SideNet: Learning representations from interactive side information for zero-shot Chinese character recognition
PATTERN RECOGNITION(2024)
摘要
Existing methods for zero-shot Chinese character recognition usually exploit a single type of side information such as radicals, glyphs, or strokes to establish a mapping with the input characters for the recognition of unseen categories. However, these approaches have two limitations. Firstly, the mappings are inefficient owing to their complexity. Some existing methods design radical-level mappings using a non-differentiable dictionary matching strategy, whereas others construct sophisticated embeddings to map seen and unseen characters into a unified latent space. Although the latter approach is straightforward, it lacks a learnable scheme for explicit structure construction. Secondly, the complementarity within multiple types of side information has not been effectively explored. For example, the radicals provide structural knowledge at an abstract level, whereas glyphs offer detailed information on their figurative counterparts. To this end, we propose a new method called SideNet that jointly learns character-level representations assisted by two types of interactive side information: radicals and glyphs. SideNet contains a structural conversion module that extracts radical knowledge via dimensional decomposition, and a spatial conversion module that encodes the radical counting map to produce an interactive outcome between radicals and glyph. Finally, we propose a new classifier that integrates the converted features by a similarity-guided fusion mechanism. To the best of our knowledge, this study represents the first attempt to integrate these two types of side information and explore a joint representation for zero-shot learning. Experiments show that SideNet consistently outperforms existing methods by a significant margin in diverse scenarios, including handwriting, printed art, natural scenes, and ancient Chinese characters, which demonstrates the potential of joint learning with multiple types of side information.
更多查看译文
关键词
Optical character recognition,Chinese character recognition,Zero shot learning,Open set recognition
AI 理解论文
溯源树
样例
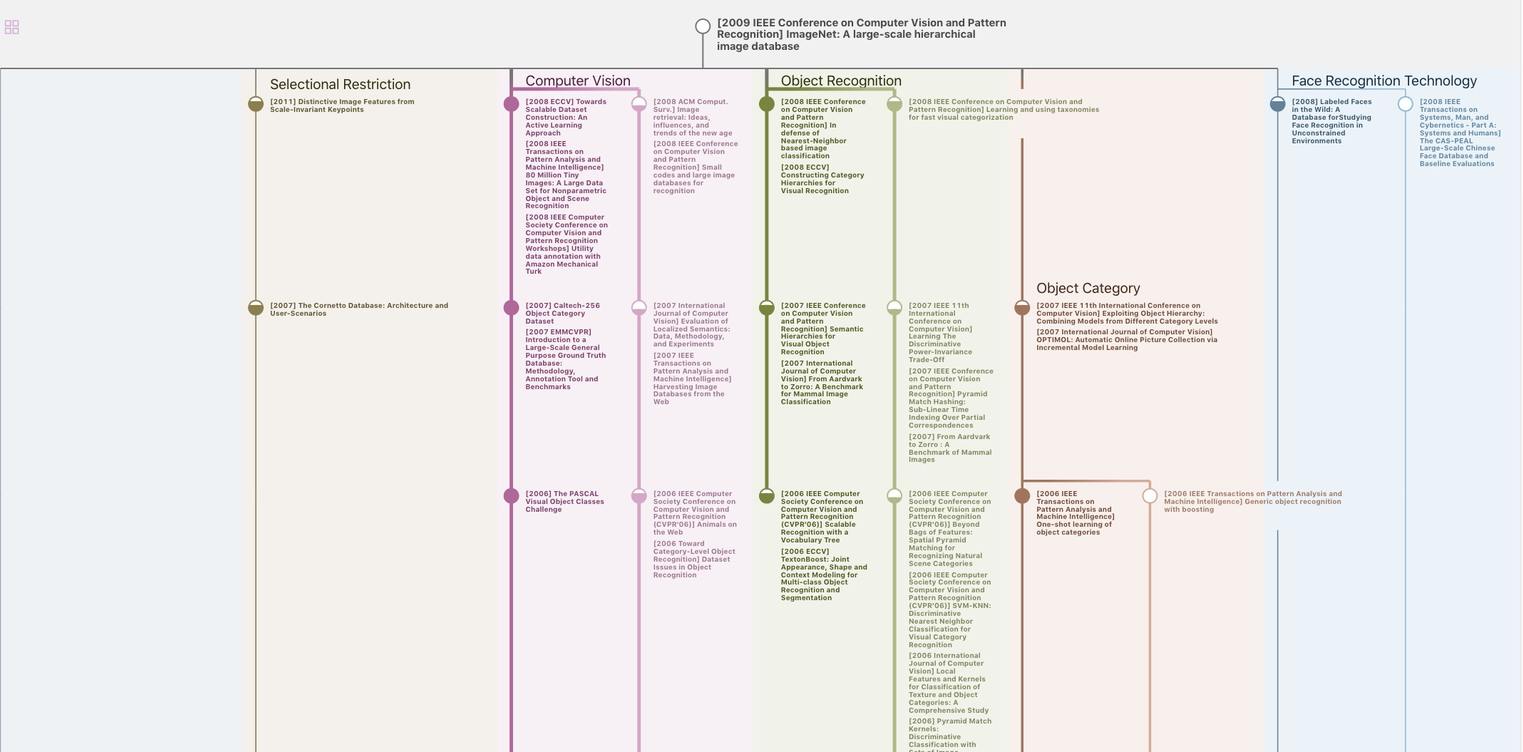
生成溯源树,研究论文发展脉络
Chat Paper
正在生成论文摘要