Domain Transform Model Driven by Deep Learning for Anti-Noise Hyperspectral and Multispectral Image Fusion
IEEE TRANSACTIONS ON GEOSCIENCE AND REMOTE SENSING(2024)
摘要
While fusion of hyperspectral images (HSIs) with low spatial resolution and multispectral images (MSIs) with high spatial resolution has achieved significant success, high-quality fusion between noisy images has always been challenging. In this article, we propose a domain transform model driven by deep learning for anti-noise hyperspectral and multispectral image fusion (DTAFN). This marks the first time that wavelet decomposition theory is combined with deep learning for noise reduction in hyperspectral and MSI fusion. DTAFN initially decomposes hyperspectral and MSIs into frequency components and constructs a novel feature interaction fusion module (FIFM). This module, while using MSIs to guide the removal of noise from HSIs, also achieves the fusion of spatial and spectral information. Furthermore, it maps the fused features to a lower dimensional subspace to enhance computational efficiency. Additionally, we introduce a spatial-spectral self-attention mechanism to optimize the reconstructed frequency components using the subspace features. In the end, the wavelet inverse transform is used to reconstruct the clean fused image. It is worth noting that the extraction of the subspace is considered a process of nonlinear low-rank component extraction, which, to a certain extent, suppresses noise signals. Numerous experiments of mixed noise image fusion are carried out, and the experimental results show that DTAFN can obtain high-quality fusion results, is robust, and superior to the state-of-the-art methods.
更多查看译文
关键词
Anti-noise,domain transform,fusion,hyper-spectral,multispectral
AI 理解论文
溯源树
样例
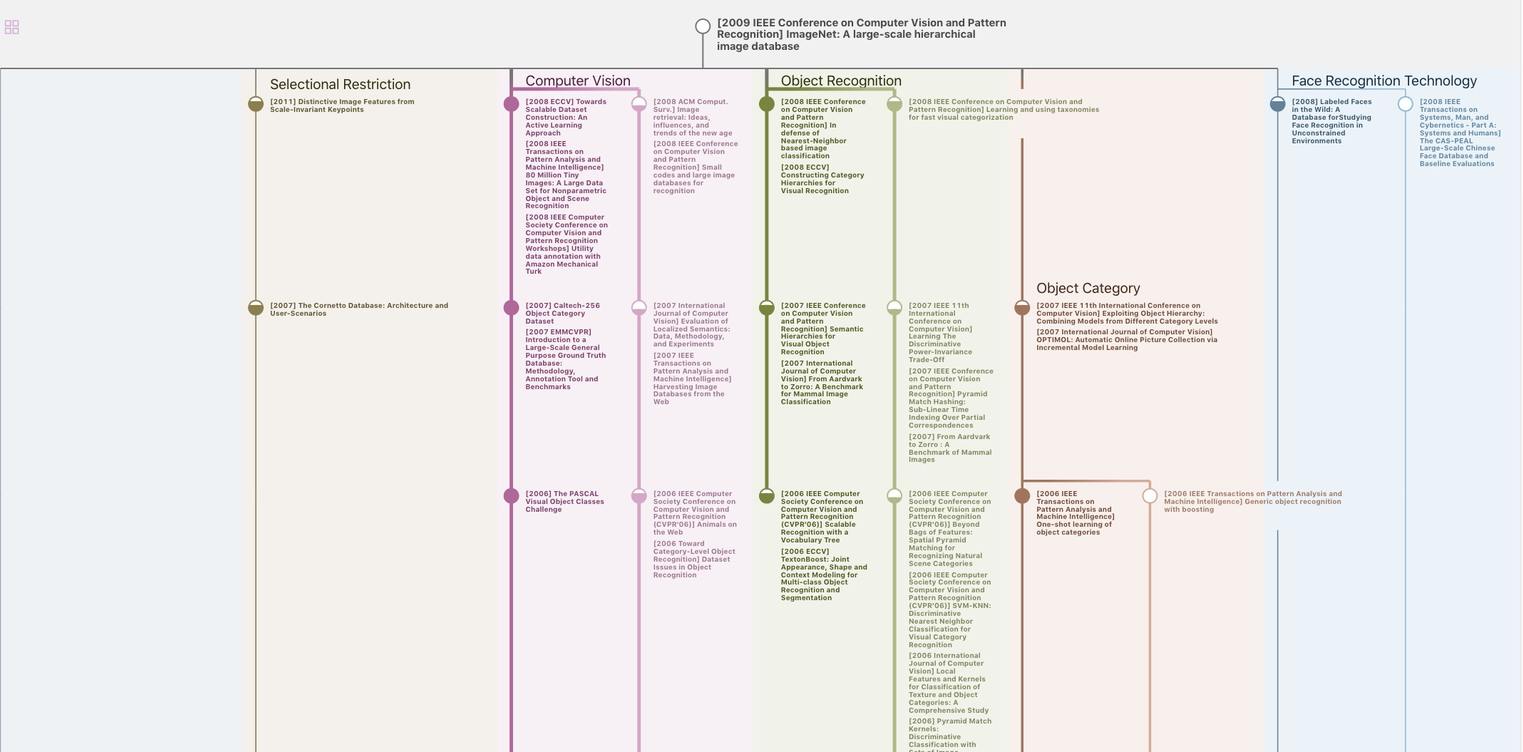
生成溯源树,研究论文发展脉络
Chat Paper
正在生成论文摘要