Attention Mechanisms in Process Mining: A Systematic Literature Review.
2023 XLIX Latin American Computer Conference (CLEI)(2023)
摘要
Process Mining (PM) focuses on monitoring and optimizing long-running business processes by examining their execution event logs (usually complex and heterogeneous) to obtain insights and enable data-driven decisions. Several Machine Learning (ML) techniques have been recently proposed to exploit these logs as learning datasets and enable examination of past events and predict future ones, but their black-box nature makes hard for human analysts to interpret their results and recognize the key parts of input data. Attention mechanisms (AM) is an ML technique that does address these shortcomings, but it has been little used for PM. This article describes the design, results and findings of a systematic literature review of attention mechanisms for PM. We addressed three research questions: (a) for which applications are AM used? (b) which kinds of AM are used? and (c) how are AM combined with other ML techniques? An initial search yield 73 papers, and inclusion/exclusion criteria left sixteen, published between 2017 and 2023. Key finding are that: (1) the most common application is sequence prediction, (2) most studies combine global and item-wise attention, added as layers after an encoder generates the continuous representation, and (3) emerging research topics include anomaly detection and data representation. This study shows that attention mechanisms can help process analysts to get some sense of interpretability, and showcases the bright potential for process mining of attention mechanisms, which paradoxically have received little attention themselves.
更多查看译文
关键词
Attention mechanisms,Deep Learning,Process Mining,Systematic Literature Review,Interpretability
AI 理解论文
溯源树
样例
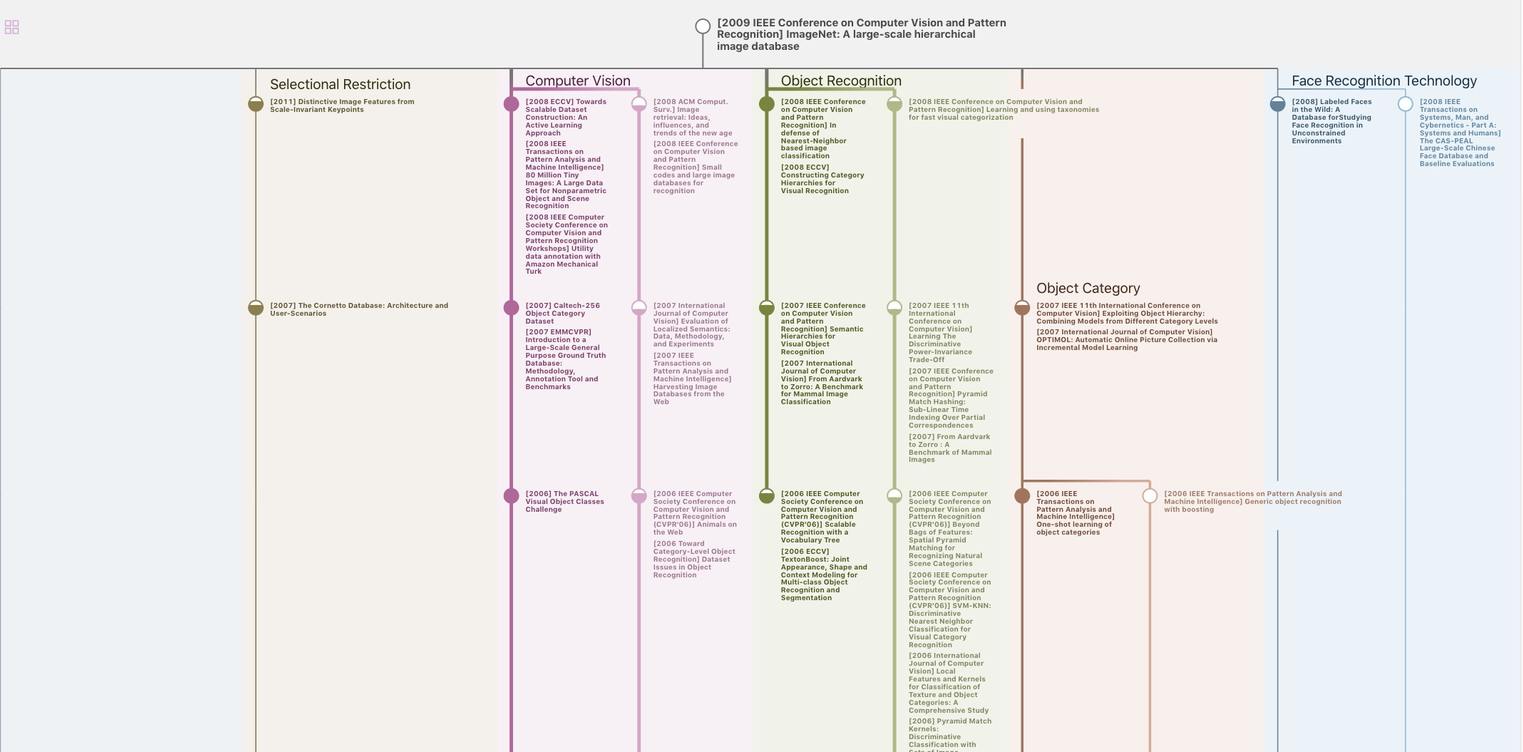
生成溯源树,研究论文发展脉络
Chat Paper
正在生成论文摘要