Visual Comparison of Text Sequences Generated by Large Language Models
2023 IEEE VISUALIZATION IN DATA SCIENCE, VDS(2023)
摘要
Causal language models have emerged as the leading technology for automating text generation tasks. Although these models tend to produce outputs that resemble human writing, they still suffer from quality issues (e.g., social biases). Researchers typically use automatic analysis methods to evaluate the model limitations, such as statistics on stereotypical words. Since different types of issues are embedded in the model parameters, the development of automated methods that capture all relevant aspects remains a challenge. To tackle this challenge, we propose a visual analytics approach that supports the exploratory analysis of text sequences generated by causal language models. Our approach enables users to specify starting prompts and effectively groups the resulting text sequences. To this end, we leverage a unified, ontology-driven embedding space, serving as a shared foundation for the thematic concepts present in the generated text sequences. Visual summaries provide insights into various levels of granularity within the generated data. Among others, we propose a novel comparison visualization that slices the embedding space and represents the differences between two prompt outputs in a radial layout. We demonstrate the effectiveness of our approach through case studies, showcasing its potential to reveal model biases and other quality issues.
更多查看译文
关键词
Causal Language Models,Text Generation,Prompt Output Comparison
AI 理解论文
溯源树
样例
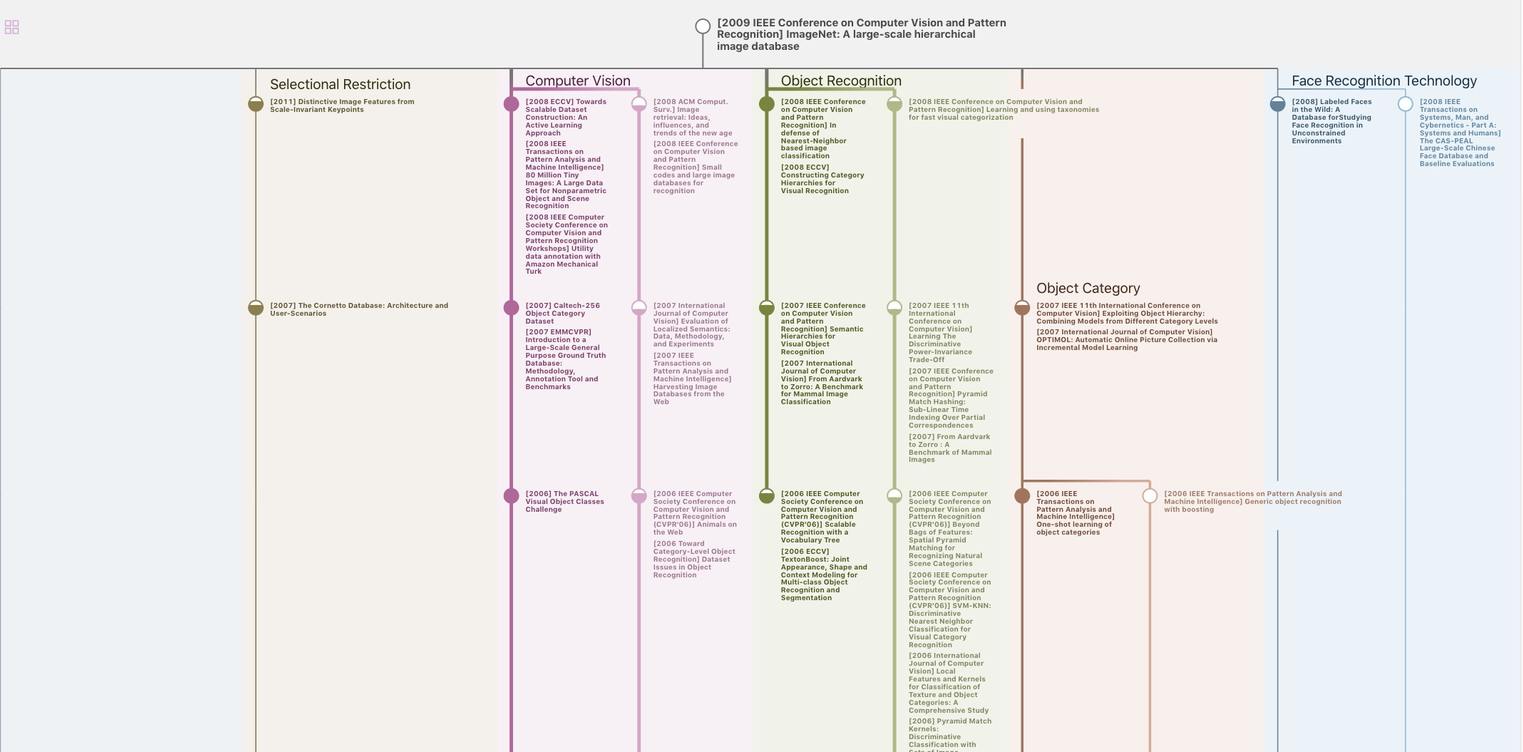
生成溯源树,研究论文发展脉络
Chat Paper
正在生成论文摘要