LitNeRF: Intrinsic Radiance Decomposition for High-Quality View Synthesis and Relighting of Faces.
ACM SIGGRAPH Conference and Exhibition on Computer Graphics and Interactive Techniques in Asia(2023)
摘要
High-fidelity, photorealistic 3D capture of a human face is a long-standing problem in computer graphics – the complex material of skin, intricate geometry of hair, and fine scale textural details make it challenging. Traditional techniques rely on very large and expensive capture rigs to reconstruct explicit mesh geometry and appearance maps, and are limited by the accuracy of hand-crafted reflectance models. More recent volumetric methods (e.g., NeRFs) have enabled view-synthesis and sometimes relighting by learning an implicit representation of the density and reflectance basis, but suffer from artifacts and blurriness due to the inherent ambiguities in volumetric modeling. These problems are further exacerbated when capturing with few cameras and light sources. We present a novel technique for high-quality capture of a human face for 3D view synthesis and relighting using a sparse, compact capture rig consisting of 15 cameras and 15 lights. Our method combines a neural volumetric representation with traditional mesh reconstruction from multiview stereo. The proxy geometry allows us to anchor the 3D density field to prevent artifacts and guide the disentanglement of intrinsic radiance components of the face appearance such as diffuse and specular reflectance, and incident radiance (shadowing) fields. Our hybrid representation significantly improves the state-of-the-art quality for arbitrarily dense renders of a face from desired camera viewpoint as well as environmental, directional, and near-field lighting.
更多查看译文
AI 理解论文
溯源树
样例
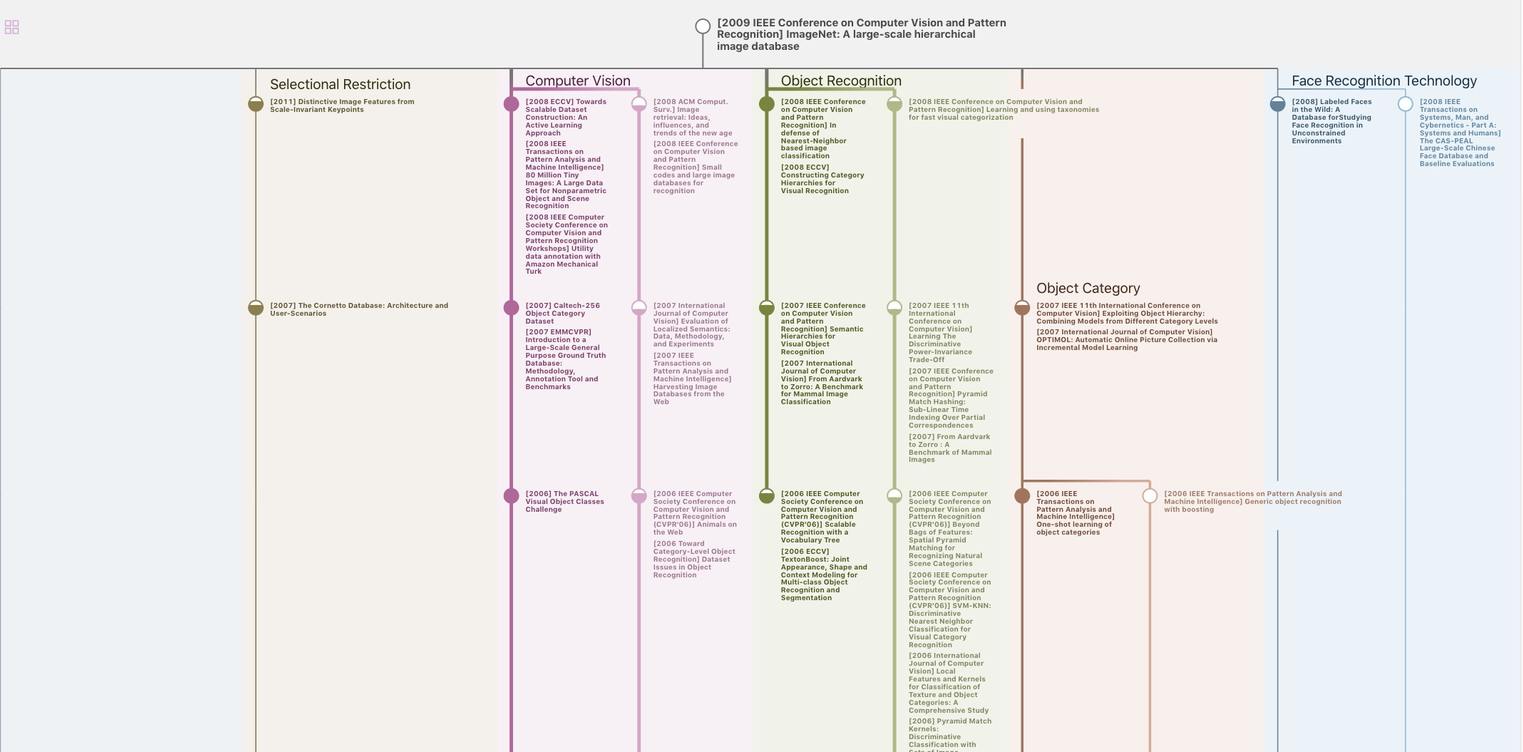
生成溯源树,研究论文发展脉络
Chat Paper
正在生成论文摘要