataset bias to improve the recognition performance of the pre-trained model on
the corresponding dataset. Based on this finding, we propose the bias tuning
way and demonstrate that directly incorporating the learnable bias outperforms
the learnable prompts in the same parameter settings. In datasets with limited
category information, , EuroSAT, bias tuning surpasses prompt tuning by a
large margin. With a deeper understanding of the multi-modal prompt, we hope
our work can inspire new and solid research in this direction.
更多查看译文
AI 理解论文
溯源树
样例
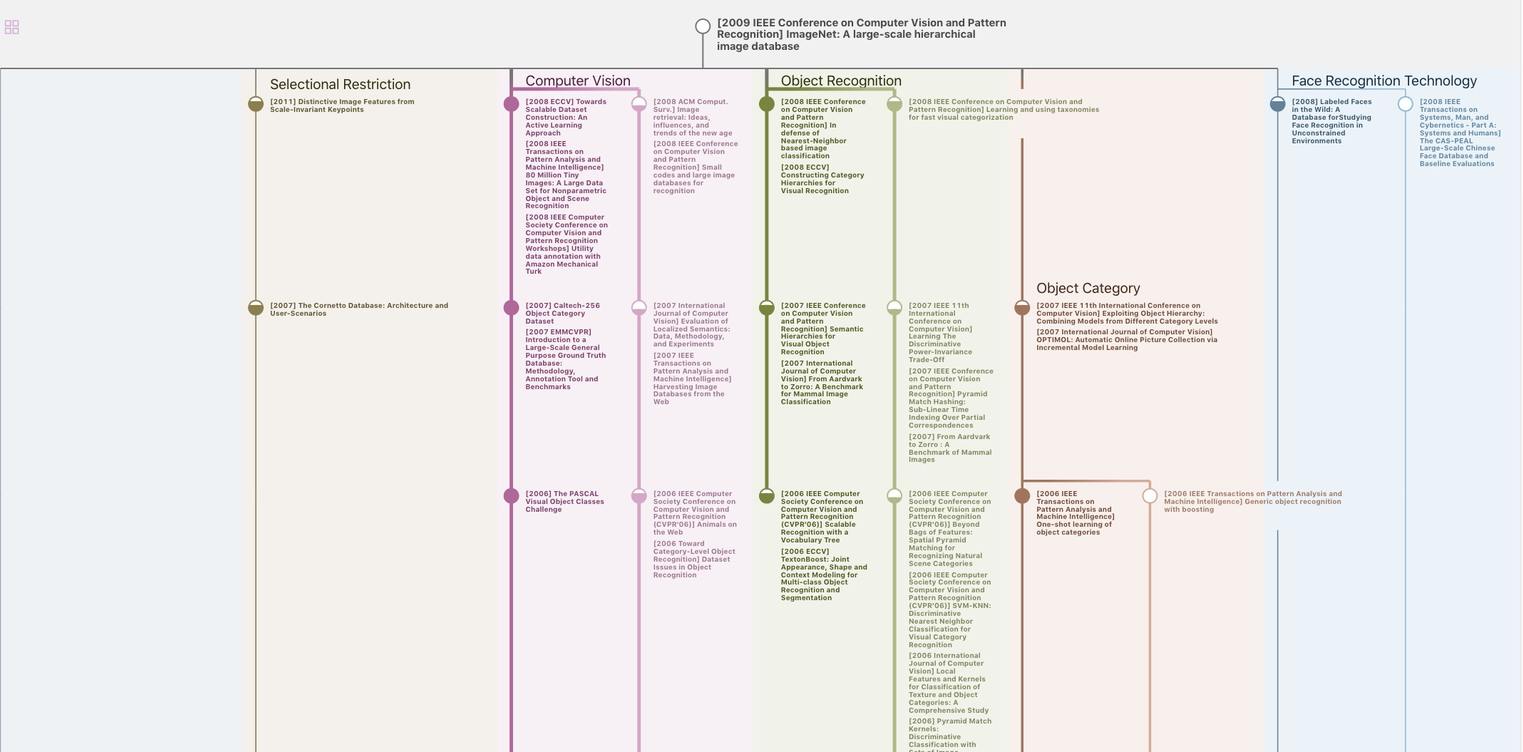
生成溯源树,研究论文发展脉络
Chat Paper
正在生成论文摘要