Maximizing Ranking-Aware Recommendation Quality for Low-Complexity Network-Friendly Recommendation
2023 IEEE 98TH VEHICULAR TECHNOLOGY CONFERENCE, VTC2023-FALL(2023)
摘要
Network-friendly recommendation (NFR) has been identified as a promising method to facilitate the network performance. However, the current NFR approaches introduce non-negligible computation overhead and primarily focus on the content to recommend, while neglecting the impact of ranking among contents, sharing limited recommendation quality. In this paper, we aim to design a recommendation re-ranking algorithm to maximize the ranking-aware recommendation quality while satisfying the network constraint. We formulate the problem as an integer programming (IP) problem that maximizes the mean reciprocal rank of the baseline recommendation under network constraint. To address the computational challenge posed by the large-scale IP problem, we propose a low-complexity algorithm that enables real-time NFR. Specifically, our approach reduces the computational complexity by re-ranking only the measurement list, instead of the entire content list. Empirical results on real-world datasets demonstrate that compared with state-of-the-art NFR baselines, the proposed scheme delivers an improvement of more than 4.8% in both precision and coverage, while reducing 97.5% computation time.
更多查看译文
关键词
Network-friendly Recommendation,Re-ranking Optimization,Integer Programming
AI 理解论文
溯源树
样例
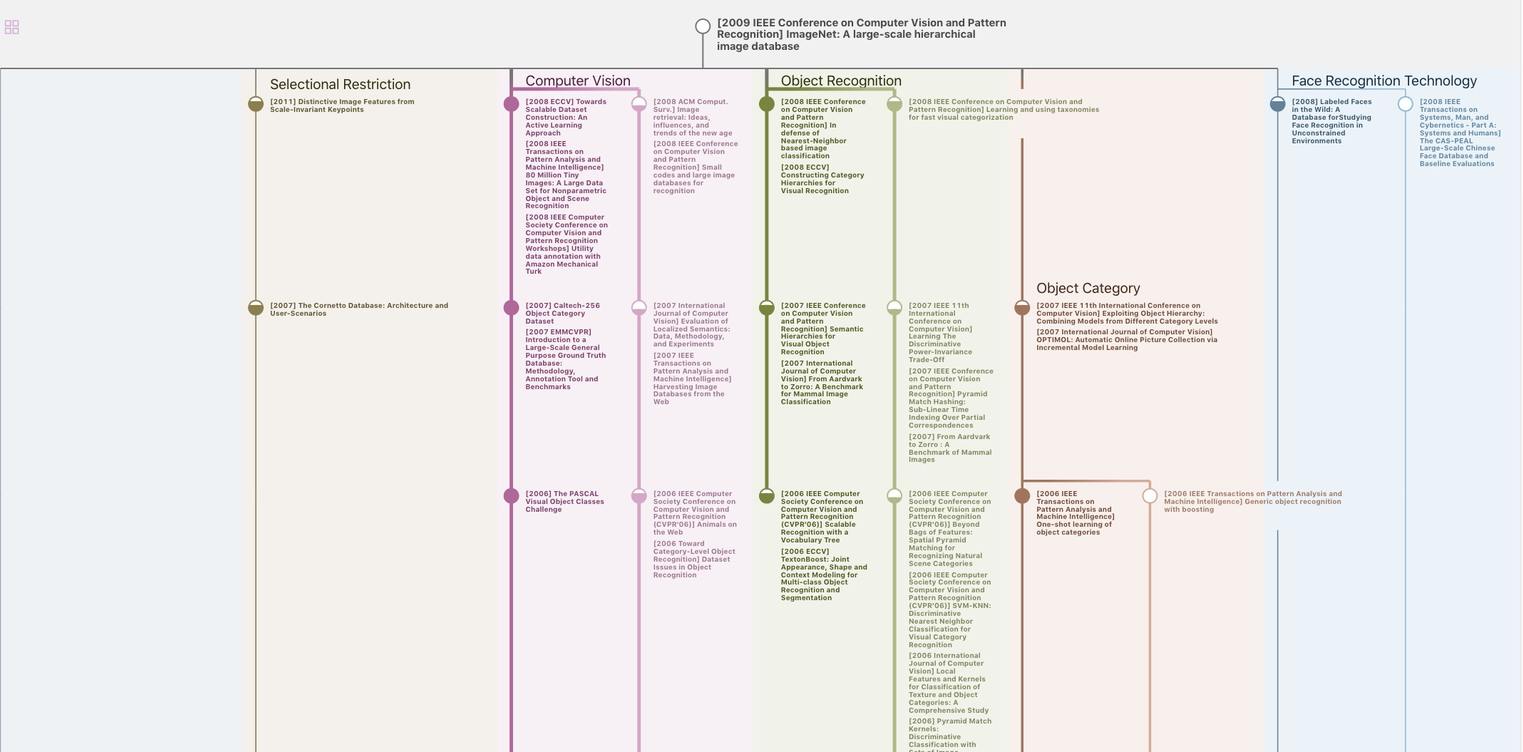
生成溯源树,研究论文发展脉络
Chat Paper
正在生成论文摘要