A machine learning approach for the classification of sports based on a coaches' perspective of environmental, individual and task requirements: A sports profile analysis
JOURNAL OF SPORTS SCIENCES(2023)
摘要
Well-designed talent programmes in sports with a focus on talent identification, orientation, development, and transfer support the engagement of young individuals and the pursuit of elite performance. To facilitate these processes, an analysis of task, environmental and individual characteristics per sport is much needed. The aims of this study were to 1) analyse whether unique profiles per sport could be established by generic characteristics and 2) to discuss similarities and differences for the potential application in talent development and transfer. By means of a validated survey, 1247 coaches from 34 sports ranked 18 characteristics on importance to their sports (0 = not important - 10 = very important). To discriminate the responses per sport a Discriminant Analysis (DA) was carried out. To refine the DA-classification, Uniform Manifold Approximation and Projection (UMAP) with CatBoost classifier was performed. To test the performance of the CatBoost classifier-algorithm, a confusion-matrix was generated. The cross-validated DA showed that 70.2% of the coaches were correctly classified to their sport. The UMAP/CatBoost technique revealed 75.1% accuracy with correctly predicted responses per sport ranging from 18.2% (sailing) to 98.2% (soccer). With varying precision, the algorithm was able to differentiate sports by importance of its characteristics indicating similarities and differences per sport.
更多查看译文
关键词
Talent transfer,talent orientation,talent development,youth sports,talent identification
AI 理解论文
溯源树
样例
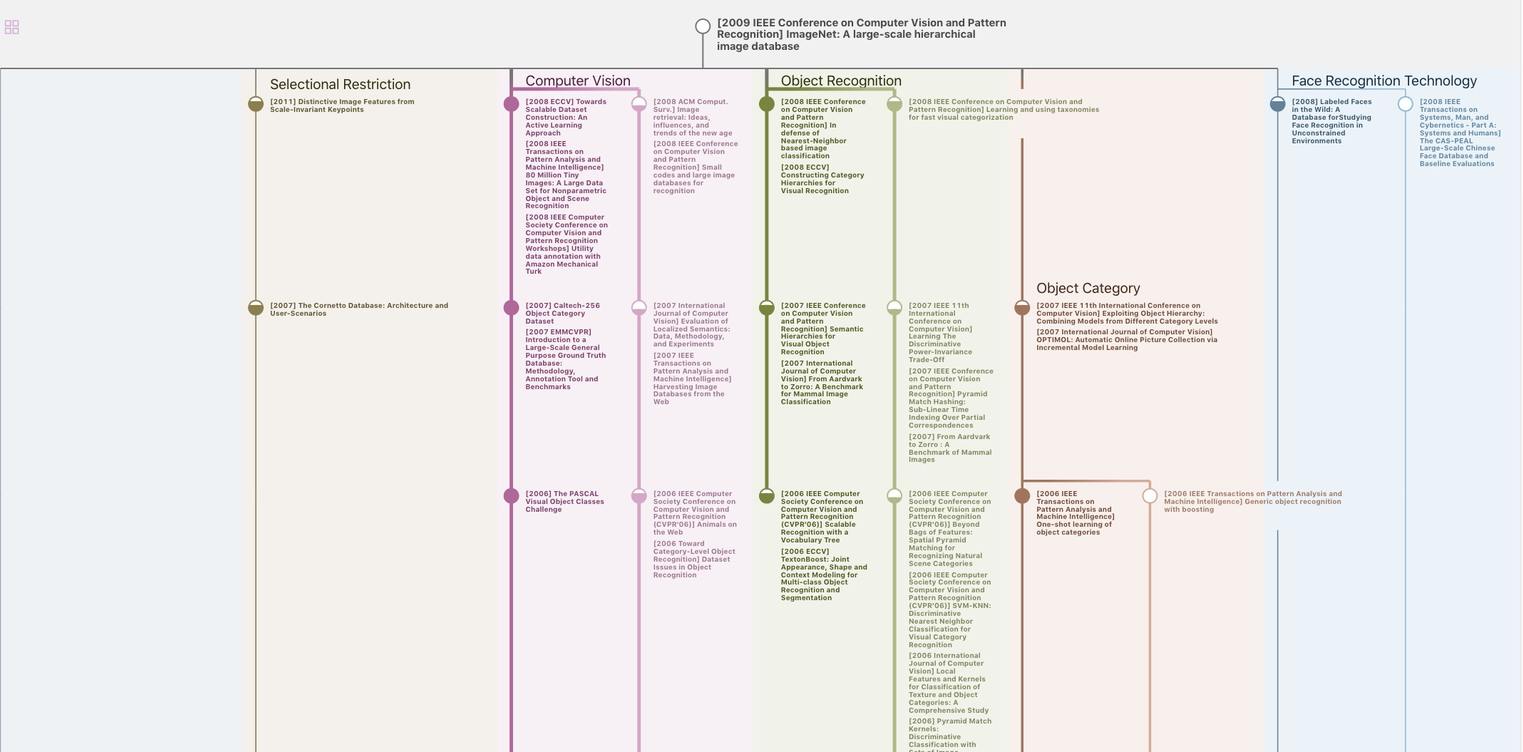
生成溯源树,研究论文发展脉络
Chat Paper
正在生成论文摘要