Heterogeneous Graph Neural Architecture Search with GPT-4
CoRR(2023)
摘要
Heterogeneous graph neural architecture search (HGNAS) represents a powerful
tool for automatically designing effective heterogeneous graph neural networks.
However, existing HGNAS algorithms suffer from inefficient searches and
unstable results. In this paper, we present a new GPT-4 based HGNAS model to
improve the search efficiency and search accuracy of HGNAS. Specifically, we
present a new GPT-4 enhanced Heterogeneous Graph Neural Architecture Search
(GHGNAS for short). The basic idea of GHGNAS is to design a set of prompts that
can guide GPT-4 toward the task of generating new heterogeneous graph neural
architectures. By iteratively asking GPT-4 with the prompts, GHGNAS continually
validates the accuracy of the generated HGNNs and uses the feedback to further
optimize the prompts. Experimental results show that GHGNAS can design new
HGNNs by leveraging the powerful generalization capability of GPT-4. Moreover,
GHGNAS runs more effectively and stably than previous HGNAS models based on
reinforcement learning and differentiable search algorithms.
更多查看译文
AI 理解论文
溯源树
样例
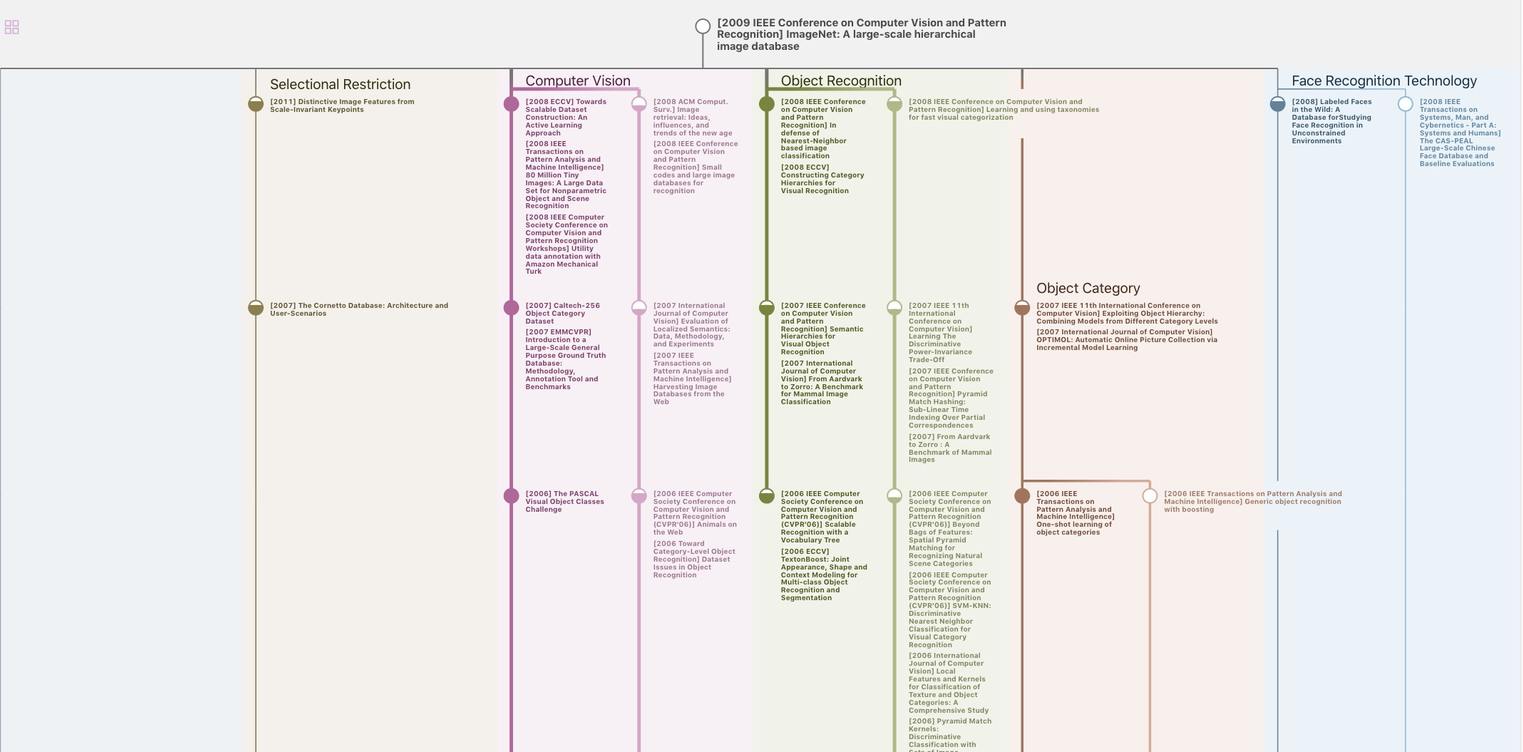
生成溯源树,研究论文发展脉络
Chat Paper
正在生成论文摘要