A hybrid deep learning modeling based on lumped parameter model of coal-fired circulating fluidized beds for real-time prediction
FUEL(2024)
摘要
The integration of large-scale renewable energy sources into the electrical grid has presented challenges that dynamic characteristics and mechanisms of thermal power plants are incapable of accomplishing, particularly circulating fluidized beds. Traditional mechanism models make it difficult to obtain precise parameter moni-toring because of the complicated mechanism process. Accurate parameter monitoring in the circulating fluid-ized bed unit, assisted by the advent of artificial intelligence, significantly increases the unit's operating stability. The combination of artificial intelligence technology and mechanism model research provides a new solution to improve the accuracy of parameter monitoring. The bed temperature parameter of circulating fluidized bed units is taken as one of the most predominant characteristics of CFB operational stability. By analyzing and simplifying the bed temperature lumped mechanism model, this research identified the expression form of the feedforward neural network model and the calculation form of the convolutional neural network model and proposed a deep learning model based on the bed temperature lumped parameter model. The superior effectiveness and precision of the model are verified by the operation data of two actual units in this paper. The prediction model in this chapter achieved mean absolute error (MAE) and mean absolute percentage error (MAPE) values of 2.2579 degrees C, 1.5453 degrees C, 0.2417%, and 0.1736%, respectively. Finally, the model has also been successfully applied in the prediction task of in-situ sulfur dioxide of circulating fluidized bed units.
更多查看译文
关键词
CFB,Bed temperature,Deep learning,Hybrid modeling method
AI 理解论文
溯源树
样例
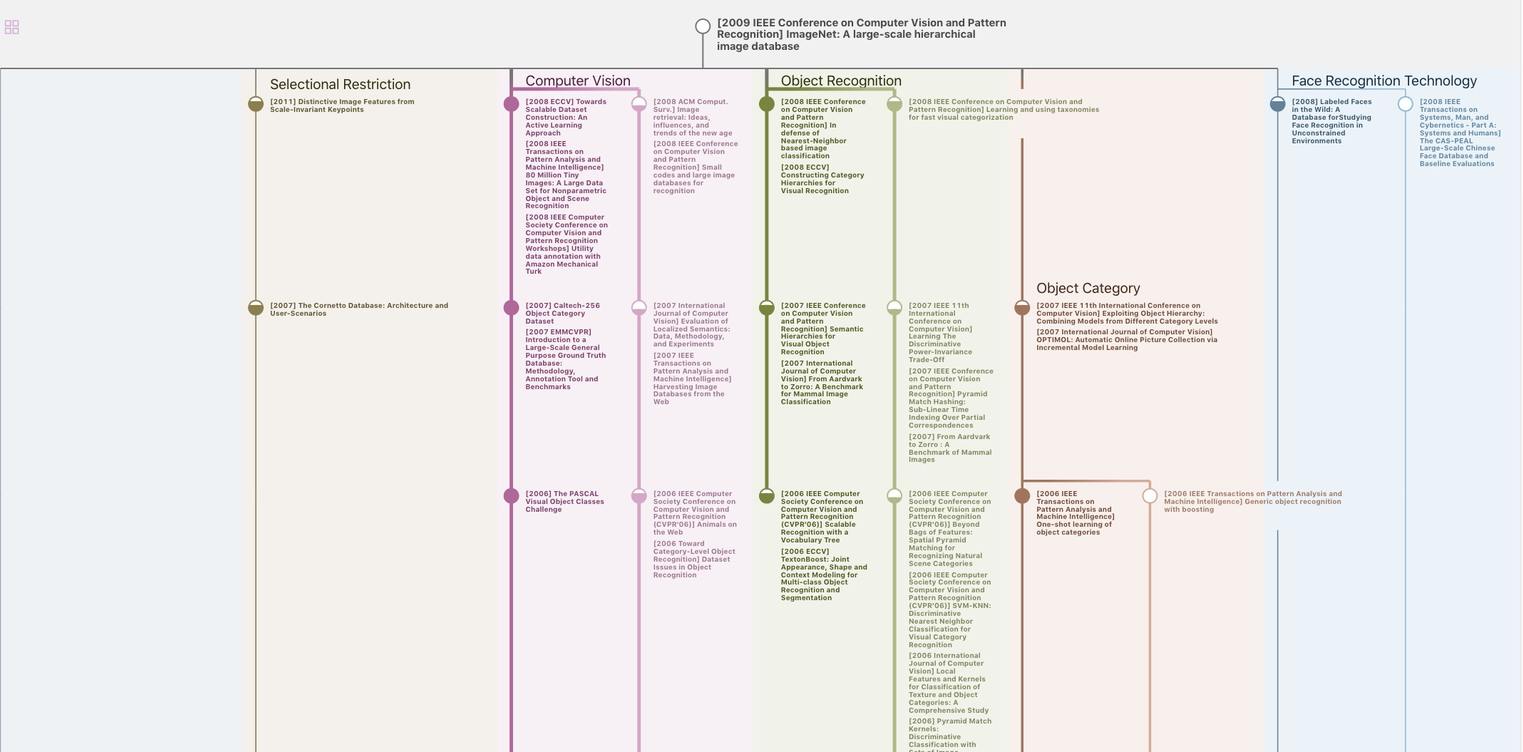
生成溯源树,研究论文发展脉络
Chat Paper
正在生成论文摘要