Value of Information and Timing-aware Scheduling for Federated Learning
CoRR(2023)
摘要
Data possesses significant value as it fuels advancements in AI. However,
protecting the privacy of the data generated by end-user devices has become
crucial. Federated Learning (FL) offers a solution by preserving data privacy
during training. FL brings the model directly to User Equipments (UEs) for
local training by an access point (AP). The AP periodically aggregates trained
parameters from UEs, enhancing the model and sending it back to them. However,
due to communication constraints, only a subset of UEs can update parameters
during each global aggregation. Consequently, developing innovative scheduling
algorithms is vital to enable complete FL implementation and enhance FL
convergence. In this paper, we present a scheduling policy combining Age of
Update (AoU) concepts and data Shapley metrics. This policy considers the
freshness and value of received parameter updates from individual data sources
and real-time channel conditions to enhance FL's operational efficiency. The
proposed algorithm is simple, and its effectiveness is demonstrated through
simulations.
更多查看译文
关键词
Age of Update,Data Shapely,Federated Learning,Scheduling
AI 理解论文
溯源树
样例
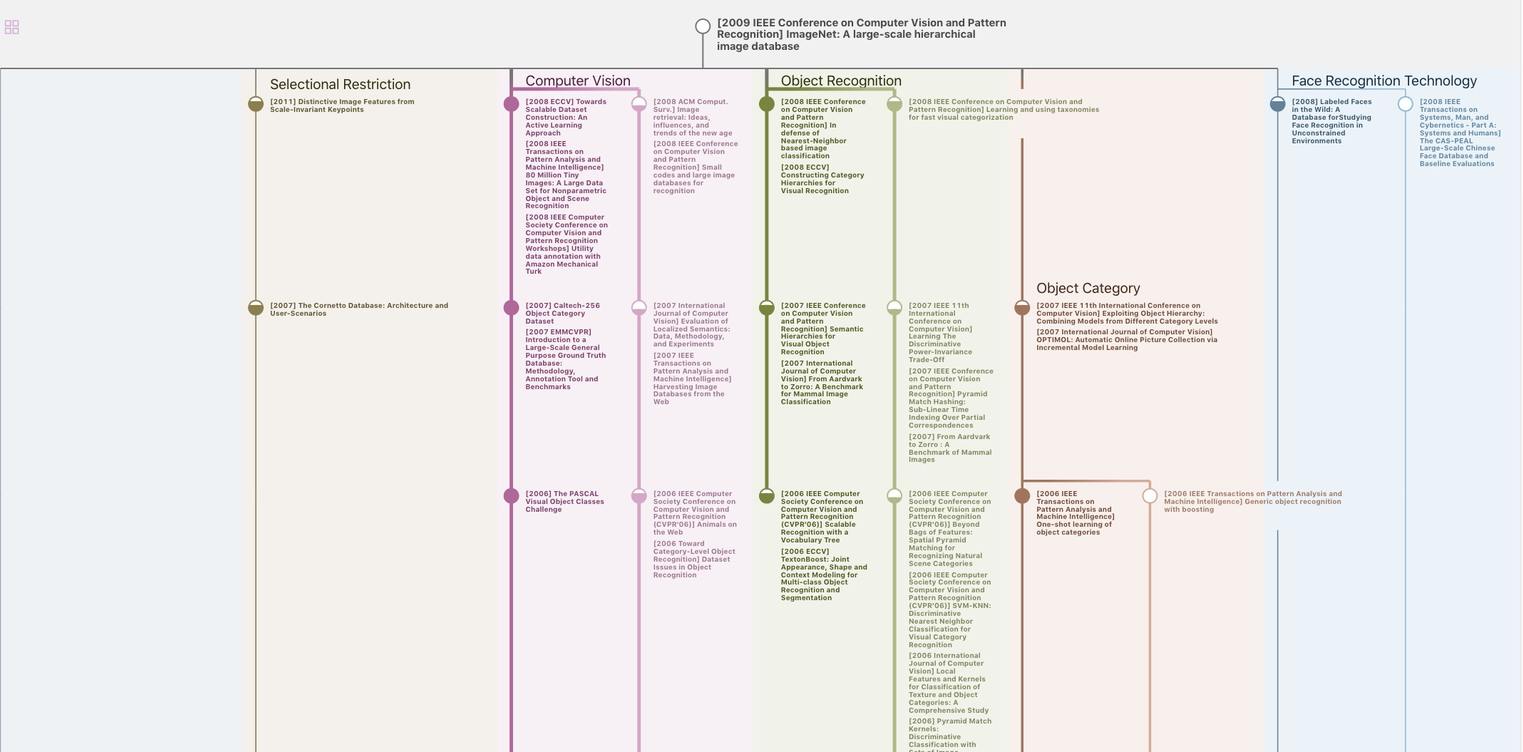
生成溯源树,研究论文发展脉络
Chat Paper
正在生成论文摘要