High-throughput computational screening and molecular fingerprint design of metal-organic framework adsorbents for separation of C3 components
GIANT(2024)
摘要
To decrease the consumption of energy and material resources caused by the traditional "two-step" process for separating propylene from the C3 crude components, including propane, propylene, methylacetylene and propadiene, this work utilizes the metal-organic frameworks (MOFs) involved "one-step" process to purify propylene. First, the relationship between the geometric/energy descriptors of MOFs and their performance metrics was established through univariate analysis, and results show that the top-performance MOFs can be screened by the differences of LCD, rho, VSA and phi. Then, different combinations of descriptors and algorithms were used for machine learning. The combination (RF algorithm, 7 basic descriptors + PSD + MorganFPs + nodes + topologies) with the best prediction accuracy ( R = 0.87) for predicting the performance of MOFs was found. Finally, 4 optimal pore structures for the design of high propylene adsorption performance materials were summarized, which mainly contain cylindrical channels and spherical cavities similar in size to the target gas. The microscopic control of pore structures obtained from our bottom-up approach is useful for the development of MOFs and other nanoporous materials which can be used for "one-step" separation of propylene from C3 mixtures in various industrial situations.
更多查看译文
关键词
High-throughput computational screening,Propylene adsorption,Metal-organic frameworks,Molecular fingerprint,Machine learning
AI 理解论文
溯源树
样例
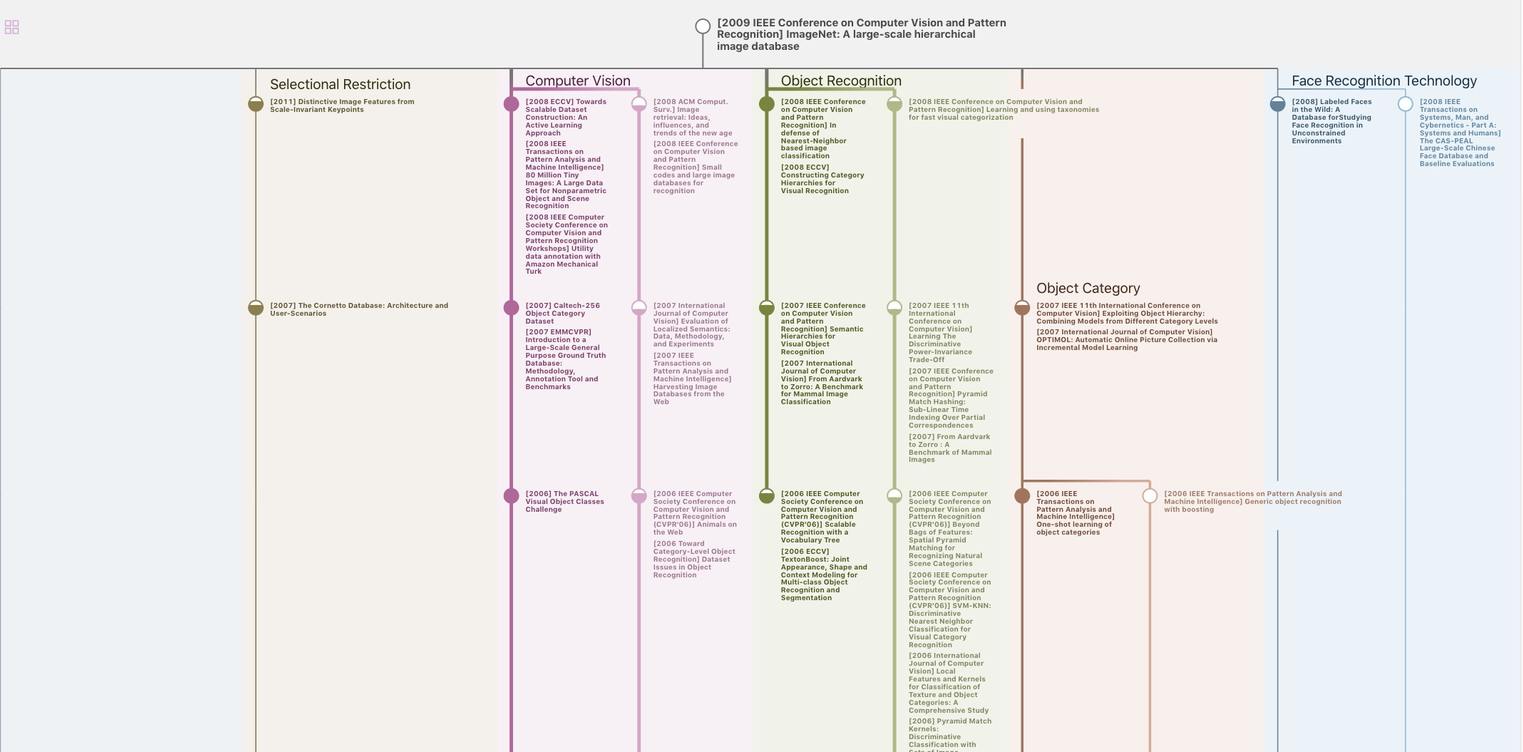
生成溯源树,研究论文发展脉络
Chat Paper
正在生成论文摘要