Robust and efficient algorithms for conversational contextual bandit
INFORMATION SCIENCES(2024)
摘要
Conversational contextual bandit is one of the notable variants of contextual bandit and it is shown to have superior performance in recommendation applications. The core idea of conversational contextual bandits utilizing is conversational feedback from users to improve the speed of learning user preference. We show that in real-world applications conversational feedback can be imbalanced and such feedback causes the latest conversational contextual bandit algorithm to conduct many conversations but has a slower learning speed than the baseline algorithm without conversational feedback. How to deal with imbalanced conversational feedback? How to schedule conversations across the learning horizon? In-depth analysis of the limitations of one representative conversational contextual bandit algorithm reveals insights to design ICF-UCB ((Imbalanced Conversational Feedback Upper Confidence Bound)) algorithm, which maintains a fast learning speed under imbalanced feedbacks. ICF-UCB achieves this by adaptively eliminating conversations that may slow down the learning speed. Furthermore, ICF-UCB adaptively schedules conversations to the decision rounds where suboptimal actions may trap the decision maker. It also adaptively selects appropriate conversations to avoid such traps. This algorithm is shown to have sublinear regret. Extensive experiments on synthetic datasets and public real-world datasets (from Yelp and TripAdvisor) validate the superior performance of ICF-UCB for recommendation tasks.
更多查看译文
关键词
Conversational contextual bandit,Imbalanced conversation feedback,Upper confidence bound,Regret analysis
AI 理解论文
溯源树
样例
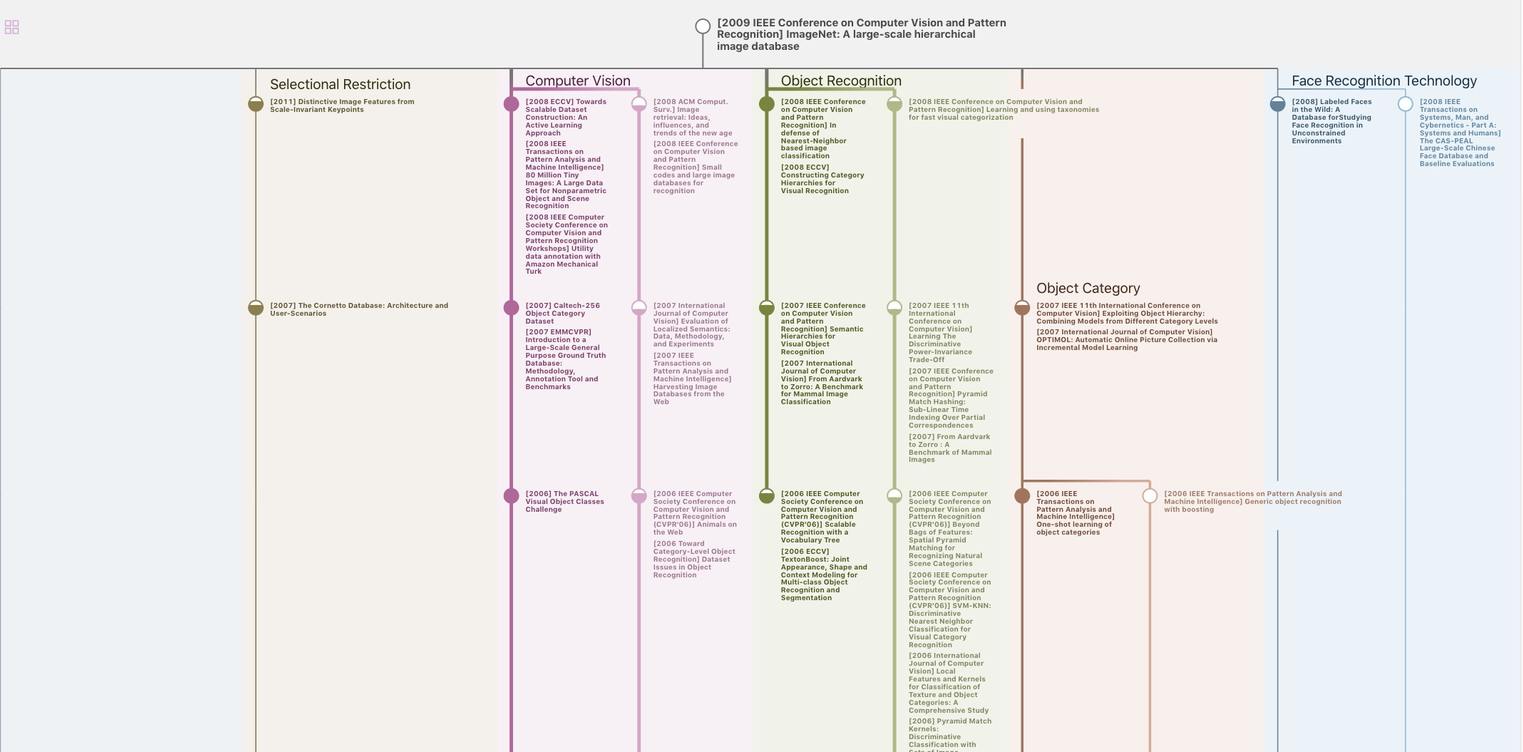
生成溯源树,研究论文发展脉络
Chat Paper
正在生成论文摘要