Beyond Empirical Windowing: An Attention-Based Approach for Trust Prediction in Autonomous Vehicles
CoRR(2023)
摘要
Humans' internal states play a key role in human-machine interaction, leading
to the rise of human state estimation as a prominent field. Compared to swift
state changes such as surprise and irritation, modeling gradual states like
trust and satisfaction are further challenged by label sparsity: long
time-series signals are usually associated with a single label, making it
difficult to identify the critical span of state shifts. Windowing has been one
widely-used technique to enable localized analysis of long time-series data.
However, the performance of downstream models can be sensitive to the window
size, and determining the optimal window size demands domain expertise and
extensive search. To address this challenge, we propose a Selective Windowing
Attention Network (SWAN), which employs window prompts and masked attention
transformation to enable the selection of attended intervals with flexible
lengths. We evaluate SWAN on the task of trust prediction on a new multimodal
driving simulation dataset. Experiments show that SWAN significantly
outperforms an existing empirical window selection baseline and neural network
baselines including CNN-LSTM and Transformer. Furthermore, it shows robustness
across a wide span of windowing ranges, compared to the traditional windowing
approach.
更多查看译文
关键词
Trust in automation,Attention,Time-series
AI 理解论文
溯源树
样例
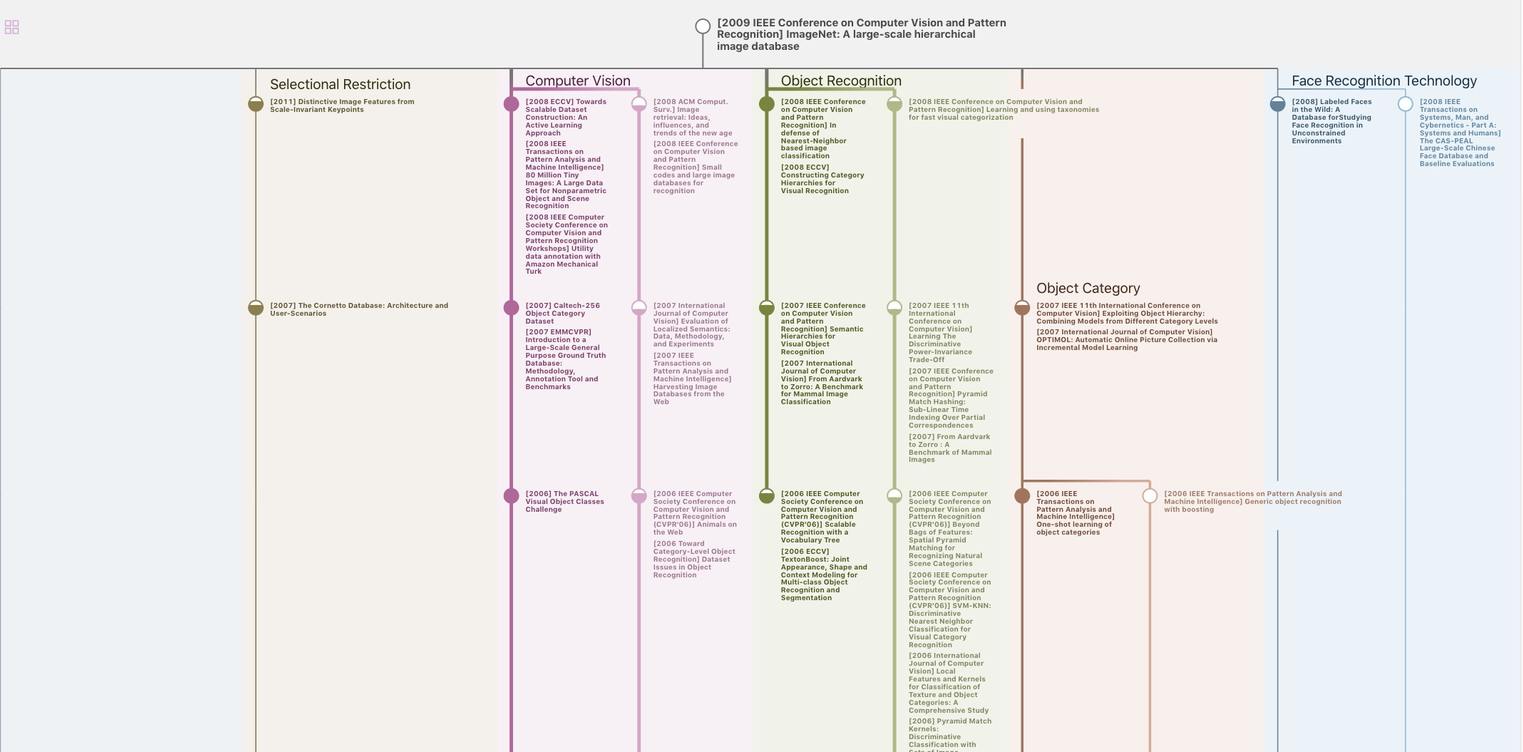
生成溯源树,研究论文发展脉络
Chat Paper
正在生成论文摘要