Task-like training paradigm in CLIP for zero-shot sketch-based image retrieval
Multimedia Tools and Applications(2023)
摘要
The Contrastive Language-Image Pre-training model (CLIP) has recently gained attention in the zero-shot domain. However it still falls short in addressing cross-modal perception, and the semantic gap between seen and unseen classes in Zero-Shot Sketch-Based Image Retrieval (ZS-SBIR). To overcome these obstacles, we propose a Task-Like Training paradigm (TLT). In this work, we view the cross-modal perception and the semantic gap as a multi-task learning process. Before tackling the challenges, we fully utilize CLIP’s text encoder and propose text-based identification learning mechanism to assist the model to learn discriminative features quickly. Next, we propose text prompt tutoring and the cross-modal consistency learning to solve cross-modal perception and the semantic gap, respectively. Meanwhile, we present a collaborative architecture to explore the potential shared information between tasks. Extensive results show that our approach significantly outperforms the state-of-the-art methods on Sketchy, Sketchy-No, Tuberlin, and QuickDraw datasets.
更多查看译文
关键词
Zero-shot sketch-based image retrieval,Text prompt tutoring,Text-based identification learning,Cross-modal consistency learning,Collaborative architecture
AI 理解论文
溯源树
样例
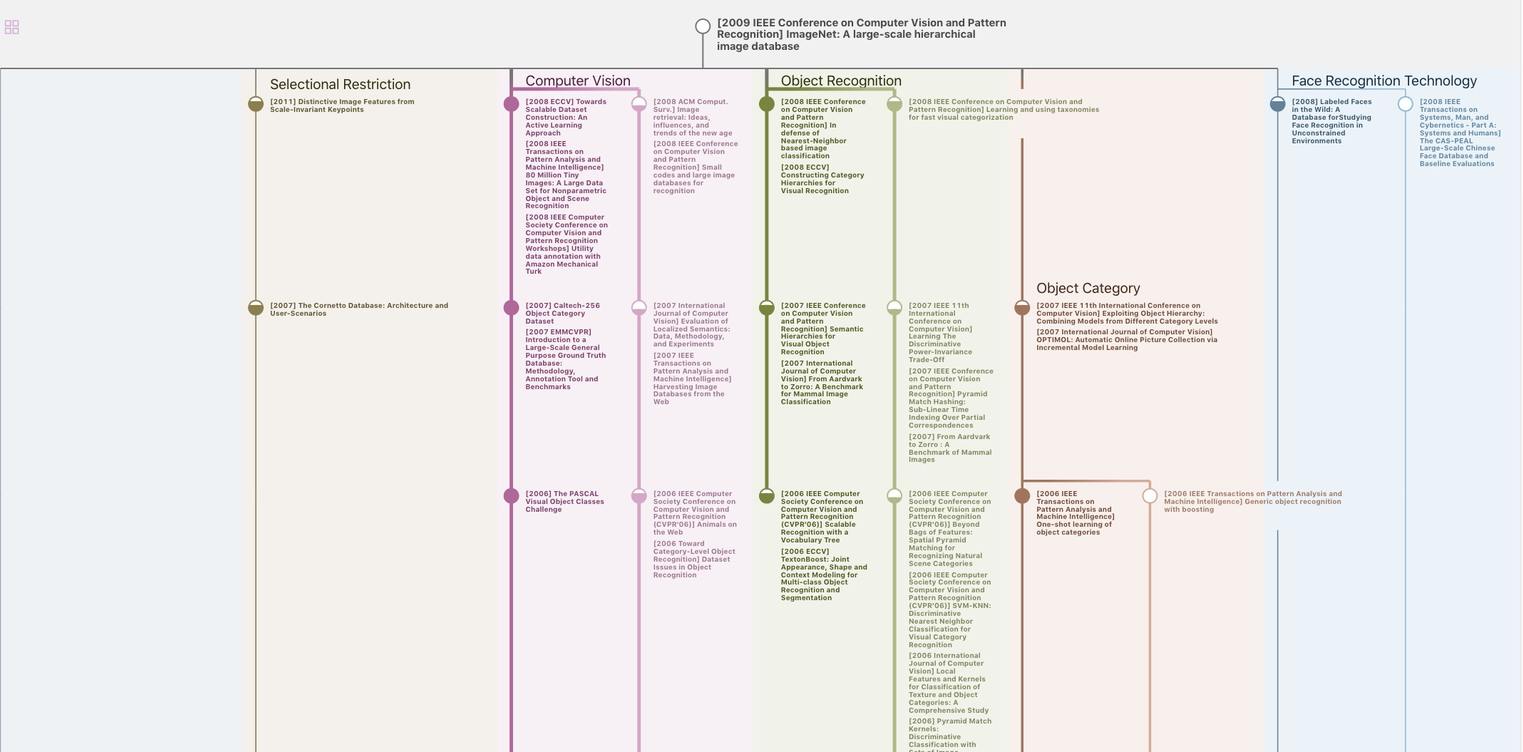
生成溯源树,研究论文发展脉络
Chat Paper
正在生成论文摘要