RedCore: Relative Advantage Aware Cross-Modal Representation Learning for Missing Modalities with Imbalanced Missing Rates
AAAI 2024(2024)
摘要
Multimodal learning is susceptible to modality missing, which poses a major obstacle for its practical applications and, thus, invigorates increasing research interest. In this paper, we investigate two challenging problems: 1) when modality missing exists in the training data, how to exploit the incomplete samples while guaranteeing that they are properly supervised? 2) when the missing rates of different modalities vary, causing or exacerbating the imbalance among modalities, how to address the imbalance and ensure all modalities are well-trained. To tackle these two challenges, we first introduce the variational information bottleneck (VIB) method for the cross-modal representation learning of missing modalities, which capitalizes on the available modalities and the labels as supervision. Then, accounting for the imbalanced missing rates, we define relative advantage to quantify the advantage of each modality over others. Accordingly, a bi-level optimization problem is formulated to adaptively regulate the supervision of all modalities during training. As a whole, the proposed approach features Relative advantage aware Cross-modal representation learning (abbreviated as RedCore) for missing modalities with imbalanced missing rates. Extensive empirical results demonstrate that RedCore outperforms competing models in that it exhibits superior robustness against either large or imbalanced missing rates. The code is available at: https://github.com/sunjunaimer/RedCore.
更多查看译文
关键词
ML: Multimodal Learning,ML: Applications,NLP: Language Grounding & Multi-modal NLP
AI 理解论文
溯源树
样例
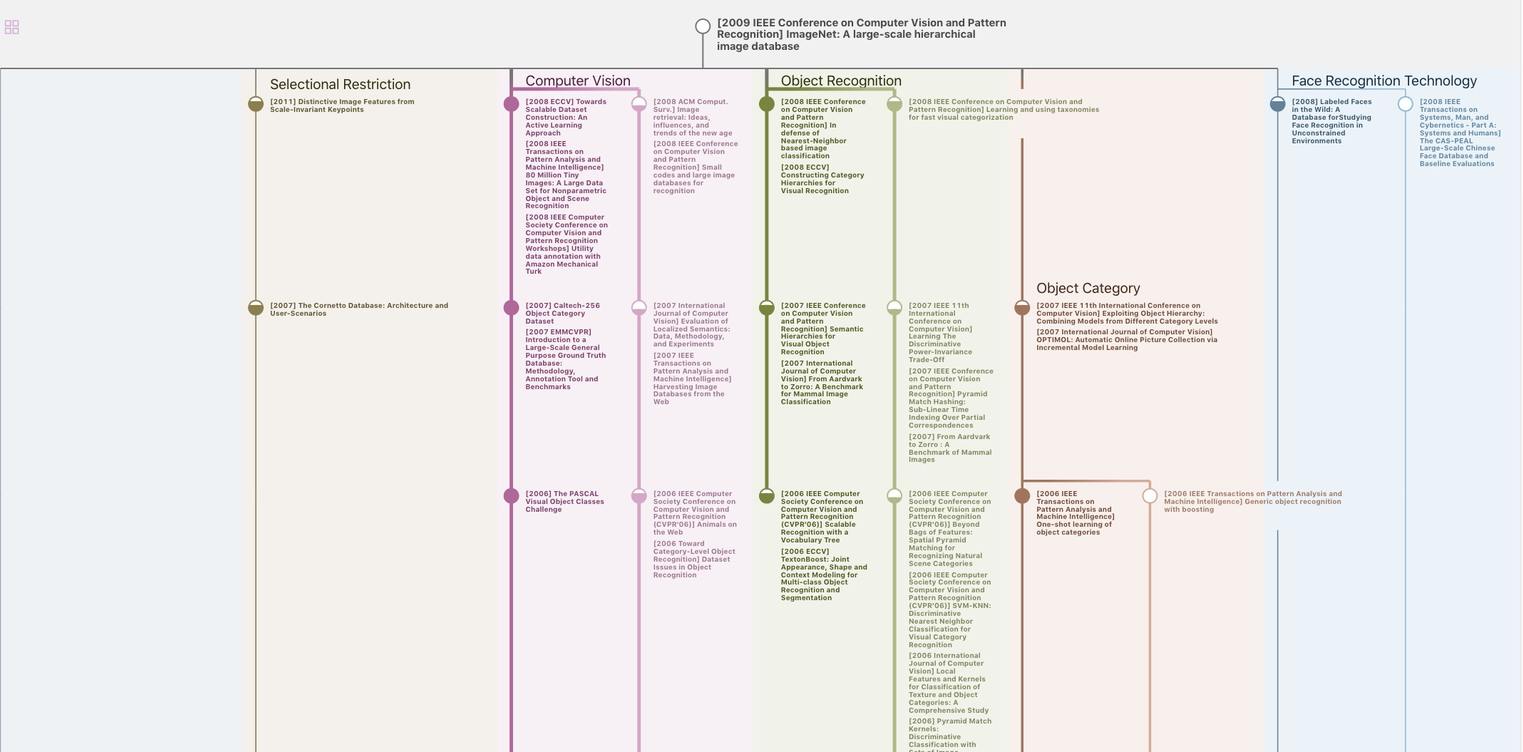
生成溯源树,研究论文发展脉络
Chat Paper
正在生成论文摘要