Comparing Quadrotor Control Policies for Zero-Shot Reinforcement Learning under Uncertainty and Partial Observability
2023 IEEE/RSJ INTERNATIONAL CONFERENCE ON INTELLIGENT ROBOTS AND SYSTEMS (IROS)(2023)
摘要
To alleviate the sample complexity of reinforcement learning algorithms, simulations are a common approach to train control policies before deploying the policy on a real-world robot. However, a gap between simulation and reality generally persists, which endorses the aim to train robust policies already in simulation such that those can be transferred to a real robot at a high success rate. In this paper, we investigate history-dependent policies for drone control in the context of zero-shot transfer learning, where the training is conducted exclusively in simulation. We compare policies represented by feed-forward neural networks with recurrent neural networks and assess both performance and robustness on a real-world quadrotor. Furthermore, we study if an end-to-end learned representation can control a quadrotor based on raw onboard-sensor information only, rendering accurate state estimation from a Kalman filter obsolete. Our results show that recurrent control policies achieve similar performance and robustness as feed-forward policies when acting on state estimates. With raw sensory data, however, recurrent networks offer higher success rates for sim-to-real transfer than feed-forward networks. We also find that recurrent architectures are advantageous when system parameters such as latency are uncertain.
更多查看译文
AI 理解论文
溯源树
样例
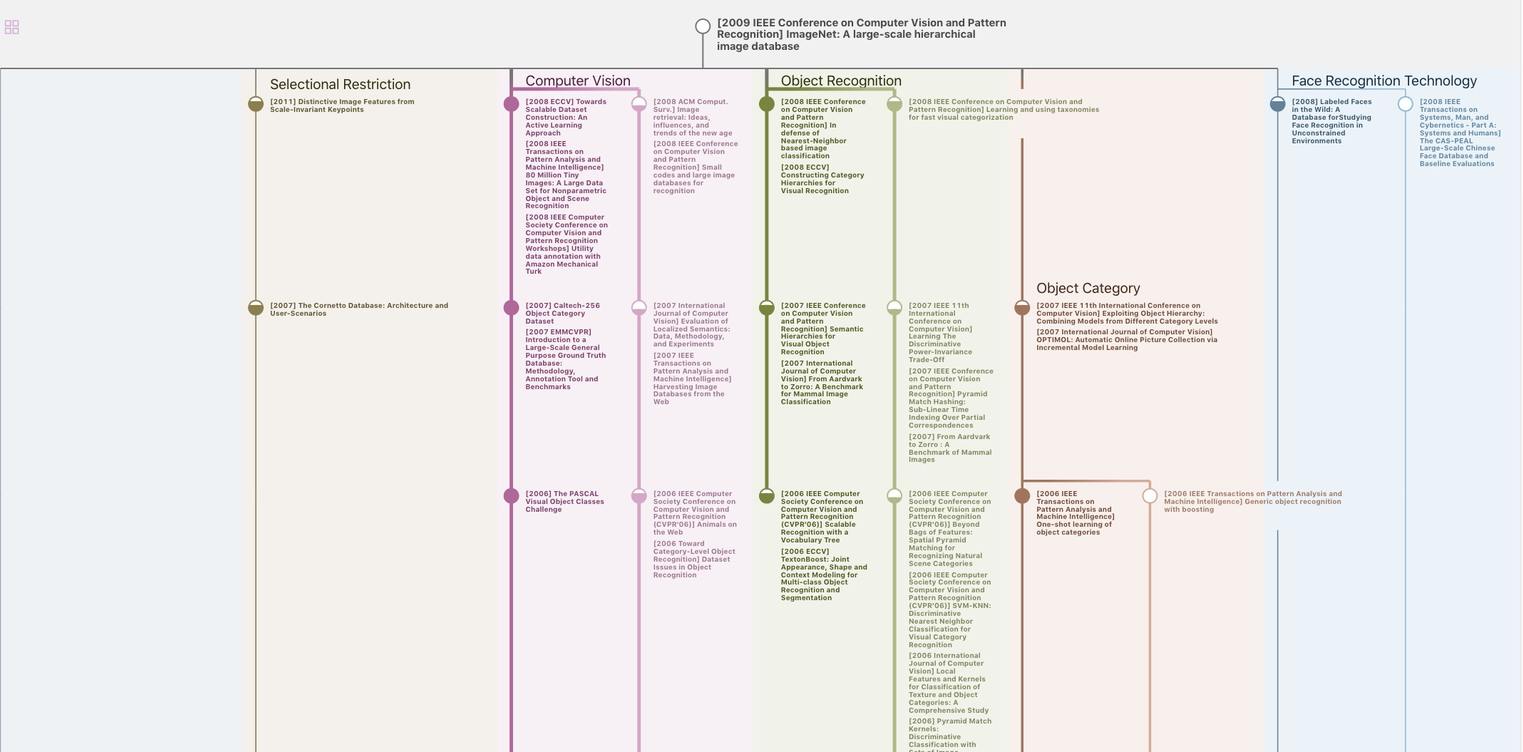
生成溯源树,研究论文发展脉络
Chat Paper
正在生成论文摘要