Task-Friendly Underwater Image Enhancement for Machine Vision Applications
IEEE TRANSACTIONS ON GEOSCIENCE AND REMOTE SENSING(2024)
摘要
Underwater images are often affected by color cast and blurring, which degrade the performance of underwater machine vision tasks. While existing underwater image enhancement (UIE) methods have been proposed to improve image quality for human perception, their effectiveness in enhancing machine vision performance is limited. In this article, a novel unsupervised UIE framework based on disentangled representation (DR) is proposed, which is designed for machine vision tasks. Specifically, the proposed framework disentangles the underwater image into two parts in the latent space according to whether they are beneficial to machine vision tasks: the task-friendly content features and the task-unfriendly distortion features. In addition, a semantic-aware contrastive module (SACM) is employed to alleviate the impact of losing key information required for machine vision tasks using the strategy of contrastive learning. Furthermore, two branches on the features and images are incorporated into the enhancement network, which serve the purpose of delivering task-relevant information to the enhancement model and guide the network to generate task-friendly images. Evaluation of the proposed method is conducted on multiple underwater image datasets, and a comparison is made with state-of-the-art enhancement methods in terms of machine vision performance. The experimental results demonstrate that the proposed method surpasses existing approaches in improving the accuracy and robustness of machine vision tasks, including object detection, semantic segmentation, and saliency detection in underwater environments. Our code is available at https://github.com/gemyumeng/TFUIE.
更多查看译文
关键词
Contrastive learning,disentangled representation (DR),machine vision tasks,underwater image enhancement (UIE)
AI 理解论文
溯源树
样例
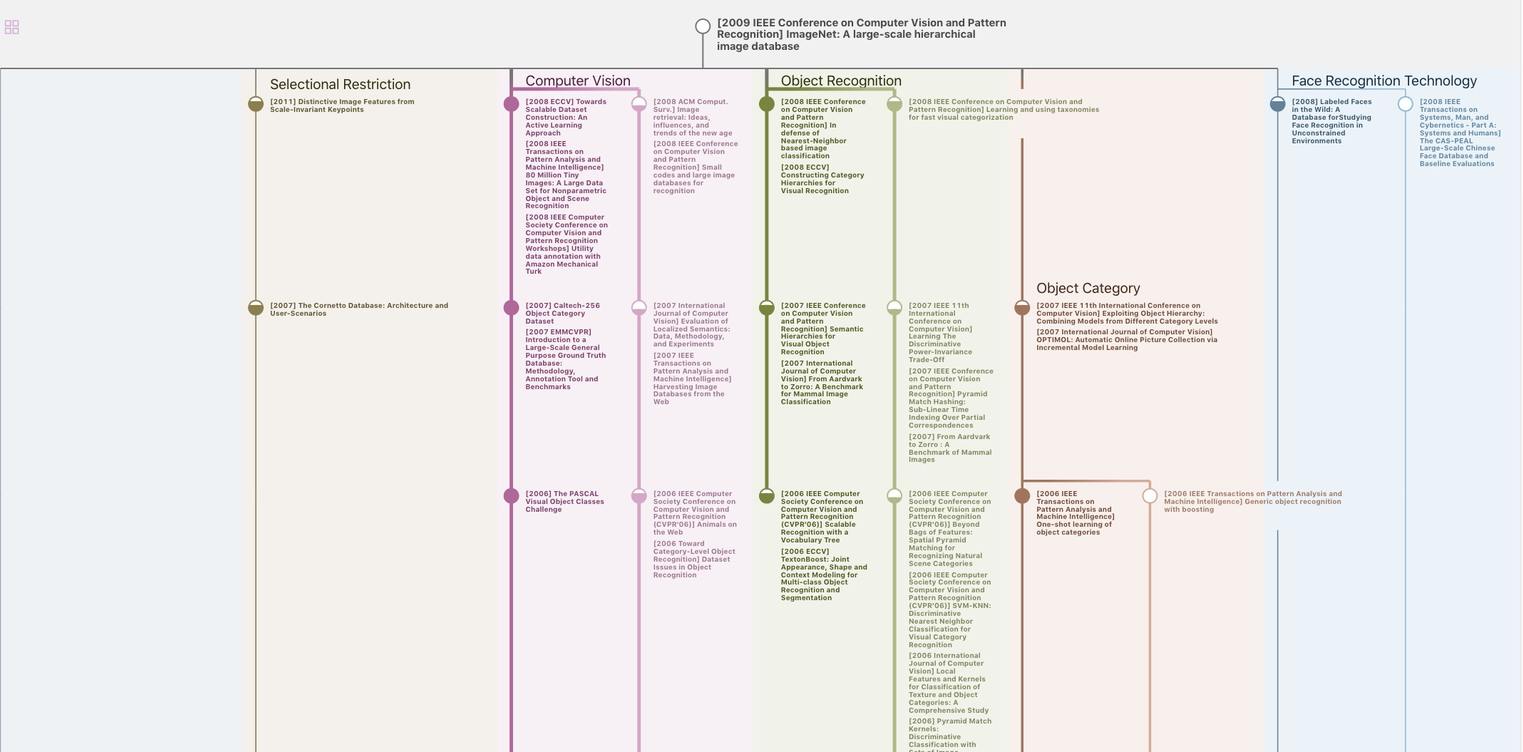
生成溯源树,研究论文发展脉络
Chat Paper
正在生成论文摘要