Causal explaining guided domain generalization for rotating machinery intelligent fault diagnosis
EXPERT SYSTEMS WITH APPLICATIONS(2024)
摘要
Post-hoc explaining approaches for deep learning (DL) models has attracted much attention in safety-critical applications such as rotating machinery intelligent fault diagnosis (IFD). However, with the help of the expla-nation techniques, the models are still fragile to domain shifts caused by varying speeds and loads without help for improving their cross-domain performance. Since humans in the decision-making loop are essential for a reliable diagnostic system to determine the reliability of diagnosis, this paper proposes a causal explaining guided domain generalization (CXDG) method to realize trust worthy IFD with human in the decision loop. Specifically, an explaining model is trained with the conditional mutual information, which is a causal strength metric, and utilized to tell the causal features in the input data as the attributions of the diagnostic model. A translation process of the attributions is proposed to make the explaining process understandable. Furthermore, the aim of this paper is not only explaining but also beyond that the diagnostic model is guided to focus on the causal features to improve its generalization ability in unseen domains. The effectiveness of the method is validated on two experiment datasets. The results show that the proposed method can both explain the attri-butions of the diagnosis model and be beneficial to the generalization ability.
更多查看译文
关键词
Explainable artificial intelligence,Domain generalization,Intelligent fault diagnosis,Causality
AI 理解论文
溯源树
样例
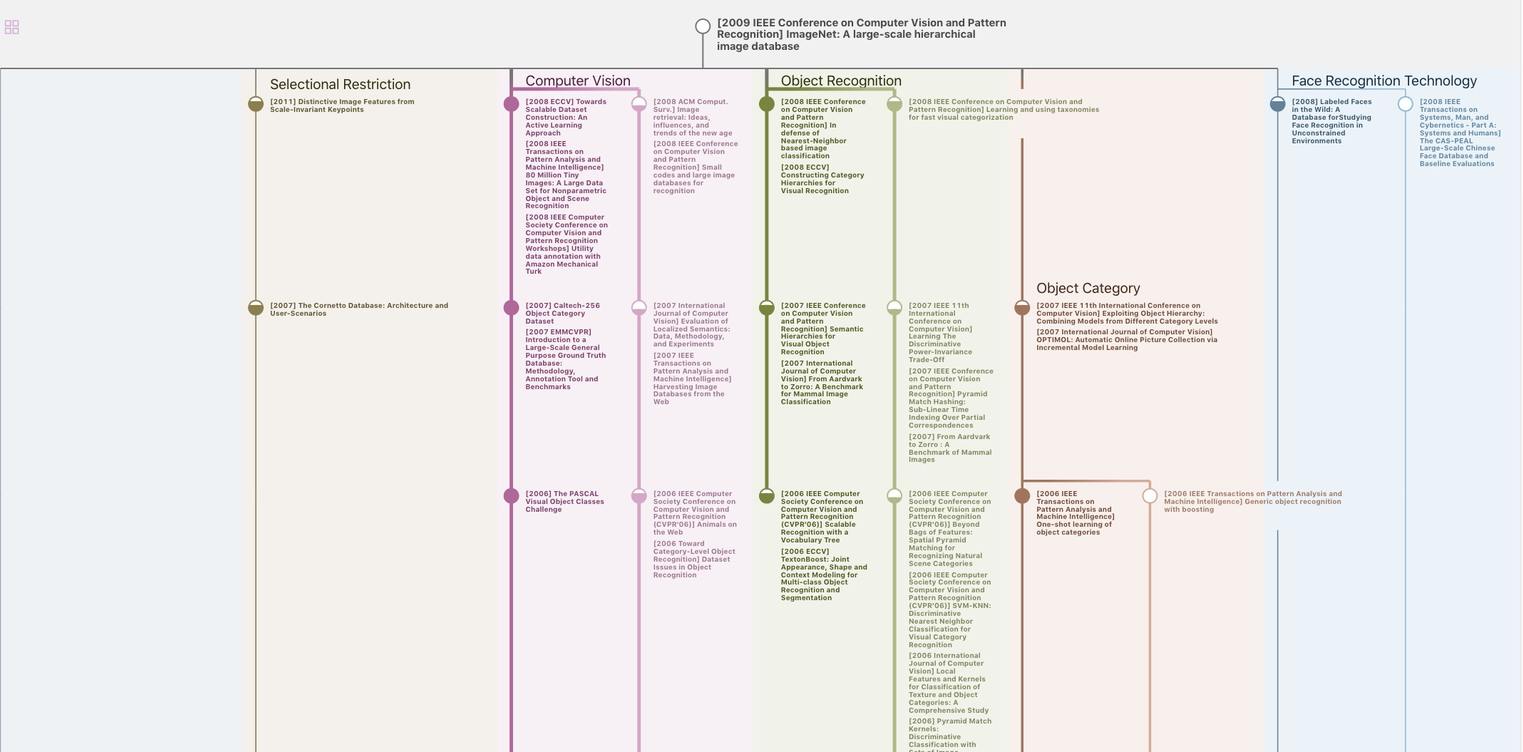
生成溯源树,研究论文发展脉络
Chat Paper
正在生成论文摘要