Dynamics simulation-driven fault diagnosis of rolling bearings using security transfer support matrix machine
RELIABILITY ENGINEERING & SYSTEM SAFETY(2024)
摘要
Transfer fault diagnosis holds paramount significances in safeguarding the reliability and safety of rolling bearings. However, the current studies require massive experiment or field data in the source domain. Besides, sparse data (only single or several samples for each fault) and strong-noise data in the target domain are prone to cause the negative transfer problem. Importantly, most transfer fault diagnosis methods conduct fault classifi-cation in vector space, so the learning ability is weak for matrix-form fault features such as 2D time-frequency images. To address these issues, this paper proposes a novel dynamics simulation-driven fault diagnosis framework with security transfer support matrix machine (STSMM). In this framework, a high-fidelity bearing dynamic model is designed to generate sufficient source-domain data, which greatly reduces the acquisition cost of real data. A new matrix-form transfer learning model, i.e., STSMM, is proposed to effectively utilize the structural information contained in matrix data and achieve the simulation-to-real transfer of fault knowledge. Besides, a security transfer strategy is embedded in STSMM to improve the cross-domain diagnosis performance and theoretically avoid the negative transfer problem caused by sparse data and strong-noise data in the target domain. Experiment results demonstrate the effectiveness and superiority of the proposed framework.
更多查看译文
关键词
Intelligent fault diagnosis,Bearing dynamic simulation,Transfer learning,Support matrix machine,Negative transfer
AI 理解论文
溯源树
样例
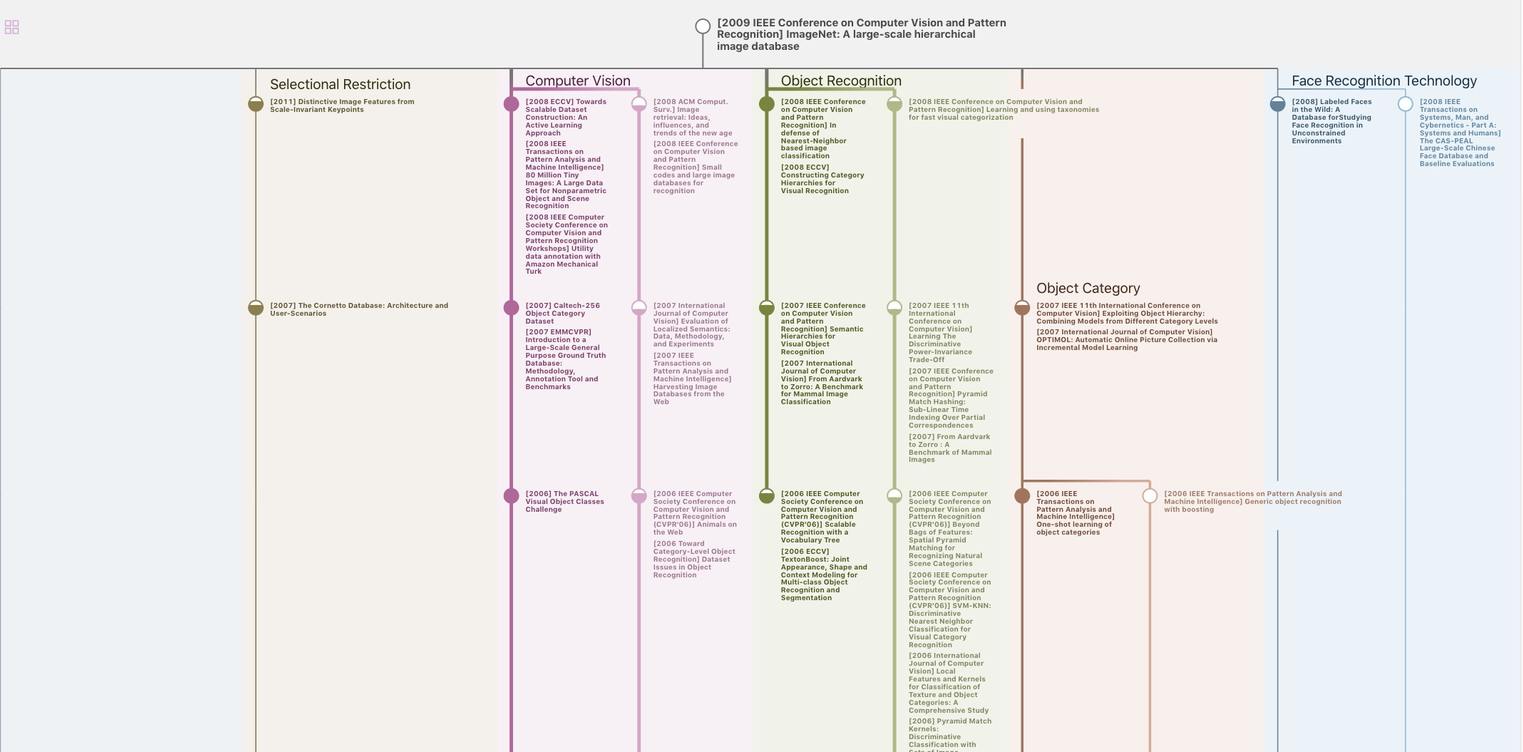
生成溯源树,研究论文发展脉络
Chat Paper
正在生成论文摘要