Agricultural weed identification in images and videos by integrating optimized deep learning architecture on an edge computing technology
COMPUTERS AND ELECTRONICS IN AGRICULTURE(2024)
摘要
Recent advancements in deep learning (DL)-based model optimization techniques have resulted in better weed identification accuracy. However, optimizing these models to identify weeds in images captured using small unmanned aerial system (UAS) has not been much explored. Moreover, leveraging the optimized model on resource constrained edge platform that could be easily integrated with UAS for real-time weed identification could be of significant advantage in developing precision aerial spraying weed management technology. Therefore, this study proposes YOLO-Spot model that is based on YOLOv7-tiny architecture, has been optimized and reconstructed to identify weeds amongst crop plants in aerial images and videos. The optimized model tends to use a smaller number of trainable parameters and reduced feature map sizes for weed identification. Most of the redundant convolutional layers along with feature map sizes have been reduced coupled with an integration of a novel module re-parameterized convolutional layer (RCL) within the neck component of the network. Furthermore, YOLO-Spot model has been trained on the three image resolutions, 320 x 320, 640 x 640 and 1280 x 1280, and has been named as YOLO-Spot_S, YOLO-Spot_M and YOLO-Spot_L, respectively. Out of all the variants, YOLO-Spot_M model has achieved significant prediction accuracy as compared to other variants and a denser layered model YOLOv7-Base. YOLO-Spot_M model utilizes over 75 % less parameters and 86 % reduced GFLOPs compared to YOLOv7-Base. As per the results, YOLO-Spot_M has outperformed YOLOv7-Base by achieving +1.3 % and +2.7 % overall accuracy and mAP(@0.5), respectively. The optimized architecture utilizes 4X less power (in W) when trained on a normal graphical processing unit (GPU). Moreover, converting YOLOSpot_M to half-precision (FP16) for resource constrained device deployment (AGX Xavier), led to a +0.6 % accuracy and 5X faster weed recognition accuracy in aerial images and videos during inferencing. Based on the metrics obtained, YOLO-Spot_M model is recommended model that could be integrated with remote sensing technologies for site-specific weed management.
更多查看译文
关键词
Deep learning,Edge computing,Model optimization,Weed detection,Unmanned aerial system (UAS)
AI 理解论文
溯源树
样例
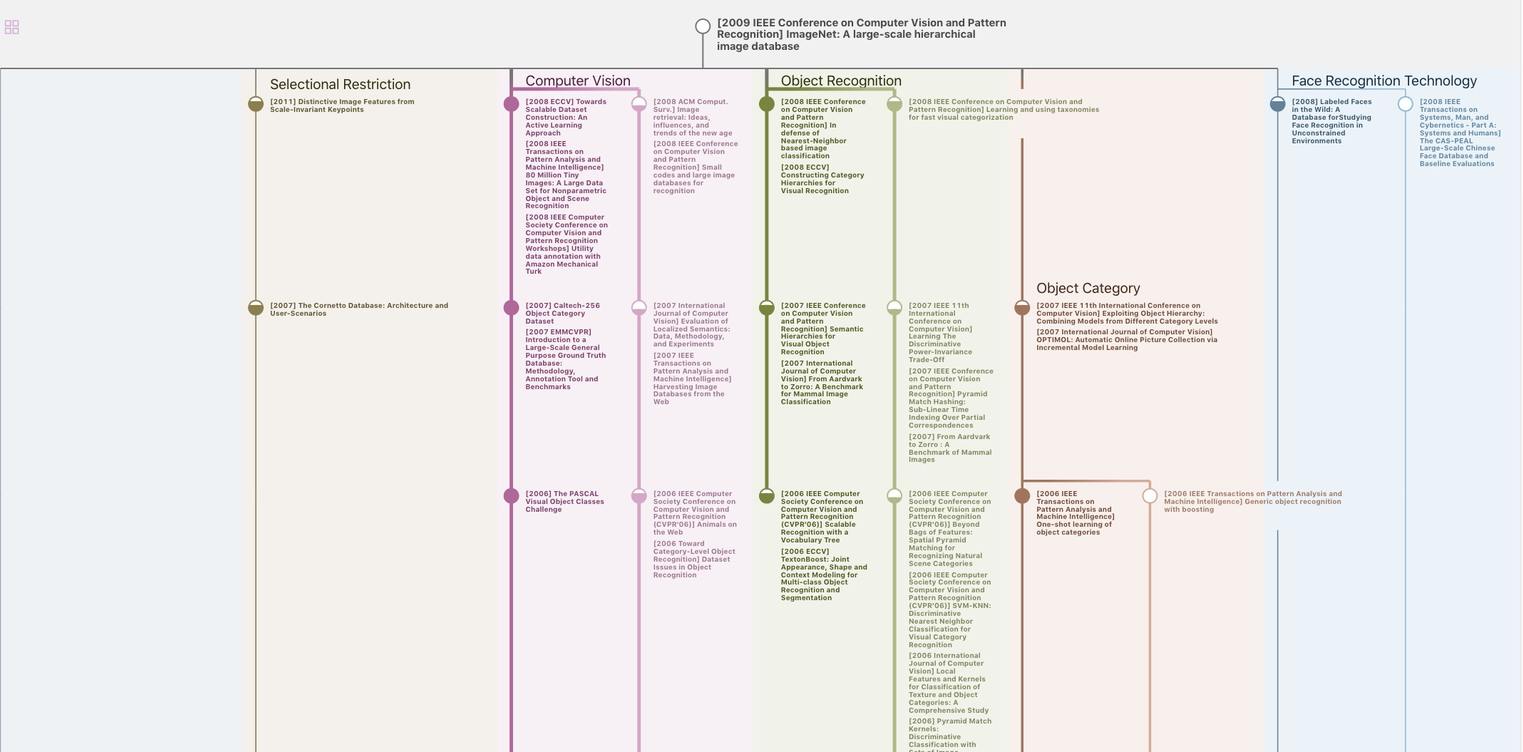
生成溯源树,研究论文发展脉络
Chat Paper
正在生成论文摘要