Cross-Modality Deep Transfer Learning: Application to Liver Segmentation in CT and MRI
MEDICAL IMAGE UNDERSTANDING AND ANALYSIS, MIUA 2023(2024)
摘要
Liver diseases cause up to two million deaths yearly. Their diagnosis and treatment plans require an accurate assessment of the liver structure and tissue characteristics. Imaging modalities such as computed tomography (CT) and Magnetic resonance (MR) can be used to assess the liver. CT has better spatial resolution compared to MR, which has better tissue contrast. Each modality has its own applications. However, CT is widely used due its ease of access, lower cost and a shorter examination time. Liver segmentation is an important step that helps to accurately identify and isolate the liver from other organs and tissues in medical images. This can be useful for diagnosing and treating liver diseases. Manual liver segmentation is costly and time-intensive. Machine learning and deep learning algorithms can be used to automate this process. However, deep learning methods require a large amount of training data, which is not available for MR. There are many CT datasets available compared to few MR datasets. The use of transfer learning can help to mitigate the problem of having a small amount of training data. We suggest training a U-Net deep learning model on the large publicly available CT dataset liver tumor segmentation challenge (LiTS), then use transfer learning to fine-tune the model to the smaller available MR datasets Duke liver dataset (DLDS) and Combined (CTMR) Healthy Abdominal Organ Segmentation (CHAOS). This allows the model to leverage the knowledge it has gained from the larger CT dataset to improve its performance on the smaller MR datasets. The model reached a dice of 0.83 on unseen DLDS data after fine-tuning to the small CHAOS dataset. It also improved the testing dice on CHAOS itself when compared to training the model from scratch on CHAOS only from 0.59 to 0.86. A universal model trained on the combined datasets achieved a testing dice of 0.928 on LiTS, 0.865 on DLDS, and 0.815 on CHAOS.
更多查看译文
关键词
U-Net,Transfer Learning.,Across modalities,Liver Segmentation
AI 理解论文
溯源树
样例
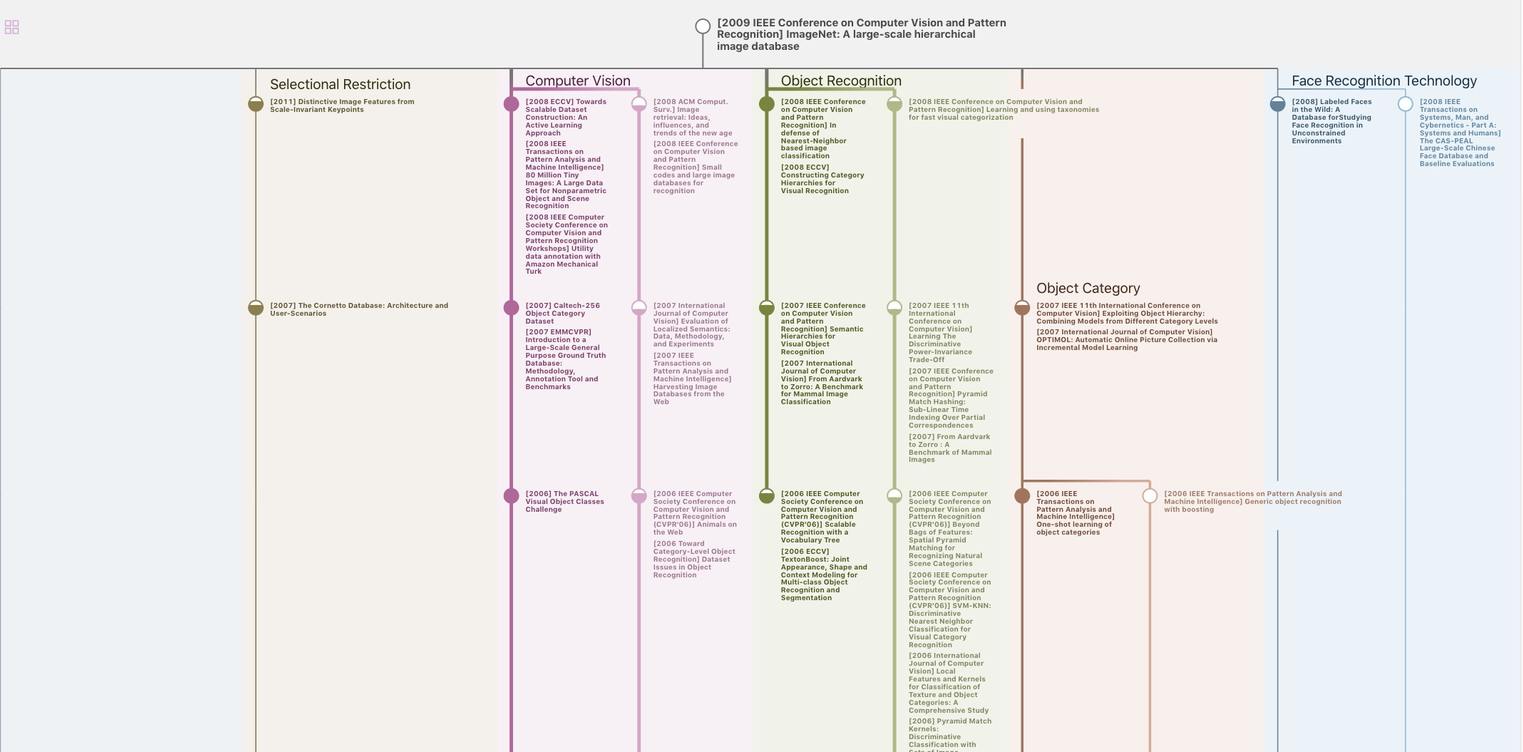
生成溯源树,研究论文发展脉络
Chat Paper
正在生成论文摘要