Streaming Graph-Based Supervoxel Computation Based on Dynamic Iterative Spanning Forest
PROGRESS IN PATTERN RECOGNITION, IMAGE ANALYSIS, COMPUTER VISION, AND APPLICATIONS, CIARP 2023, PT II(2024)
摘要
Streaming video segmentation decreases processing time by creating supervoxels taking into account small parts of the video instead of using all video content. Thanks to the good performance of the Iterative Spanning Forest to compute Supervoxels (ISF2SVX) based on Dynamic Iterative Spanning Forest (DISF) for video segmentation framework we propose a new graph-based streaming video segmentation method for supervoxel generation by using dynamic iterative spanning forest framework, so-called StreamISF, based on a pipeline composed of six stages: (1) formation of the graph for each block of the video; (2) seed oversampling; (3) IFT-based supervoxel design; (4) reduction in the number of supervoxels; (5) spread of trees; and (6) creation of the segmented video. The difference in our proposed method is that it is unnecessary to have all the video in memory and the only previous information necessary to segment a block is the intersection frame between the blocks. Moreover, experimental results show that StreamISF creates supervoxels that maintain temporal coherence, producing very competitive measures compared to the state-of-the-art. Our code is publically available at https://github.com/IMScience-PPGINF-PucMinas/StreamISF.
更多查看译文
关键词
Graph-based method,Streaming,Supervoxel,Dynamic Iterative Spanning Forest
AI 理解论文
溯源树
样例
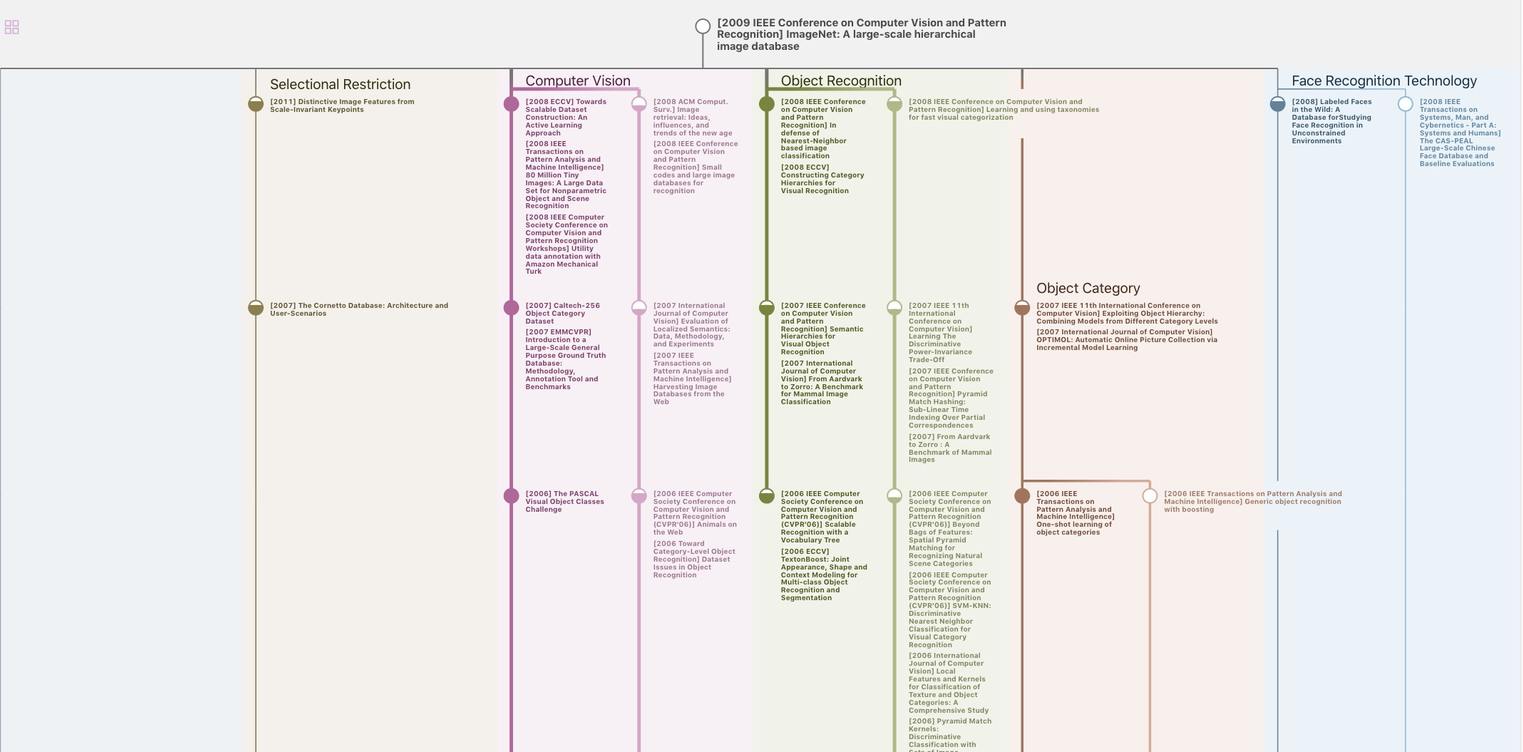
生成溯源树,研究论文发展脉络
Chat Paper
正在生成论文摘要