Uncertainty measurement for single cell RNA-seq data via Gaussian kernel: Application to unsupervised gene selection
Engineering Applications of Artificial Intelligence(2024)
摘要
A real-valued information system (RVIS) is an information system (IS) whose information values are real numbers. If the objects, attributes and information values of a RVIS change to cells, genes and gene expression values where gene expression data is single cell RNA-seq (scRNA) data, respectively, then this RVIS is referred to as a single cell gene space (scg-space). Unsupervised gene selection becomes very challenging due to a lack of decision information, which is to select the optimal gene subset that can maintain learning ability without decision information. However, little research has been done on unsupervised gene selection. Uncertainty measurement is a tool of gene selection. In view of this, this paper studies uncertainty measurement in an scg-space via Gaussian kernel and explores its application for unsupervised gene selection. In the first place, the distance between two cells in a given subspace is constructed. In the next place, the fuzzy Tcos-equivalence relation induced by this subspace is obtained employing Gaussian kernel. After that, measures of uncertainty for an scg-space are investigated. Lastly, gene selection algorithms in an scg-space are presented by using the proposed information entropy and information granularity. The presented algorithms are applied to clustering analyses of scRNA data. Multiple publicly available scRNA data sets are employed to evaluate the gene selection performances of the presented algorithms, while two commonly-used clustering methods, kmeans and AGNES, are utilized to obtain four metrics such as Silhouette Coefficient (SC), Davies-Bouldin Index (DBI), Fowlkes and Mallows Index (FMI), Normalized Mutual Information (NMI) . The clustering results demonstrated that the presented algorithms can lower significantly the number genes selected, achieve the better SC, DBI, FMI and NMI. They also show that the presented algorithms are superior to raw data and PCA and NMF regardless of using kmeans or AGNES clustering. This also indirectly demonstrates that the granulation measure and information entropy can effectively evaluate the uncertainty of an scg-space.
更多查看译文
关键词
Single cell RNA-seq data,scg-space,Gene selection,Gaussian kernel,Uncertainty measurement
AI 理解论文
溯源树
样例
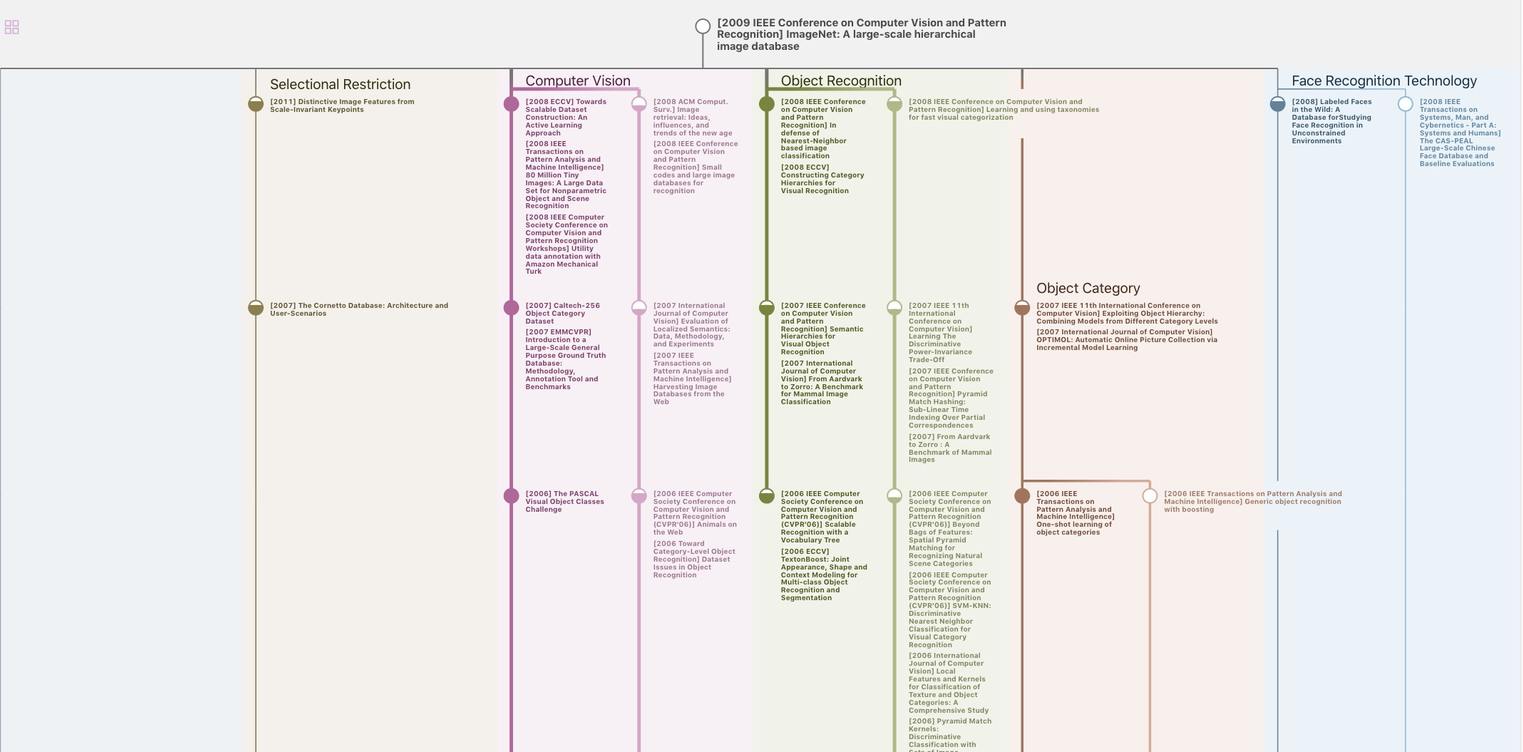
生成溯源树,研究论文发展脉络
Chat Paper
正在生成论文摘要