Automatic Neural Network Design of Scene-customization for Massive MIMO CSI Feedback
2023 IEEE 98TH VEHICULAR TECHNOLOGY CONFERENCE, VTC2023-FALL(2023)
摘要
Deep learning has revolutionized the design of channel state information (CSI) feedback modules in wireless communication. However, designing an optimal neural network (NN) architecture for CSI feedback can be laborious and time-consuming, especially for customized networks targeting different scenarios. To address this challenge, this paper proposes the use of Neural Architecture Search (NAS) to automatically generate scenario-specific CSI feedback neural network architectures. By employing automated machine learning and gradient-based NAS, an efficient and cost-effective architecture design process is achieved with reduced reliance on expert knowledge and design time, thus lowering the design threshold. This approach leverages implicit scenario knowledge and integrates it into the scenario customization process in a data-driven manner, fully harnessing the potential of deep learning in a given scenario. Experimental results demonstrate that the generated architecture called Auto-CsiNet outperforms manually designed models in terms of reconstruction performance (improvement by approximately 14%) and complexity reduction (approximately 50%), highlighting the effectiveness of NAS-based automated solutions.
更多查看译文
关键词
Massive MIMO,CSI feedback,deep learning,neural network architecture search
AI 理解论文
溯源树
样例
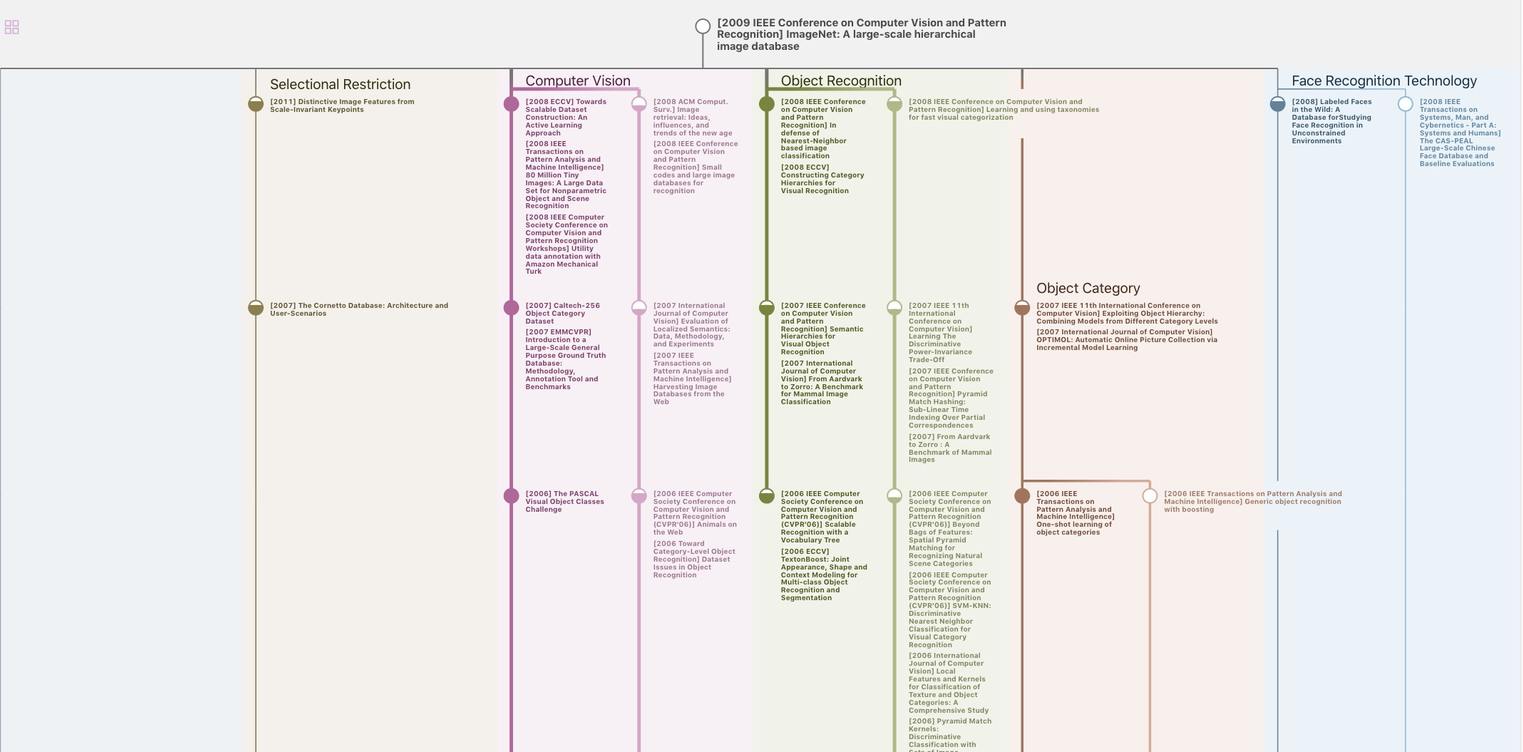
生成溯源树,研究论文发展脉络
Chat Paper
正在生成论文摘要