Cross-domain few-shot learning based on feature adaptive distillation
Neural Computing and Applications(2024)
摘要
Recently, few-shot learning (FSL) has exhibited remarkable performance in computer vision tasks. However, the existing FSL approaches perform poorly when facing data shortages and domain variations between the source and target datasets. This is because the target domain is hidden during training and the strong discriminating ability on the source domain dataset cannot be properly transferred into the good classification precision on the target dataset. To address the optimization problem for cross-domain few-shot image identification, this study proposed the Feature Adaptive Distillation (FAD) method. Specifically, we capture broader variations in feature distributions through a novel Feature Adaptive Distillation method. The two primary components of FAD are the Self-Distillation module (SD) and the Feature Adaptive module (FA). By including additional adaptive parameters for particular tasks in the feature extractor, FA enhances the generalization performance of this method. To enhance it’s ability to recognize features and determine the most effective feature extractor, the feature extractor is further self-distilled using SD. The results indicate that this method can greatly enhance the effectiveness of such kind image recognition.
更多查看译文
关键词
Cross-domain,Few-shot learning,Feature adaptive,Self-distillation
AI 理解论文
溯源树
样例
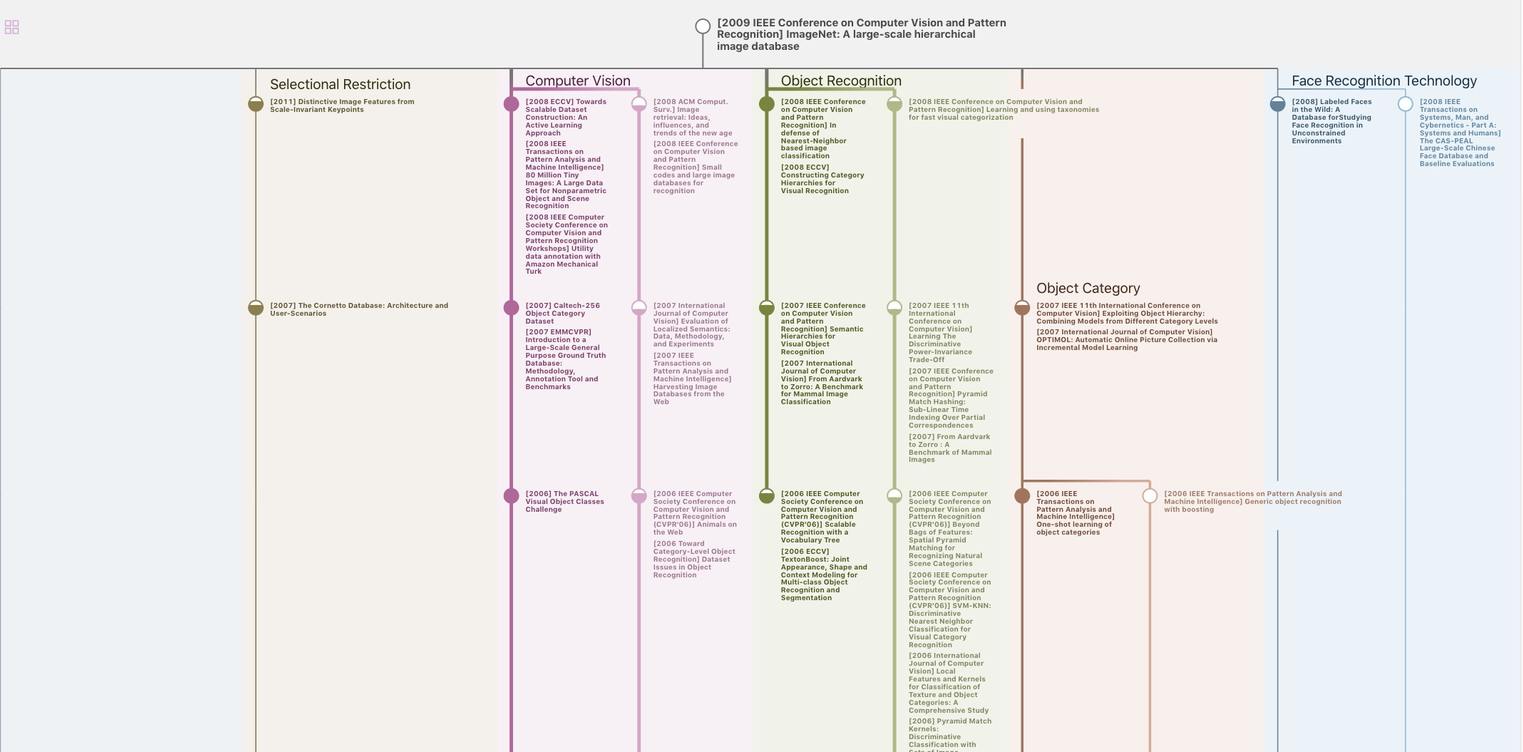
生成溯源树,研究论文发展脉络
Chat Paper
正在生成论文摘要