Stability of Multi-Agent Learning in Competitive Networks: Delaying the Onset of Chaos
AAAI 2024(2024)
摘要
The behaviour of multi agent learning in competitive network games is often studied within the context of zero sum games, in which convergence guarantees may be obtained. However, outside of this class the behaviour of learning is known to display complex behaviours and convergence cannot be always guaranteed. Nonetheless, in order to develop a complete picture of the behaviour of multi agent learning in competitive settings, the zero sum assumption must be lifted.
Motivated by this we study the Q Learning dynamics, a popular model of exploration and exploitation in multi agent learning, in competitive network games. We determine how the degree of competition, exploration rate and network connectivity impact the convergence of Q Learning. To study generic competitive games, we parameterise network games in terms of correlations between agent payoffs and study the average behaviour of the Q Learning dynamics across all games drawn from a choice of this parameter. This statistical approach establishes choices of parameters for which Q Learning dynamics converge to a stable fixed point. Differently to previous works, we find that the stability of Q Learning is explicitly dependent only on the network connectivity rather than the total number of agents. Our experiments validate these findings and show that, under certain network structures, the total number of agents can be increased without increasing the likelihood of unstable or chaotic behaviours.
更多查看译文
关键词
MAS: Multiagent Learning,GTEP: Adversarial Learning,ML: Reinforcement Learning
AI 理解论文
溯源树
样例
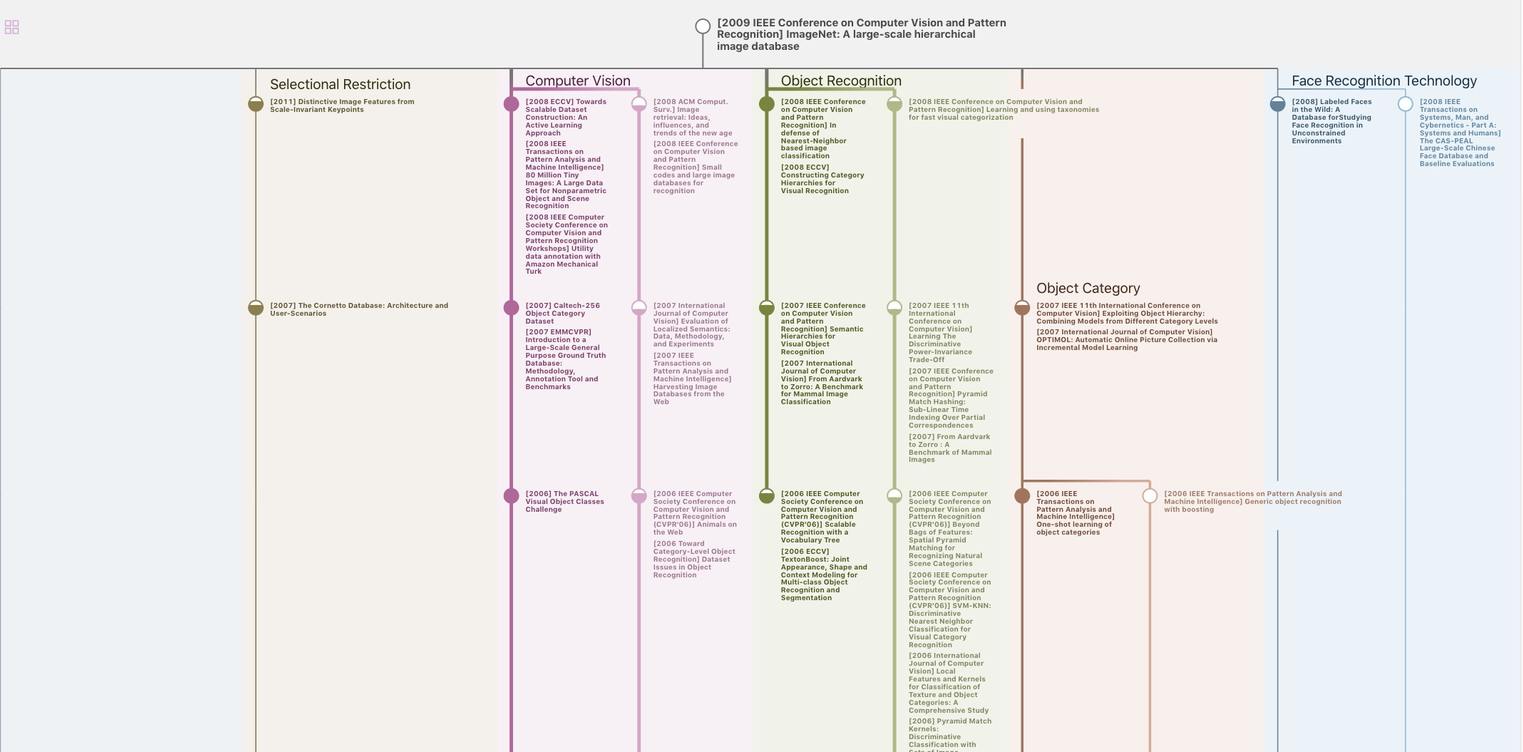
生成溯源树,研究论文发展脉络
Chat Paper
正在生成论文摘要