Spectral Privacy Detection on Black-box Graph Neural Networks
2023 IEEE 98TH VEHICULAR TECHNOLOGY CONFERENCE, VTC2023-FALL(2023)
摘要
Graph neural networks (GNNs) have emerged as a promising approach for real-world applications such as the Internet of Vehicles (IoV) or social networks, yet the spectral properties of the GNN model are under-explored, especially from a privacy detection and protection perspective. This article proposes a general framework to distinguish black-box GNN based on spectral filtering performance. The framework offers a new perspective for spectral privacy detection with a generated graph sample set. Specifically, by introducing a set of graphs of different connectivity, we could access the spectral characteristics of black-box GNN. Furthermore, we could infer information about its underlying structure, such as message passing or positional embedding. Overall, our work sheds light on the spectral properties of GNNs and opens up new avenues for analyzing their behavior in real-world applications.
更多查看译文
关键词
GNN,Spectral,Black-box,Filtration,Detection
AI 理解论文
溯源树
样例
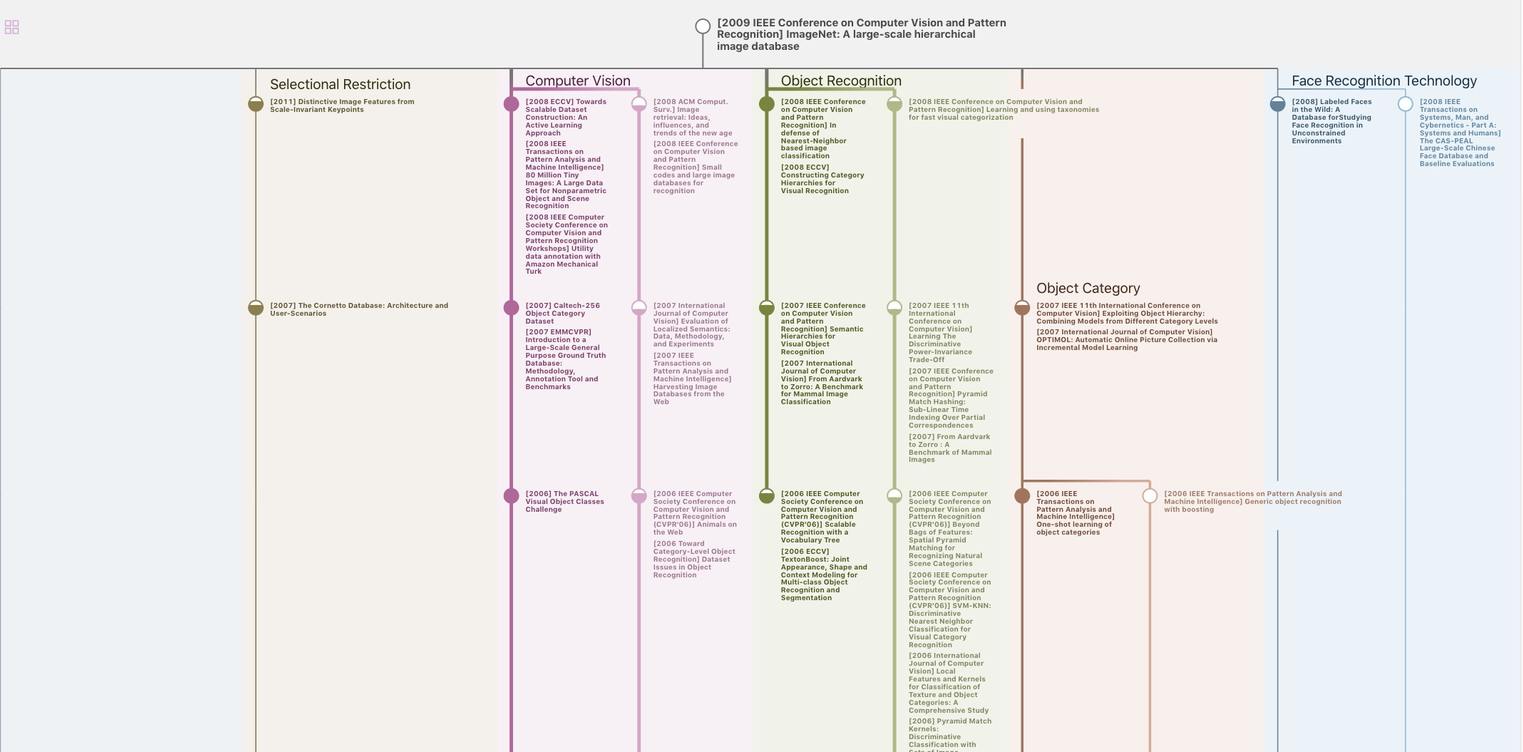
生成溯源树,研究论文发展脉络
Chat Paper
正在生成论文摘要