AE-Qdrop: Towards Accurate and Efficient Low-bit Post-training Quantization for Convolutional Neural Network
crossref(2023)
摘要
Post-training quantization is pivotal in deploying convolutional neural networks for mobile applications. Block-wise reconstruction with adaptive rounding, as employed in prior works like BrecQ and Qdrop, facilitates acceptable 4-bit quantization accuracy. However, adaptive rounding is time-intensive, and its constraint on weight optimization space curtails the potential for quantization performance. The optimality of block-wise reconstruction hinges on the quantization status of subsequent network blocks. In this investigation, we delve into the theoretical underpinnings of the limitations inherent in adaptive rounding and block-wise reconstruction. Our exploration leads to the development of a post-training quantization methodology, designated as AE-Qdrop. This algorithm operates in two distinct phases: block-wise reconstruction and global fine-tuning. The block-wise reconstruction phase introduces a progressive optimization strategy, superseding adaptive rounding, which not only augments quantization precision but also significantly improves quantization efficiency. To mitigate the risk of overfitting, we introduce a random weighted quantized activation mechanism. During the global fine-tuning phase, we account for interdependencies among quantized network blocks. The weight of each network block will be corrected with logit matching and feature matching. Extensive experiments validate that AE-Qdrop achieves high-precision and efficient quantization. For instance, in the case of 2-bit MobileNetV2, AE-Qdrop outperforms Qdrop by achieving a 6.26% enhancement in quantization accuracy and quintupling the quantization efficiency.
更多查看译文
AI 理解论文
溯源树
样例
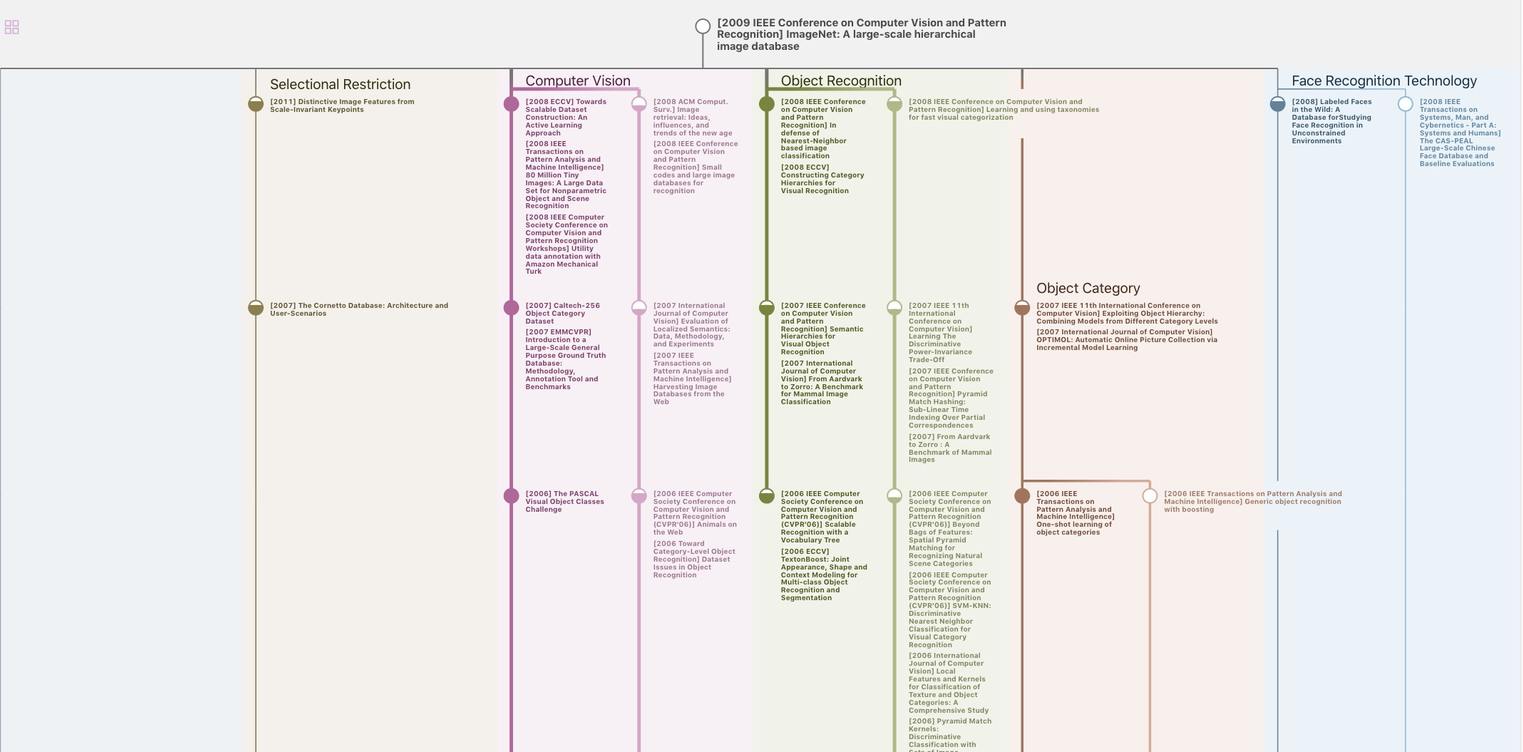
生成溯源树,研究论文发展脉络
Chat Paper
正在生成论文摘要