CNN Architecture Design Impact in DL-Based Channel Estimation Algorithms
2023 Workshop on Communication Networks and Power Systems (WCNPS)(2023)
摘要
This paper presents a trade-off study concerning the impact of convolutional neural network (CNN) architectures design on the performance of deep learning (DL) based channel estimation under orthogonal frequency-division multiplexing (OFDM) systems. Although CNNs have demonstrated remarkable performance in channel estimation, concerns arise regarding hardware implementation due to computation and power consumption limitations, particularly when dealing with limited hardware resources on the network edge. This study investigates the trade-off between the number of convolutional layers, the size and the number of convolutional filters, and the obtained CNN-based channel estimation performance, measured by the mean square error (MSE), error vector magnitude (EVM) and bit error rate (BER). The resource overhead is also analyzed considering the learnable parameters, memory usage estimation, and number of multiply-accumulate (MAC) operations.
更多查看译文
关键词
Channel estimation,CNN,DL,OFDM
AI 理解论文
溯源树
样例
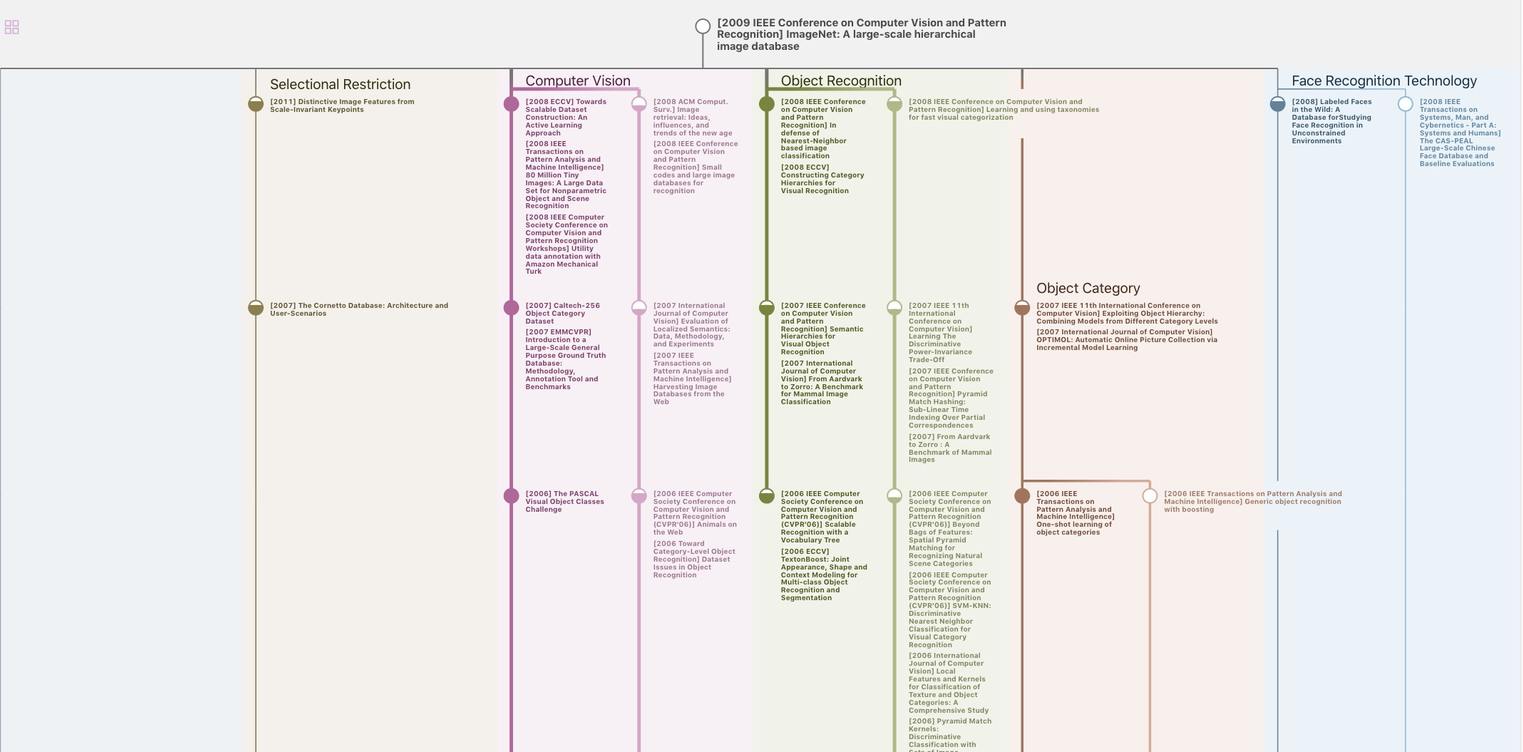
生成溯源树,研究论文发展脉络
Chat Paper
正在生成论文摘要