Coded Distributed Computing For Vehicular Edge Computing With Dual -Function Radar Communication
2023 IEEE 98TH VEHICULAR TECHNOLOGY CONFERENCE, VTC2023-FALL(2023)
摘要
In this paper, we propose a coded distributed computing (CDC) -based vehicular edge computing (VEC) framework. The framework allows a task vehicle (TV) equipped with the dual-function radar communication (DFRC) to offload its computing tasks to the nearby service vehicles (SVs) using the (rn, k) maximum distance separable (MDS). The framework is thus able to address the straggler effect that is typically caused by the high mobility of the vehicles. We then formulate an optimization problem for the TV that aims to i) minimize the overall computing latency, ii) minimize the offloading cost, and iii) maximize the radar range subject to the connection duration. For this, we optimize the MDS parameters, i.e., the number of selected SVs (rn) and the number of subtasks for coding (k), and the fractions of power allocated to the radar and communication functions. Under the high dynamic vehicular environment, the uncertainty of the SVs' computing resource and networking resources, we propose a deep reinforcement learning (DRL) algorithm based on Double Deep Q -Network (DDQN) to solve the TV's problem. To further improve the performance, we propose to incorporate a parameter norm penalty in the loss function. Simulation results show that the proposed DDQN algorithm outperforms both the DQN algorithm and the non-learning algorithm in terms of computation latency, radar range, and offloading cost.
更多查看译文
关键词
Terms Autonomous vehicles,dual-function radar communication,vehicular edge computing,maximum distance separable,double deep Q -network
AI 理解论文
溯源树
样例
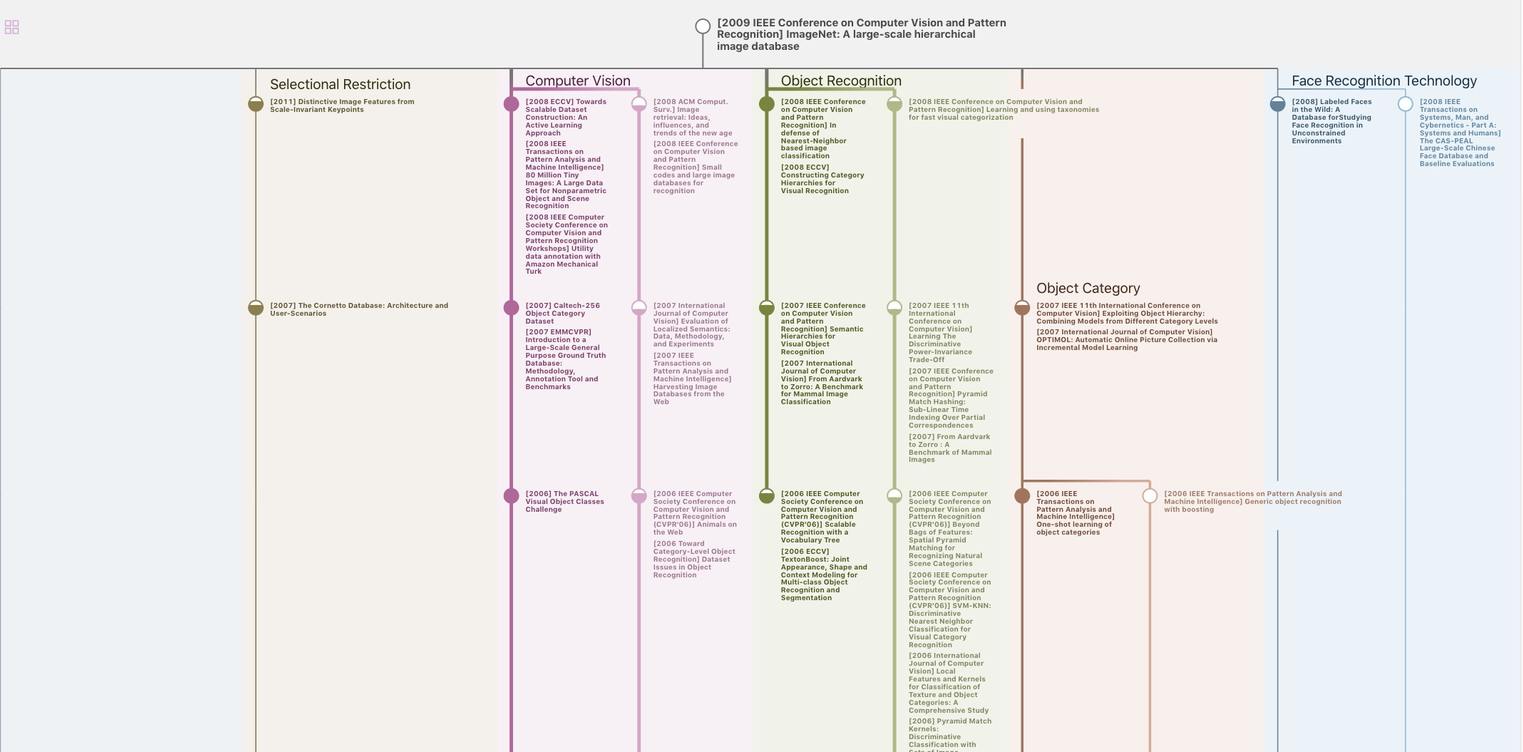
生成溯源树,研究论文发展脉络
Chat Paper
正在生成论文摘要