Steformer: Efficient Stereo Image Super-Resolution With Transformer
IEEE TRANSACTIONS ON MULTIMEDIA(2023)
摘要
With the rapid development of stereoscopic vision applications, stereo image processing techniques have attracted increasing attention in both academic and industrial communities. In this paper, we study the fundamental stereo image super-resolution (SR) problem, which aims to recover high-resolution stereo images from low-resolution (LR) stereo images. Since disparities between stereo images vary significantly, convolutional network-based stereo image SR methods show a limitation in capturing long-range dependencies. To address this problem, this paper proposes to leverage the capability of self-attention in Transformers to efficiently capture reliable stereo correspondence and incorporate cross-view information for stereo image SR. Our model, named Steformer, consists of three parts: cross attentive feature extraction, cross-to-intra information integration and high-quality image reconstruction. In particular, the cross attentive feature extraction module employs residual cross Steformer blocks (RCSB) for long-range cross-view information extraction. Then, the cross-to-intra information integration module exploits cross-view and intra-view information using cross-to-intra attention mechanism (C2IAM). Finally, residual Steformer blocks (RSB) are designed for feature pre-processing in high-quality image reconstruction. Extensive experiments show that Steformer achieves significant improvements over state-of-the-art approaches on both quantitative and qualitative evaluations, while the total number of parameters can be reduced by up to 40.71%.
更多查看译文
关键词
Superresolution,Transformers,Image reconstruction,Feature extraction,Task analysis,Convolution,Computer architecture,Stereo image processing,image super-resolution,transformer
AI 理解论文
溯源树
样例
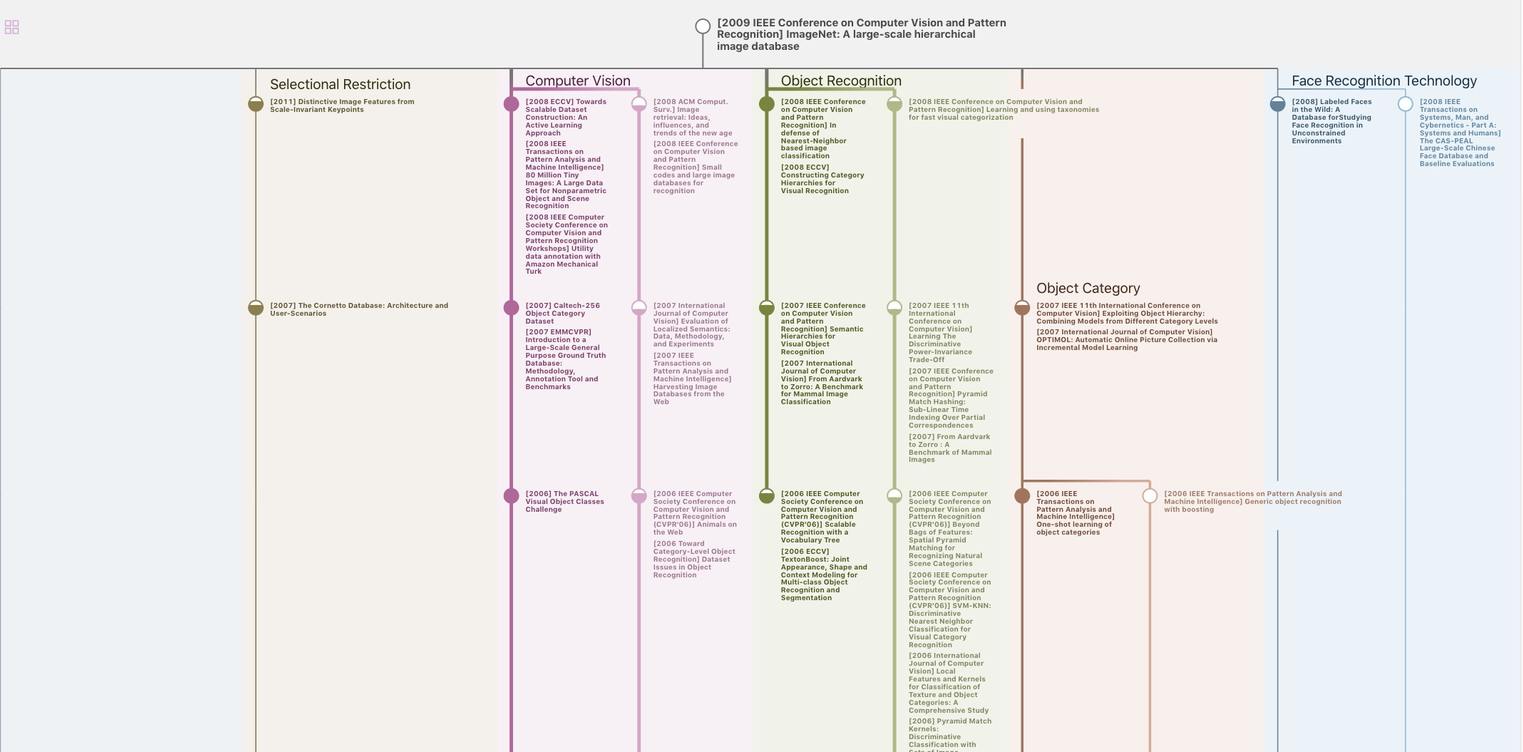
生成溯源树,研究论文发展脉络
Chat Paper
正在生成论文摘要