Condition Invariance for Autonomous Driving by Adversarial Learning
PROGRESS IN PATTERN RECOGNITION, IMAGE ANALYSIS, COMPUTER VISION, AND APPLICATIONS, CIARP 2023, PT I(2024)
摘要
Object detection is a crucial task in autonomous driving, where domain shift between the training and the test set is one of the main reasons behind the poor performance of a detector when deployed. Some erroneous priors may be learned from the training set, therefore a model must be invariant to conditions that might promote such priors. To tackle this problem, we propose an adversarial learning framework consisting of an encoder, an object-detector, and a condition-classifier. The encoder is trained to deceive the condition-classifier and aid the object-detector as much as possible throughout the learning stage, in order to obtain highly discriminative features. Experiments showed that this framework is not very competitive regarding the trade-off between precision and recall, but it does improve the ability of the model to detect smaller objects and some object classes.
更多查看译文
关键词
Adversarial learning,Autonomous driving,Computer vision,Deep learning,Domain generalization
AI 理解论文
溯源树
样例
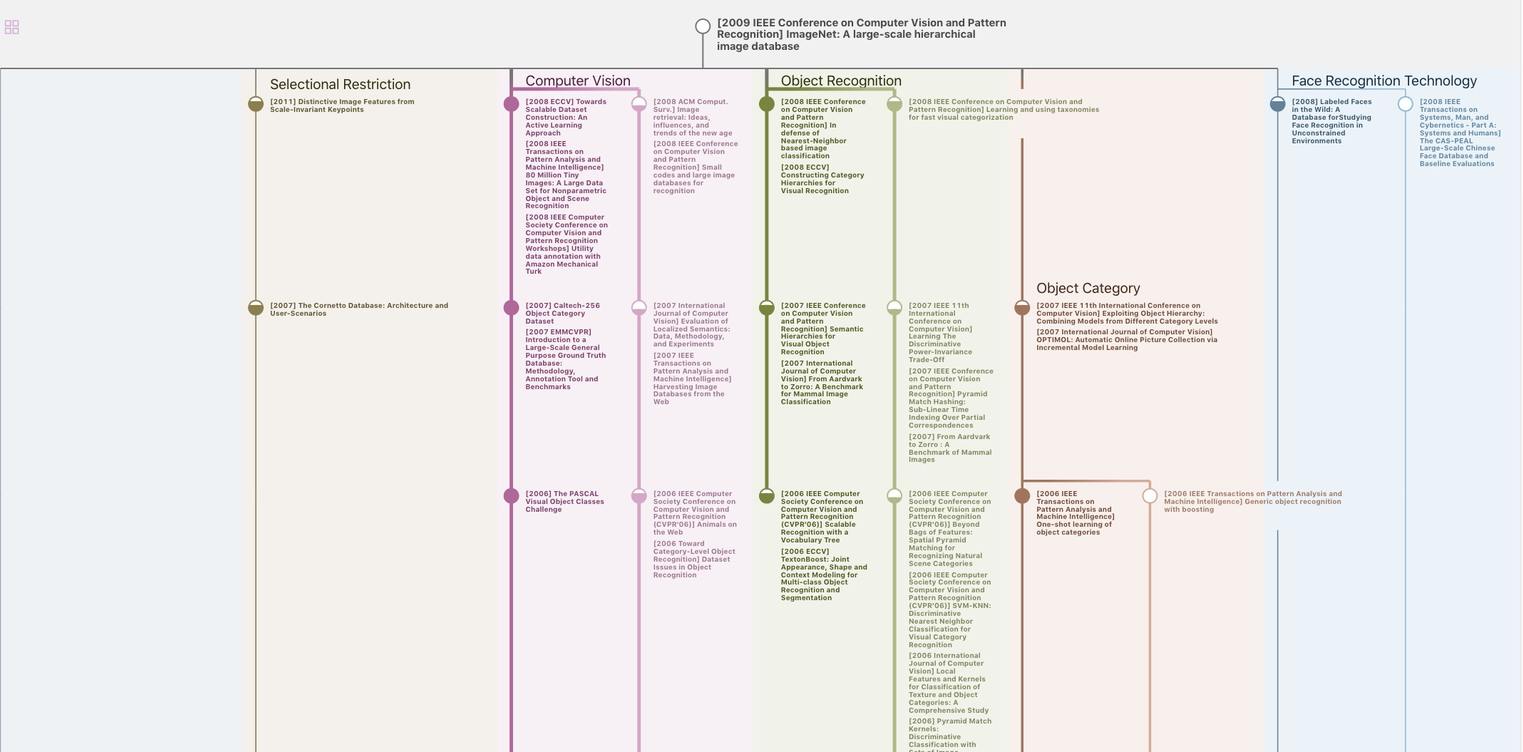
生成溯源树,研究论文发展脉络
Chat Paper
正在生成论文摘要