Validation set sampling strategies for predictive process monitoring
INFORMATION SYSTEMS(2024)
摘要
Previous studies investigating the efficacy of long short-term memory (LSTM) recurrent neural networks in predictive process monitoring and their ability to capture the underlying process structure have raised concerns about their limited ability to generalize to unseen behavior. Event logs often fail to capture the full spectrum of behavior permitted by the underlying processes. To overcome these challenges, this study introduces innovative validation set sampling strategies based on control-flow variant-based resampling. These strategies have undergone extensive evaluation to assess their impact on hyperparameter selection and early stopping, resulting in notable enhancements to the generalization capabilities of trained LSTM models. In addition, this study expands the experimental framework to enable accurate interpretation of underlying process models and provide valuable insights. By conducting experiments with event logs representing process models of varying complexities, this research elucidates the effectiveness of the proposed validation strategies. Furthermore, the extended framework facilitates investigations into the influence of event log completeness on the learning quality of predictive process models. The novel validation set sampling strategies proposed in this study facilitate the development of more effective and reliable predictive process models, ultimately bolstering generalization capabilities and improving the understanding of underlying process dynamics.
更多查看译文
关键词
Process mining,Predictive process monitoring,LSTM,Generalization,Validation set,Log completeness
AI 理解论文
溯源树
样例
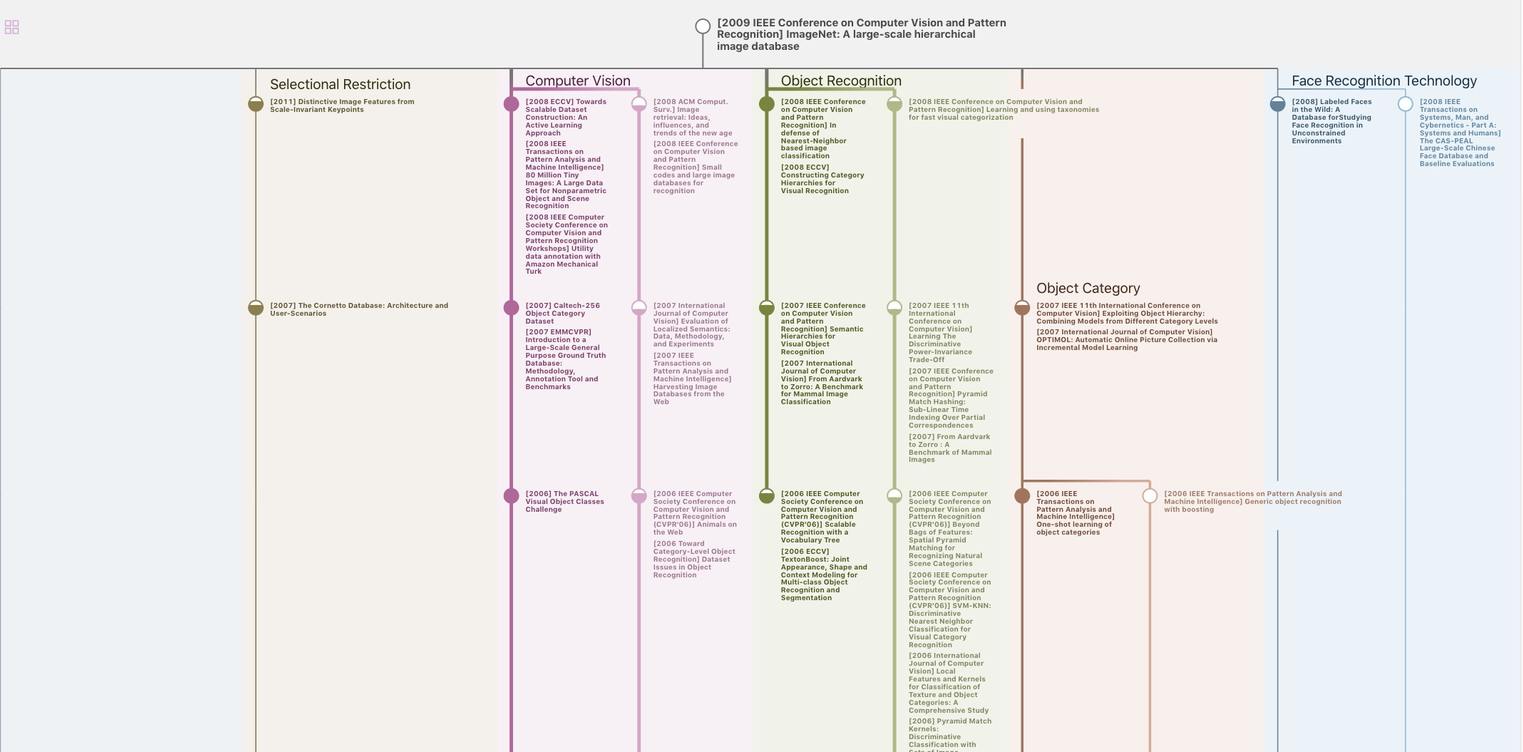
生成溯源树,研究论文发展脉络
Chat Paper
正在生成论文摘要