Federated Learning Based on CTC for Heterogeneous Internet of Things
IEEE INTERNET OF THINGS JOURNAL(2023)
摘要
Federated learning (FL) is a machine learning technique that allows for on-site data collection and processing without sacrificing data privacy and transmission. Heterogeneity is a key challenge in federated settings. Recently, cross-technology communication (CTC) has emerged as a solution for Internet of Things (IoT) heterogeneity, enabling direct communication between different wireless devices without the need for hardware modifications or gateway intervention. For example, a sophisticated WiFi device can serve as a central coordinator for other heterogeneous devices, such as LoRa, ZigBee, Bluetooth, and LTE, leading to more efficient and ubiquitous cross-network information exchange. However, heterogeneous wireless technologies present different data transmission rates and computing resources, making it difficult to achieve high accuracy in predictions due to large amounts of multidimensional data, communication delays, transmission latency, limited processing capacity, and data privacy concerns. In this work, we propose an FL framework based on CTC for heterogeneous IoT applications, called FLCTC. To demonstrate the usability of FLCTC, we implemented FLCTC and a specific solution for forest fire prediction. FLCTC was concretely implemented as a federal deep learning based on long and short-term memory and used for forest fire prediction, addressing the challenge of data characterization in heterogeneous IoT networks. FLCTC promises to improve communication efficiency and prediction accuracy. Our platform-based evaluation results show that FLCTC is feasible, with a recall of 96% and an accuracy of 88%, offering valuable insights into the use of FL with CTC for heterogeneous IoT applications.
更多查看译文
关键词
Cross-technology communication (CTC),federated learning (FL),heterogeneous Internet of Things (IoT) networks
AI 理解论文
溯源树
样例
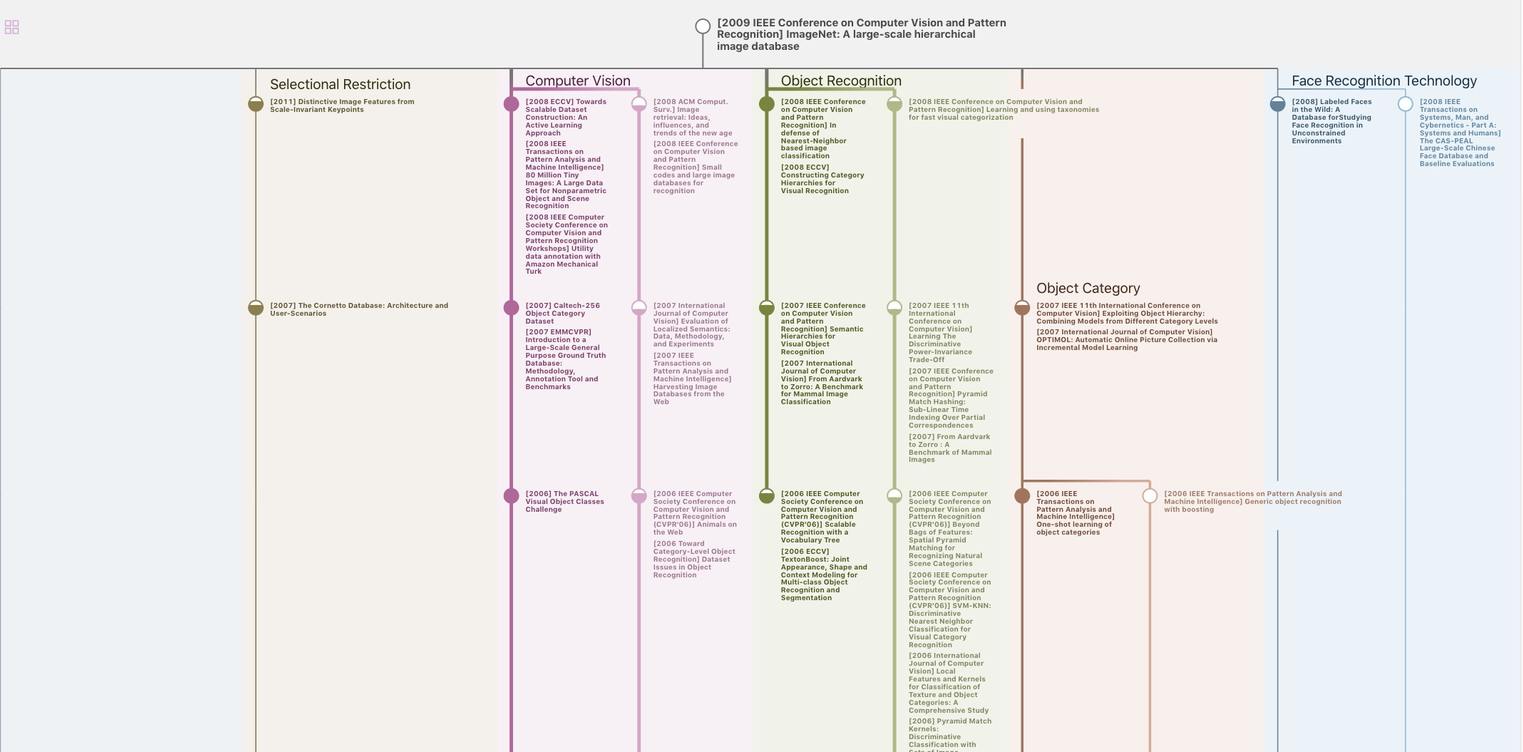
生成溯源树,研究论文发展脉络
Chat Paper
正在生成论文摘要