Stability Analysis of Switched Linear Systems with Neural Lyapunov Functions
AAAI 2024(2024)
摘要
Neural-based, data-driven analysis and control of dynamical systems have been recently investigated and have shown great promise, e.g. for safety verification or stability analysis. Indeed, not only do neural networks allow for an entirely model-free, data-driven approach, but also for handling arbitrary complex functions via their power of representation (as opposed to, e.g. algebraic optimization techniques that are restricted to polynomial functions). Whilst classical Lyapunov techniques allow to provide a formal and robust guarantee of stability of a switched dynamical system, very little is yet known about correctness guarantees for Neural Lyapunov functions, nor about their performance (amount of data needed for a certain accuracy).
We formally introduce Neural Lyapunov functions for the stability analysis of switched linear systems: we benchmark them on this paradigmatic problem, which is notoriously difficult (and in general Turing-undecidable), but which admits existing recently-developed technologies and theoretical results. Inspired by switched systems theory, we provide theoretical guarantees on the representative power of neural networks, leveraging recent results from the ML community. We additionally experimentally display how Neural Lyapunov functions compete with state-of-the-art results and techniques, while admitting a wide range of improvement, both in theory and in practice. This study intends to improve our understanding of the opportunities and current limitations of neural-based data-driven analysis and control of complex dynamical systems.
更多查看译文
关键词
General
AI 理解论文
溯源树
样例
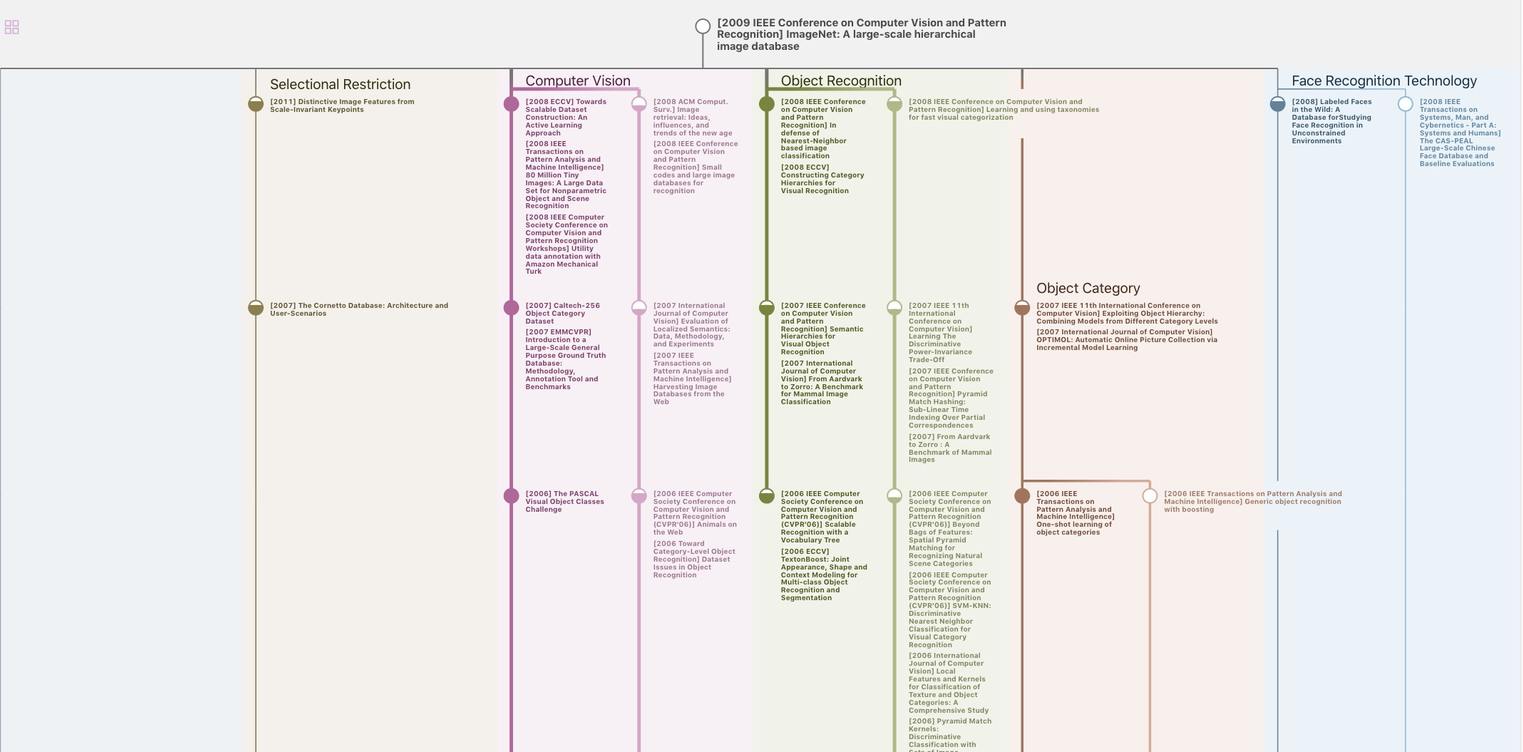
生成溯源树,研究论文发展脉络
Chat Paper
正在生成论文摘要