Multi-Agent Reinforcement Learning for Distributed Resource Allocation in Cell-Free Massive MIMO-Enabled Mobile Edge Computing Network
IEEE TRANSACTIONS ON VEHICULAR TECHNOLOGY(2023)
摘要
To support the newly introduced multimedia services with ultra-low latency and extensive computation requirements, resource-constrained end-user devices should utilize the ubiquitous computing resources available at network edge for augmenting on-board (local) processing with edge computing. In this regard, the capability of cell-free massive MIMO to provide reliable access links by guaranteeing uniform quality of service without cell edge can be exploited for a seamless parallel computing. Taking this into account, we formulate a joint communication and computing resource allocation (JCCRA) problem for a cell-free massive MIMO-enabled mobile edge computing (MEC) network with the objective of minimizing the total energy consumption of the users while meeting the ultra-low delay constraints. To derive efficient and adaptive JCCRA scheme robust to network dynamics, we present a distributed solution approach based on cooperative multi-agent reinforcement learning. The simulation results demonstrate that the proposed distributed approach can achieve comparable performance to a centralized deep deterministic policy gradient (DDPG)-based target benchmark, without incurring additional overhead and time cost. It is also shown that our approach significantly outperforms heuristic baselines in terms of energy efficiency, roughly up to 5 times less total energy consumption. Furthermore, we demonstrate substantial performance improvement compared to cellular MEC systems.
更多查看译文
关键词
Joint communication and computing resource allocation (JCCRA),mobile edge computing,cell-free massive MIMO,multi-agent reinforcement learning
AI 理解论文
溯源树
样例
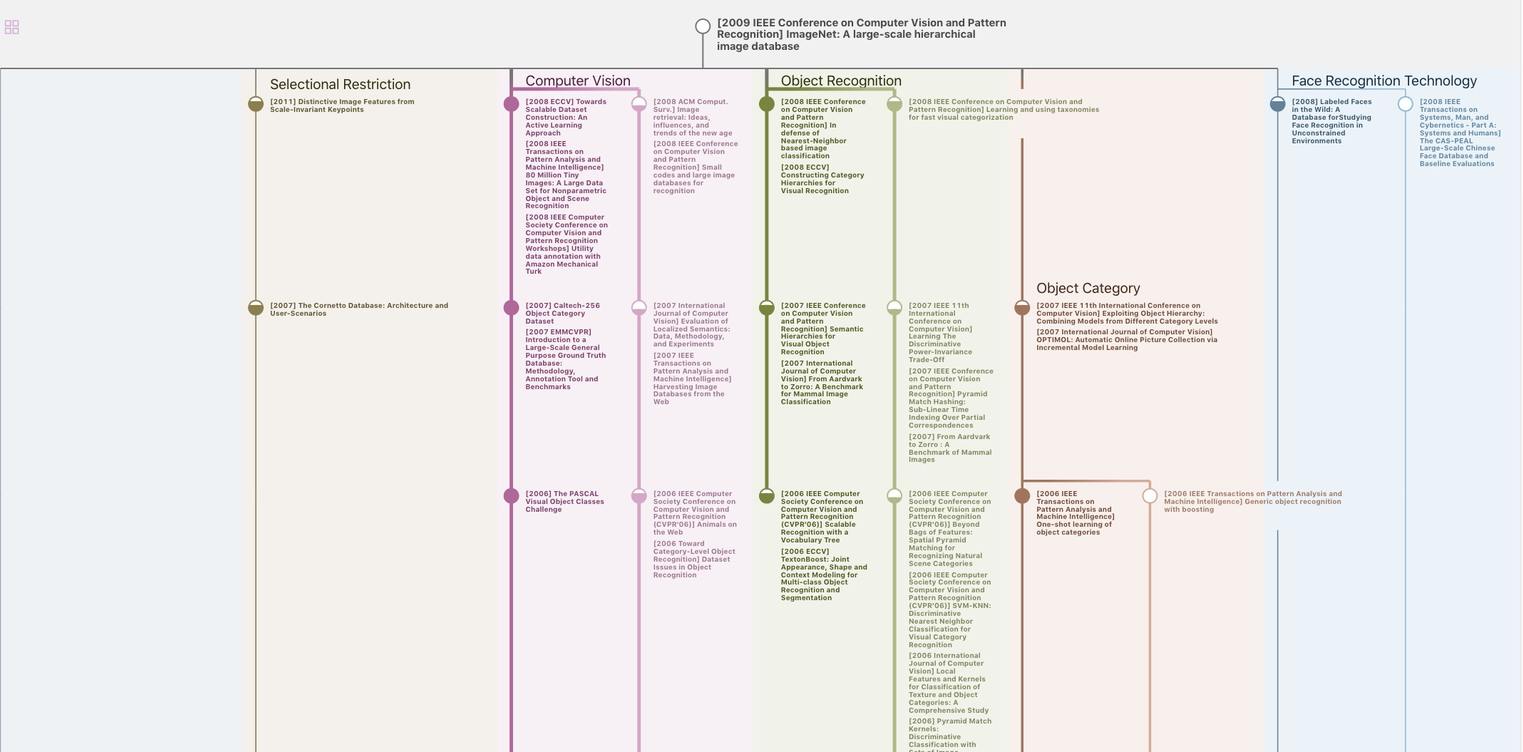
生成溯源树,研究论文发展脉络
Chat Paper
正在生成论文摘要