EM-LSD-Based Visual-Inertial Odometry With Point-Line Feature
IEEE SENSORS JOURNAL(2023)
摘要
Conventional point feature-based visual simultaneous localization and mapping (SLAM) is difficult to find reliable point feature to estimate camera pose in a structured low-texture environment. In contrast, line features are competent to work in such environment due to their advantage of expressing structural features. The line feature detection algorithm used in the current point-line fusion SLAM algorithm, such as line segment detection (LSD) algorithm suffer from massive, short-line feature, and long disconnected lines, which dramatically decrease the accuracy of pose estimation. Therefore, we propose a novel line feature detection method, named elimination, merging-line segment detection (EM-LSD), to obtain high-quality line features by the strategy of short-line rejection and approximate line segment merging. In addition, we tightly couple inertial measurement unit (IMU) and visual measurement at the back end. Furthermore, we optimize the state by minimizing the cost function that contains the information of IMU and point-line features. Finally, we perform experimental validation on the EuRoC and TUM VI dataset, and the experimental results show that our proposed EM-LSD algorithm can significantly improve the quality of extracted line features, and our proposed visual-inertial odometry algorithm can obtain higher localization accuracy than the state-of-the-art SLAM algorithm of the same type, PL-VINS.
更多查看译文
关键词
Feature extraction,Simultaneous localization and mapping,Visualization,Odometry,Cameras,Sensors,Optimization,Back-end optimization,line feature extraction,line segment detection (LSD),visual-inertial odometry
AI 理解论文
溯源树
样例
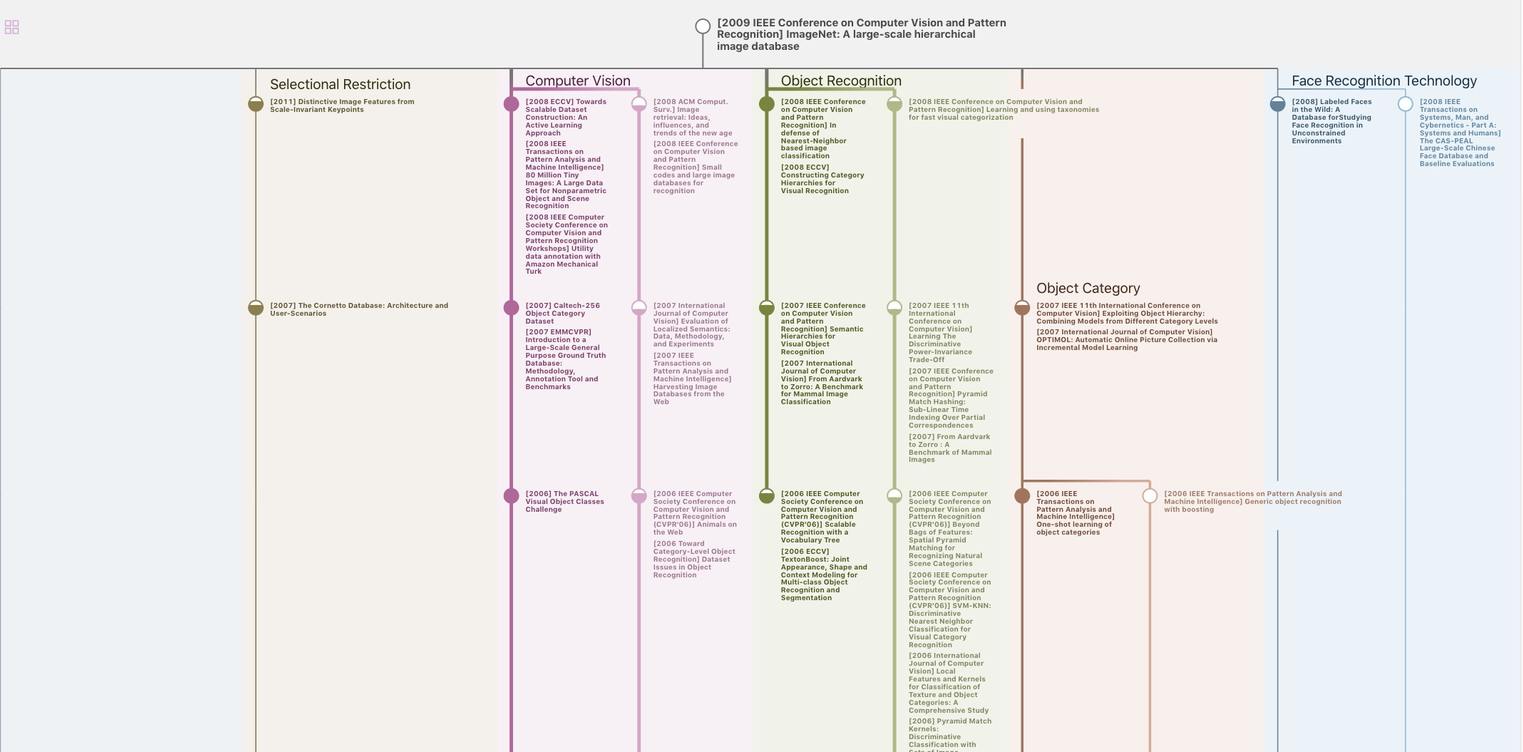
生成溯源树,研究论文发展脉络
Chat Paper
正在生成论文摘要